Featured
How Machine Learning Is Transforming Psychological Science
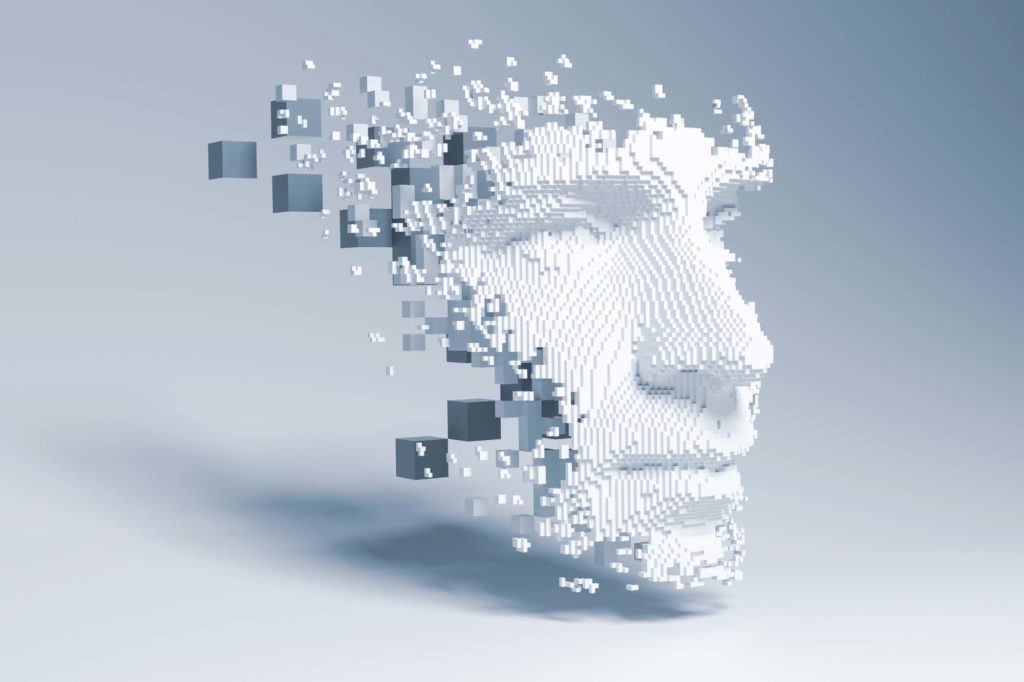
- Artificial intelligence and machine-learning are providing insights that will soon transcend scientists’ observational capabilities, potentially leading to revolutionary advances in understanding human psychology.
- Already, machine-learning techniques have enabled innovative ways to study cognition, personality, behavior, learning, emotions, and more.
- Some researchers caution that algorithms learn from data sources that may contain biases and flawed measurements, affecting their predictive accuracy.
From novel data sources, novel applications • Glossary • Predicting distress • The “black box”
Today, we can train computer programs to give us directions, suggest streaming movies we might enjoy, and even vacuum our living rooms. But machine learning is emerging as far more than a source of convenience; it’s helping scientists better understand our minds.
The growing use of big data and artificial intelligence (AI) is generating trailblazing discoveries and theories about human cognition, behavior, personality, and mental health. This advanced technology stands to transcend the limits of scientists’ observational capabilities.
“What’s going to happen over the next decade, just as a consequence of having more data, is that machine-learning systems are going to be able to pull out more insights than the humans who were thinking about those data may be able to [generate],” Tom Griffiths, a professor of psychology and computer science at Princeton University, said in an interview.
Though some psychological scientists caution that machine learning is too embryonic to yield indubitable conclusions, many see the technology as a revolutionary path toward capturing human psychology in all its complexity.
“AI can provide innovative ideas that may have taken considerable time for humans, in part because it is less constrained by limits on available knowledge and biases,” psychological scientist Laura K. Bartlett and her colleagues at the London School of Economics and Political Science wrote in an article published in Perspectives on Psychological Science (Bartlett, et al., 2022).
In the past 5 years alone, researchers have demonstrated the use of machine learning to examine consciousness, decision-making, perception, and behavior.
From novel data sources, novel applications
Machine-learning research is evolving rapidly thanks to mammoth increases in computing power and 21st-century data sources, including social media, smartphone texts, and crowd-sourced research tools such as Amazon Mechanical Turk (MTurk).
“Machine learning’s utility is born out of necessity with these novel data types,” Ross Jacobucci, a University of Notre Dame quantitative psychologist, said in an interview. “To analyze most of the data collected from novel sources, you can’t use traditional statistical models.”
