Presidential Column
Children, Creativity, and the Real Key to Intelligence
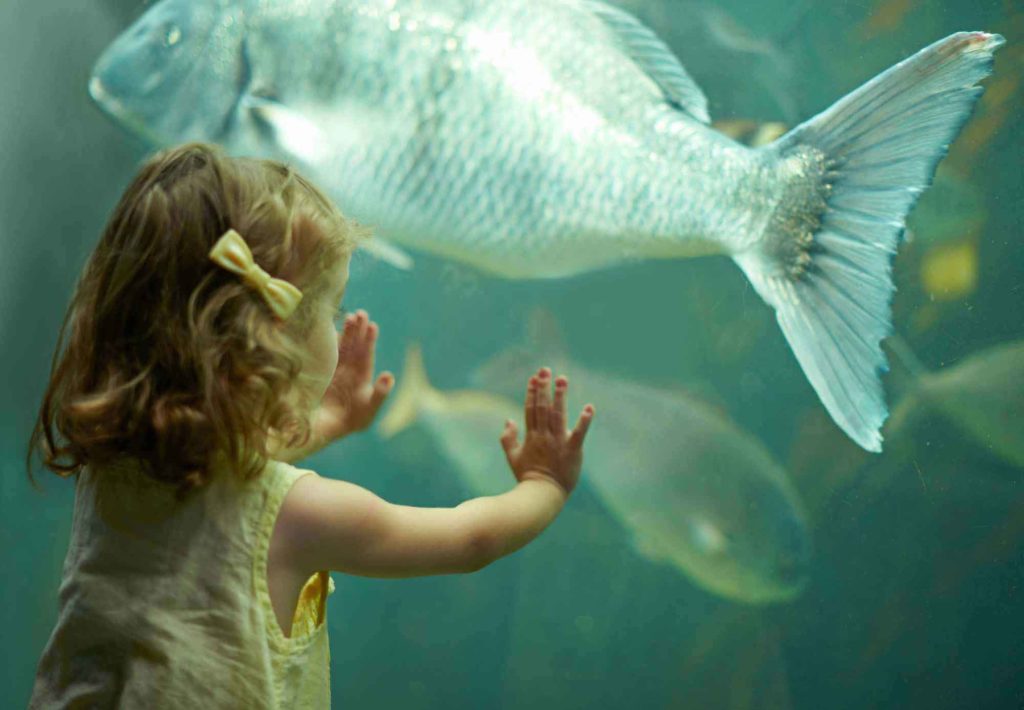
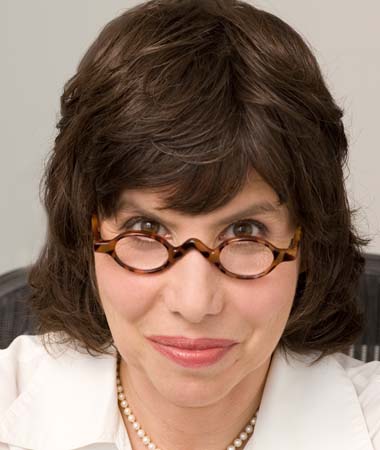
Recently, one of the researchers at the Berkeley Artificial Intelligence Research Lab was taking her 4-year-old son for a walk through the campus. The little boy looked up at the famous campanile clock tower and exclaimed with surprise and puzzlement, “There’s a clock way up there!” Then, after a few minutes, he thoughtfully explained, “I guess they put the clock up there so that the children couldn’t reach it and break it.” Everyone with a 4-year-old has similar stories of preschool creativity—charming, unexpected takes on the world and its mysteries that nevertheless have their own logic and sense.
Suppose you ask a Large Language Artificial Intelligence (AI) model the same question: Why is there a clock on top of the campanile? You will get a much more predictable and less interesting answer. GPT-3, one of the most impressive models, based on billions of words of text, replies sensibly enough, “There is a clock on top of the campanile because it is a campanile clock,” or “The clock on top of the campanile is there to tell time.” In fact, predictability is the whole point of these models. The models are trained by giving them a few words of text and asking them to predict the next few words for billions of cases. This contrast between the creative 4-year-old and the predictable AI may be one of the keys to understanding how human intelligence works and how it might interact with artificial intelligence. Psychology, and especially child psychology, will play a crucial role in creating and using the technology of the future.