The emergence of massive data sets and advanced technology has spawned university labs focusing specifically on the use of machine learning. Carnegie Mellon University (CMU), for instance, launched BrainHub, an interdisciplinary initiative aimed at developing new technologies to measure and analyze the brain. The University of Colorado Boulder’s Institute of Cognitive Science houses experts in psychology, computer science, neuroscience, linguistics, and other disciplines and aims to modernize the study of human cognition. Stanford University’s Computational Psychology and Well-Being Lab uses social-media data and machine learning to examine health and psychological issues.
Griffiths, a Guggenheim Fellow, directs Princeton’s Computational Cognitive Science Lab, which builds mathematical models to understand the roots of human cognition. He and collaborators at the University of Chicago and the Stevens Institute of Technology recently taught an AI algorithm to model people’s first impressions of others.
Glossary
Artificial intelligence (AI)—the ability of a computer system to mimic human learning, problem-solving and other cognitive functions using math and logic.
Machine learning—a subset of AI that uses mathematical models to help computers learn independently based on prior experience.
Neural network—a computer system patterned after the activity of neurons in the human brain.
Deep learning—a machine learning application that interprets big data and recognizes patterns.
The research team asked thousands of people, recruited on MTurk, to give their first impressions of computer-generated photos of faces. Over nearly 11,000 sessions, the participants ranked each pictured individual on qualities such as intelligence, attractiveness, trustworthiness, religiosity, and political orientation. The researchers used the mass of responses to train an artificial neural network—a form of AI that processes information much like the human brain—to make similar snap judgments of photographed faces.
They learned the algorithm’s judgments mirrored many of the participants’ impressions. Smiling faces were seen as more trustworthy, for example. People wearing glasses were judged to be more intelligent (Peterson et al., 2022).
The results suggest that AI can help predict how others, including potential employers or romantic partners, will perceive us on the basis of our facial features and expressions.
“The algorithm doesn’t provide targeted feedback or explain why a given image evokes a particular judgment,” Jordan W. Suchow, a cognitive psychologist at the Stevens Institute, said in a press release. “But even so it can help us to understand how we’re seen—we could rank a series of photos according to which one makes you look most trustworthy, for instance, allowing you to make choices about how you present yourself.”
Griffiths and his collaborators have also created algorithms to generate new theories on risky decision-making and planning (Peterson, et al., 2021; Callaway, et al., 2022). Others have employed machine learning in a variety of behavioral, personality, cognitive, and clinical studies.
Management researchers such as computational psychologist Sandra C. Matz at Columbia University have applied a machine-learning technique to study the link between spending and personality traits. In a study reported in Psychological Science, Matz and colleagues collected data from nearly 2,200 consenting users of a money-management app, resulting in two million spending records from credit cards and bank transactions. The account holders also completed a personality survey that measured materialism, self-control, and the “Big Five” personality traits of openness to experience, conscientiousness, extraversion, agreeableness, and neuroticism.
The researchers organized the spending data into broad categories—including supermarkets, furniture stores, insurance policies, online stores, and coffee shops. They then used random forest modeling, a machine-learning technique that combines multiple algorithms, to analyze whether participants’ relative spending across categories signaled specific personality types.
The scientists marked several ties between spending habits and certain traits, especially the narrow qualities of materialism and self-control. Those scoring high on materialism, for example, spent more on jewelry and less on charitable donations (Gladstone et al., 2019).
Machine-learning techniques have also enabled innovative ways to study emotions across cultures. Daniel Oberfeld-Twistel, a psychological scientist at Johannes Gutenberg University Mainz, created an algorithm that he and an international research team used to explore how people from different parts of the world associate colors with emotions (e.g., red with anger). They combined questionnaire responses from 4,598 individuals in 30 countries with Oberfeld-Twistel’s creation to show the large number of color/emotion associations that are similar across the globe and those that vary from country to country (Jonauskaite et al., 2020).
Machine learning is also yielding discoveries that could provide insights into human learning and improve education. CMU researchers Robert Mason and Marcel Just, for example, used machine learning to identify potential improvements in scientific instruction. They recruited 9 advanced physics and engineering students and had them undergo brain scans while they studied 30 concepts including gravity, entropy, and velocity. Using a neural decoding technique developed at CMU, the researchers found that each concept triggered its own brain activation pattern. The results, the authors said, reveal how the brain learns and discovers abstract scientific concepts (Mason & Just, 2016).