At first, it may seem incongruous to compare a 4-year-old child and the latest state-of-the-art AI. Surely, the preschool world of finger paints, teddy bears, and imaginary tea parties is at the farthest remove from the Silicon Valley universe of venture capitalists, start-ups, and machine-learning algorithms (though both groups do have an irrational fondness for unicorns). And surely these powerful, expensive machines would outshine small children at every kind of task, even if they couldn’t yet entirely compete with grown-ups.
But way back in 1950, Alan Turing, who invented the modern computer, pointed out why children might be the best model for truly intelligent AI. In his paper “Computing Machinery and Intelligence,” he introduced the famous “Turing test.” If a machine could convincingly simulate an adult conversation, we would have to accept that it was intelligent. But though everyone knows about that imitation test, few people notice that in the very next section, Turing proposed a different and more challenging one: “Instead of trying to produce a programme to simulate the adult mind, why not rather try to produce one which simulates the child’s?” Turing’s point was that whereas adults may possess and use particular kinds of knowledge, children actually construct that knowledge based on their experience. That kind of learning may be the real key to intelligence.
In fact, in the last few years, AI researchers have turned to developmental psychologists to understand the kinds of intelligence that let us learn so much. This idea has a long history in cognitive science. The neural network models of the ’80s and the Bayesian generative models of the aughts inspired collaborations between developmental psychologists and computer scientists. But the recent “AI spring,” which depends so much on machine learning, has revived the collaboration in a newly exciting way. My lab at Berkeley is part of a Machine Common Sense program that tries to see if we can give AI systems some of the common-sense learning capacities that we take for granted in young children.
What are your thoughts on AI? Artificial intelligence is the theme of the next issue of the Observer (January/February 2023), and APS wants to hear from members. What do you believe are the biggest opportunities, as well as the biggest ethical challenges, that psychological science must address involving AI? Comment by scrolling down (you must be logged in), or weigh in by completing this form.
These projects have made us realize just how profound the differences are between current AI and children. The most impressive new systems, like GPT-3 or Dall-E, work by extracting statistical patterns from enormous data sets, taking advantage of the hundreds of millions of words and images on the internet. These systems are also “supervised”—they get positive or negative feedback about each decision they make. And although they can make impressive predictions, they are not so good at generalizing to new examples, especially if those examples are very different from the examples they were trained on.
By contrast, young children are exposed to very different kinds of data. They learn from real interactive experiences with just a few people, animals, and objects. The information they get isn’t tightly controlled, but spontaneous and haphazard. And yet, as the campanile example illustrates, they are very good at generalizing to new situations.
How do children do it? We think there are two essential techniques that allow children to go beyond the kind of statistics extraction that is standard in machine learning. First, they build abstract models of the world around them—intuitive theories of physics, biology, and math, and of the psychological and social world, too. The idea that children are little scientists going beyond the data to build theories is not new of course; the Cognitive Development Society just celebrated the 30-something birthday of the “theory theory.” But one of the big benefits of a theory, whether for a scientist, a child, or a computer, is that it lets us go beyond the data we have seen before to make radically new predictions and generalizations. It’s exciting to think that we might be able to describe those models mathematically and design artificial systems that can build them, too.
The second piece of the puzzle is exploration. Children are active, experimental learners—they don’t just passively absorb information. Instead, they do things to the world to find out more about it. It may look to us as if the kids are just “getting into everything.” But a raft of new developmental studies show that even babies and toddlers are surprisingly sophisticated and intelligent explorers, searching out just the information they need to learn most effectively. And several recent studies, including ones from our lab, show that children really are more exploratory than grown-ups. In AI, researchers are trying to design curious, “self-supervised,” intrinsically motivated agents that, like children, actively seek out knowledge.
There is another kind of exploration that is particularly relevant for thinking about childhood creativity, a kind of internal exploration rather than the external “getting into everything” kind. Where do ideas like the campanile conundrum solution come from? Somehow children are always coming up with ideas that are novel and unexpected and yet plausible. This is much harder for current AI systems. They can do very well if a problem is precisely defined and relatively circumscribed, even if it’s really hard, like finding the right move in chess. But the kind of open-ended creativity that we see in children involves a challenging combination of randomness and rationality. When you interact for a while with a system like GPT-3, you notice that it tends to veer from the banal to the completely nonsensical. Somehow children find the creative sweet spot between the obvious and the crazy.