Cognitive psychologist Sidney K. D’Mello and his colleagues at the University of Colorado Boulder have used a machine-learning algorithm to examine eye-tracking data involving students; they identified eye patterns associated with reading comprehension and mind wandering (D’Mello et al., 2020; Hutt et al., 2017). Educational psychologists Michael Sailer and Frank Fischer of Ludwig Maximilian University of Munich have employed artificial neural networks to provide feedback that helped teachers better identify students with dyslexia and other learning difficulties (Sailer et al., 2022).
Related content we think you’ll enjoy
-
Children, Creativity, and the Real Key to Intelligence
APS President Alison Gopnik writes that the contrast between the reasoning of creative 4-year-olds and predictable artificial intelligence may be a key to understanding how human intelligence works.
-
The Emerging Science of Suicide Prevention
Advances in assessment and intervention could help tip the scale toward survival, one life at a time.
-
Children’s Preference for Learning Could Help Create Curious AI
The strategies children use to search for rewards in their environment could be used to create more sophisticated forms of artificial intelligence.
Predicting distress
Psychological scientists have increasingly turned to artificial intelligence to spot and predict mental health problems within large populations. APS James McKeen Cattell Fellow Ian Deary and his colleagues at the University of Edinburgh have demonstrated the use of machine learning to parse specific psychological and demographic traits that influence mental health. Deary, working with psychological scientists Drew Altschul and Matthew Iveson, trained an algorithm to examine generational differences in loneliness. Tapping longitudinal data sets, they measured psychological and sociodemographic traits of more than 4,000 individuals in two age groups: 45–69 and over 70. By training an algorithm to identify the most significant predictors of loneliness, they identified several risk factors. Those influences included low emotional stability and solitary living—especially among the oldest men (Altschul et al., 2021).
Johannes C. Eichstaedt, director of the Computational Psychology and Well-Being Lab at Stanford, mixes machine learning with U.S. Census, polling, and social-media data to study a variety of health and behavior issues. He and his colleagues are showing how algorithms can help predict depression, loneliness, and even heart disease (Eischsteadt et al., 2016, 2018).
Relatedly, Yale University psychological scientist and APS Spence Awardee Arielle Baskin-Sommers and colleagues trained a machine-learning model to sift through longitudinal data from 9- and 10-year-old children to predict the development of conduct disorder (Chan, et al, 2022). Paola Pedrelli, an assistant professor of psychology at Harvard Medical School, has been working with Massachusetts Institute of Technology professor Rosalind Picard to develop algorithms that can help diagnose and monitor symptoms among patients being treated for major depression (Gold & Gross, 2022). At the University of Vermont, clinical psychologist Ellen McGinnis led a study that used an algorithm to detect signs of depression and anxiety in young children’s speech patterns (McGinnis et al., 2019).
But findings from clinical research using AI have generated some qualms. Researchers have cautioned that machine-learning models analyze psychological variables that may have been poorly measured in the first place. Data sets may include non-representative samples or measurement errors that algorithms absorb and use to produce their predictions.
“The fact that we use more powerful machine-learning methods does not negate the term garbage in–garbage out,” Jacobucci and Kevin J. Grimm of Arizona State University wrote in an article for Perspectives on Psychological Science (Jacobucci & Grimm, 2020). Jacobucci has raised particular concerns about studies that use AI to predict suicide risk. A variety of studies have demonstrated machine-learning techniques that flag indicators of suicidal thinking and behavior in large data sets (Walsh et al., 2017, Ribeiro et al., 2019). But Jacobucci’s own research suggests that machine-learning approaches are no better at predicting suicidal behaviors than traditional measures (Jacobucci et al., 2021).
“At a higher level I would say the promise of machine learning with traditional data types in psychology has been somewhat unmet,” he said in an interview. “I think a number of papers on suicide have found slight benefits of machine learning over linear models. But from an actionable perspective, I don’t really know what it’s adding.”
Machine-learning research may also be hampered by so-called algorithmic bias. Models learn from data sets that may contain homogenous samples or the implicit assumptions of the scientists who collected the data in the first place. As APS Fellow Robert Goldstone, a cognitive psychologist at Indiana University, wrote in Current Directions in Psychological Science, AI is “not immune to the biases of the society that created it” (Goldstone, 2022).
For example, a machine-learning model may be trained only on data involving White individuals, and the predictions the model produce may not generalize to other racial groups, psychological scientist Louis Tay (Purdue University) and colleagues wrote in an article for Advances in Methods and Practices in Psychological Science.
Tay and his co-authors shared some techniques that psychologists can use to mitigate machine-learning bias, such as making sure a trained machine-learning model functions similarly across different subgroups of interest (Tay et al., 2022).