Trying to design computers that learn like children is a fascinating project for developmental psychologists because it makes us realize the power and mystery of children’s minds. And we will need to incorporate some childlike model-building and exploration into AI systems to solve even quite simple problems. The classic “Moravec’s paradox” in AI points out that often things that are hard for people are easy for computers and vice versa. It’s easier to make a machine that can calculate the right chess moves than one that can pick up scattered chess pieces and put them in the right squares on the board. Designing robots that can actually interact with the real world in a robust and flexible way is much harder than designing systems that can win Atari games. Child-like model building and exploration may be important for useful applications, like a robot that could fold laundry or sort screws and nails.
Of course, any attempt to create what the researchers call AGI—artificial general intelligence that could be on a par with humans—will have to solve those problems, too. But should AGI be our ambition? Maybe not. Perhaps a better way to think about artificial intelligence is that it can create technologies that are complementary to human intelligence rather than in competition with them. And here again it’s worth considering the contrast between the language models and the 4-year-olds.
I’ve argued that many AI systems are best understood as what we might call “cultural technologies.” They are like writing, print, libraries, internet search engines, or even language itself. They summarize and “crowd-source” knowledge rather than creating it. They are techniques for passing on information from one group of people to another, rather than techniques for creating a new kind of person. Asking whether GPT-3 or Dall-E is intelligent or knows about the world is like asking whether the University of California’s library is intelligent or whether a Google search “knows” the answer to your questions.

Cultural technologies aren’t like intelligent humans, but they are essential for human intelligence. Psychologists like Joseph Henrich have argued that these cultural abilities to transmit information from one generation to the next are at the heart of our evolutionary success. New technologies that make cultural transmission easier and more effective have been among the greatest engines of human progress. But like all powerful technologies, they can also be dangerous. Socrates thought that writing was a really bad idea. You couldn’t have the Socratic dialogues in writing that you could in speech, he said, and people might believe things were true just because they were written down—and he was right. Technological innovations let Benjamin Franklin print inexpensive pamphlets that spread the word about democracy and supported the best aspects of the American Revolution. But the same technology also released a flood of libel and obscenity, as bad as anything on Twitter and Facebook, and contributed to the worst aspects of the French Revolution. People can be biased, gullible, racist, sexist, and irrational. So summaries of what people who preceded us have thought, whether from an “old wives’ tale,” a library, or the internet, inherit all of those flaws. And that can clearly be true for AI models, too.
Psychologists who study cultural transmission and evolution point out that effective cultural progress depends on a delicate balance between imitation and innovation. Imitating and learning from the past generation is crucial, and developmental psychologists have shown that even infants are adept at imitating others. AI models like GPT-3 are essentially imitating millions of human writers. But that learning would never lead to progress without the complementary ability for creative innovation. The ability of each generation to revise, alter, abandon, expand, and (hopefully) improve on the knowledge and skill of the previous generation has always been crucial for progress. That kind of creative innovation is the specialty of children and adolescents.
This process of creative innovation applies to designing the AI systems themselves. In the past when we invented new cultural technologies, we also had to invent new norms, rules, laws, and institutions to make sure that the benefits of those systems outweighed the costs. Writing, print, and libraries only worked because we also invented editors and librarians, libel laws and privacy regulations. Human innovation will always be the essential complement to the cultural technologies we create.
Feedback on this article? Email [email protected] or login to comment. Interested in writing for us? Read our contributor guidelines.
Related content we think you’ll enjoy
-
Children’s Preference for Learning Could Help Create Curious AI
The strategies children use to search for rewards in their environment could be used to create more sophisticated forms of artificial intelligence.
-
What Do Babies Have That Computers Don’t?
Machines are getting smarter, but they’re no match for human infants — APS William James Fellow Linda B. Smith explains why.
-
A Cloudy Future: Why We Don’t Trust Algorithms When They’re Almost Always Right
Researchers explore our preference for human skill and instinct over technologies that have proven themselves better than us at driving, performing surgery, and making hiring decisions.
APS regularly opens certain online articles for discussion on our website. Effective February 2021, you must be a logged-in APS member to post comments. By posting a comment, you agree to our Community Guidelines and the display of your profile information, including your name and affiliation. Any opinions, findings, conclusions, or recommendations present in article comments are those of the writers and do not necessarily reflect the views of APS or the article’s author. For more information, please see our Community Guidelines.
Please login with your APS account to comment.