The “black box”
Among psychologists’ other concerns about machine-learning techniques are the so-called “black box” results they produce; the algorithms can predict an outcome but do not provide the causal or explanatory information that traditional methods generate. Researchers such as Griffiths are developing interpretable machine-learning models (Agrawal et al., 2020). But quantitative psychological researchers Tal Yarkoni and Jacob Westfall of the University of Texas at Austin say that research programs may prove more fruitful by focusing on the predictive power of machine learning and treating explanation as a secondary goal. They note that models held up as explanations of behavior in an initial sample faltered in replications with subsequent samples.
“We argue that psychology’s near-total focus on explaining the causes of behavior has led much of the field to be populated by research programs that provide intricate theories of psychological mechanism, but that have little (or unknown) ability to predict future behaviors with any appreciable accuracy,” Yarkoni and Westfall wrote in an article for Perspectives on Psychological Science. “We propose that principles and techniques from the field of machine learning can help psychology become a more predictive science” (Yarkoni & Westfall, 2017).
Beyond prediction, machine learning and big data will enable social scientists to chart new territory in exploring psychological phenomena.
“The truth is, human behavior is very complex,” Griffiths said, “and the more data we get, the more we can actually identify systematic variables that are influencing that complexity.”
Feedback on this article? Email [email protected] or login to comment. Interested in writing for us? Read our contributor guidelines.
Agrawal, M., Peterson, J.C., & Griffiths, T.L. (2020). Scaling up psychology via scientific regret minimization. Proceedings of the National Academy of Sciences, U.S.A., 117(16), 8825–8835. https://doi.org/10.1073/pnas.1915841117
Altschul, D., Iveson, M., & Deary, I.J. (2021). Generational differences in loneliness and its
psychological and sociodemographic predictors: An exploratory and confirmatory
machine learning study. Psychological Medicine, 51, 991–1000. https://doi.org/10.1017/S0033291719003933
Bartlett, L. K., Pirrone, A., Javed, N., & Gobet, F. (2022). Computational scientific discovery in psychology. Perspectives on Psychological Science. https://doi.org/10.1177/17456916221091833
Callaway, F., van Opheusden, B., Gul, S., Das, P., Krueger, P.M., Griffiths, T.L., & Lieder F. (2022). Rational use of cognitive resources in human planning. Nature Human Behavior, 6, 1112–1125. https://doi.org/10.1038/s41562-022-01332-8
Chan, L., Simmons, C., Tillem, S., Conley, M., Brazil, I.A., & Baskin-Sommers, A. (2022). Classifying conduct disorder using a biopsychosocial model and machine learning methods. Biological Psychiatry: Cognitive Neuroscience and Neuroimaging. https://doi.org/10.1016/j.bpsc.2022.02.004
D’Mello, S.K., Southwell, R., & Gregg, J. (2020). Machine-learned computational models can enhance the study of text and discourse: A case study using eye tracking to model reading comprehension. Discourse Processes, 57(5-6), 420–440. https://doi.org/10.1080/0163853X.2020.1739600
Eichstaedt, J. C., Schwartz, H. A., Kern, M. L., Park, G., Labarthe, D. R., Merchant, R. M., Jha, S., Agrawal, M., Dziurzynski, L. A., Sap, M., Weeg, C., Larson, E. E., Ungar, L. H., & Seligman, M. E. P. (2015). Psychological language on Twitter predicts county-level heart disease mortality. Psychological Science, 26(2), 159–169. https://doi.org/10.1177/0956797614557867
Eichstaedt, J. C., Smith, R. J., Merchant, R. M., & Schwartz, H. A. (2018). Facebook language predicts depression in medical records. Proceedings of the National Academy of Sciences, U.S.A., 115(44), 11203–11208. https://doi.org/10.1073/pnas.1802331115
Gladstone, J. J., Matz, S. C., & Lemaire, A. (2019). Can psychological traits be inferred from spending? Evidence from transactional data. Psychological Science, 30(7), 1087–1096. https://doi.org/10.1177/0956797619849435
Gold, A. & Gross, D. (2022). Deploying machine learning to improve mental health. MIT News. https://news.mit.edu/2022/deploying-machine-learning-improve-mental-health-rosalind-picard-0126
Goldstone, R. L. (2022). Performance, well-being, motivation, and identity in an age of abundant data: Introduction to the “Well-Measured Life” special issue of Current Directions in Psychological Science. Current Directions in Psychological Science, 31(1), 3–11. https://doi.org/10.1177/09637214211053834
Hutt, S., Mills, C., Bosch, N., Krasich, K., Brockmole, J., & D’Mello, S. (2017). “Out of the fr-eye-ing plan”: Towards gaze-based models of attention during learning with technology in the classroom. Proceedings of the 25th Conference on User Modeling, Adaptation and Personalization, 94–103. https://doi.org/10.1145/3079628.3079669
Jacobucci, R., & Grimm, K. J. (2020). Machine learning and psychological research: The unexplored effect of measurement. Perspectives on Psychological Science, 15(3), 809–816. https://doi.org/10.1177/1745691620902467
Jacobucci, R., Littlefield, A. K., Millner, A. J., Kleiman, E. M., & Steinley, D. (2021). Evidence of inflated prediction performance: A commentary on machine learning and suicide research. Clinical Psychological Science, 9(1), 129–134. https://doi.org/10.1177/2167702620954216
Jonauskaite, D., Abu-Akel, A., Dael, N., Oberfeld, D., Abdel-Khalek, A. M., Al-Rasheed, A. S., Antonietti, J.-P., Bogushevskaya, V., Chamseddine, A., Chkonia, E., Corona, V., Fonseca-Pedrero, E., Griber, Y. A., Grimshaw, G., Hasan, A. A., Havelka, J., Hirnstein, M., Karlsson, B. S. A., Laurent, E., … Mohr, C. (2020). Universal patterns in color–emotion associations are further shaped by linguistic and geographic proximity. Psychological Science, 31(10), 1245–1260. https://doi.org/10.1177/0956797620948810
Mason, R. A., & Just, M. A. (2016). Neural representations of physics concepts. Psychological Science, 27(6), 904–913. https://doi.org/10.1177/0956797616641941
McGinnis, E. W., Anderau, S. P., Hruschak, J., Gurchiek, R. D., Lopez-Duran, N. L., Fitzgerald, K., Rosenblum, K. L., Muzik, M., & McGinnis, R. (2019). Giving voice to vulnerable children: Machine learning analysis of speech detects anxiety and depressions in early childhood. IEEE Journal of Biomedical and Health Informatics, 23(6), 2294–2301. https://doi.org/10.1109/JBHI.2019.2913590
Peterson, J. C., Bourgin, D. D., Agrawal, M., Reichman, D., & Griffiths, T. L. (2021). Using large-scale experiments and machine learning to discover theories of human decision-making. Science, 372, 1209–1214. https://doi.org/10.1126/science.abe2629
Peterson, J. C., Uddenberg, S., Griffiths, T. L., Todorov, A., & Suchow, J. W. (2022). Deep models of superficial face judgments. Proceedings of the National Academy of Sciences, U.S.A., 119(17), Article e2115228119. https://doi.org/10.1073/pnas.2115228119
Ribeiro J. D., Huang X., Fox K. R., Walsh C. G., & Linthicum K. P. (2019). Predicting imminent suicidal thoughts and nonfatal attempts: The role of complexity. Clinical Psychological Science, 7, 941–957. https://doi.org/10.1177/216770261983846
Sailer, M., Bauer, E., Hofmann, R., Kiesewetter, J., Glas, J., Gurevych, I., & Fischer, F. (2022). Adaptive feedback from artificial neural networks facilitates pre-service teachers’ diagnostic reasoning in simulation-based learning. Learning and Instruction, 83. https://doi.org/10.1016/j.learninstruc.2022.101620
Tay, L., Woo S.E, Hickman, L., Booth B.M., & D’Mello S. (2022). A conceptual framework for Investigating and mitigating machine-learning measurement bias (MLMB) in psychological assessment. Advances in Methods and Practices in Psychological Science, 5(1). https://doi.org/10.1177/25152459211061337
Walsh, C. G., Ribeiro, J. D., & Franklin, J. C. (2017). Predicting risk of suicide attempts over time through machine learning. Clinical Psychological Science, 5(3), 457–469. https://doi.org/10.1177/2167702617691560
Yarkoni, T., & Westfall, J. (2017). Choosing prediction over explanation in psychology: Lessons from machine learning. Perspectives on Psychological Science, 12(6), 1100–1122. https://doi.org/10.1177/1745691617693393
APS regularly opens certain online articles for discussion on our website. Effective February 2021, you must be a logged-in APS member to post comments. By posting a comment, you agree to our Community Guidelines and the display of your profile information, including your name and affiliation. Any opinions, findings, conclusions, or recommendations present in article comments are those of the writers and do not necessarily reflect the views of APS or the article’s author. For more information, please see our Community Guidelines.
Please login with your APS account to comment.