Featured
Research at the Interface of Artificial Intelligence and Psychological Science, 2018–2022
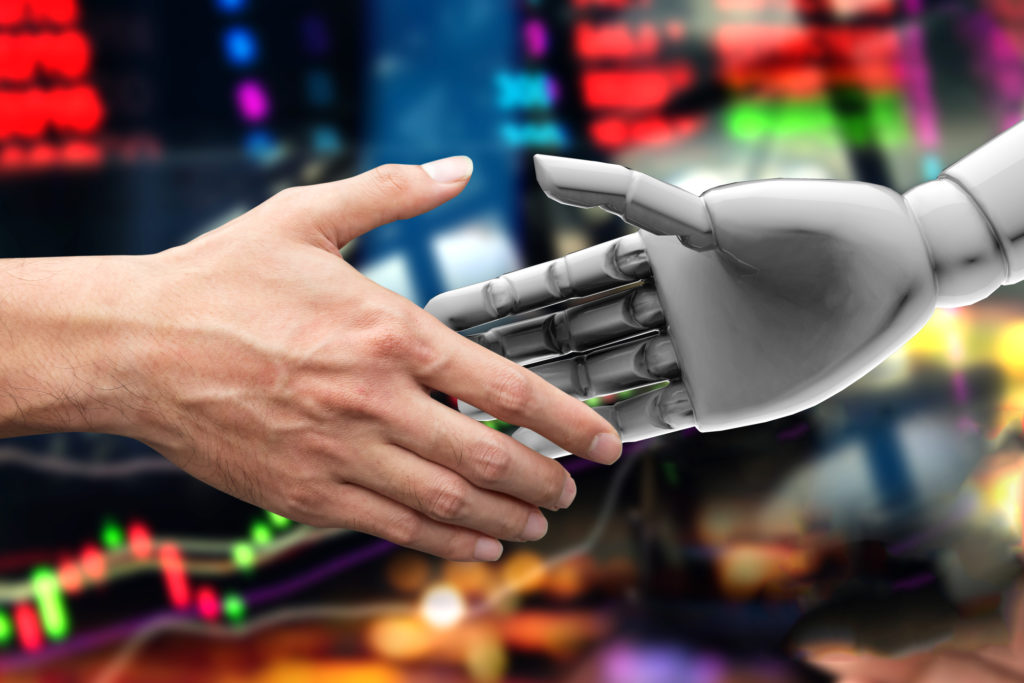
In 1956, a group of scientists coined the term “artificial intelligence” (AI) to refer to the implementation in man-made hardware, such as computers, of the intelligence features that are found in humans and animals. Today, the Oxford English Dictionary defines AI as the theory and development of computer systems able to perform tasks that usually require human intelligence, such as language or decision-making.
Click here to see a list of relevant AI terms and their definitions.
AI has been described as having the potential to improve human lives—for instance, automating certain work functions, guiding driverless cars, increasing the accuracy of clinical diagnoses, improving personalized treatment options, and helping students learn more efficiently. Efforts to implement intelligence into machines have also been thought to lead to breakthrough understanding and new theories of how biological beings learn.
But developments in AI have also met criticism. Ethical issues must be considered when machines and researchers have access to large amounts of data, in many cases attached to personal information. Relying on the decisions made by machines can have catastrophic effects, as can relying on decisions made by humans, but responsibility is easier to assign with humans. And AI must be understood in a social context, taking into account the environments in which people live and how they perceive those environments, as well as their perceptions about AI. Moreover, some researchers have argued that AI’s heavy use of statistics to identify regularities in large amounts of data might not result in a better scientific understanding of intelligence and cognition.
Many articles in the APS journals have addressed AI’s potential benefits and risks. This collection of research explores various aspects of AI, published between 2018 and 2022 in the APS journals Psychological Science, Clinical Psychological Science, Current Directions in Psychological Science, Perspectives on Psychological Science, Psychological Science in the Public Interest, and Advances in Methods and Practices in Psychological Science.
Contributing to psychological science: Powerful measurement tools and models
Computational Scientific Discovery in Psychology
Laura K. Bartlett, Angelo Pirrone, Noman Javed, and Fernand Gobet
Perspectives on Psychological Science
Bartlett and colleagues addressed the current and future directions of computational scientific discovery, including AI, and its applications in psychological science. Along with AI becoming increasingly prevalent in our daily lives, its application to different scientific domains is becoming more widespread. AI can assist in new discoveries both as a tool that gives scientists more freedom to generate new theories and by making creative discoveries autonomously. Conversely, psychological concepts such as heuristics have refined and improved artificial systems.
Robots as Mirrors of the Human Mind
Agnieszka Wykowska
Current Directions in Psychological Science
Robots can increase our knowledge about human cognition and serve as tools for research in psychological science. Wykowska gave examples in which robots have been used to study mechanisms of social cognition that require reciprocal interaction between two people (e.g., joint attention, when one person directs their attention to a location and their partner attends there in response). The author also discussed whether and when robots are perceived as possessing human characteristics and how robots have been used to implement computational models of human cognition.
Comparing the Visual Representations and Performance of Humans and Deep Neural Networks
Robert A. Jacobs and Christopher J. Bates
Current Directions in Psychological Science
Deep neural networks (DNNs)—artificial intelligence systems that can learn from data input—might provide insights about human intelligence, namely human visual perception. The visual processing strategies of DNNs and people are similar but also present some differences: (a) Small perturbations in images are enough for an object not to be recognized by DNNs but do not affect human visual perception; (b) people are better than DNNs at classifying images of letters after only a few training examples; and (c) people are better than DNNs at comparing images and evaluating whether they are similar. Overall, people are better at using image context or previous knowledge than DNNs. These discrepancies signal the need for more work to make DNNs better psychological models for visual perception. Jacobs and Bates suggested that creating better training experiences for DNNs and limiting their processing powers to mimic limits in human systems (e.g., attentional mechanisms) will likely improve DNNs’ ability to become better models for psychological processes.
Identifying Objects and Remembering Images: Insights From Deep Neural Networks
Nicole C. Rust and Barnes G. L. Jannuzi
Current Directions in Psychological Science
Rust and Jannuzi summarized how researchers have used deep artificial neural networks to gain insights into how the high-level visual cortex contributes to object identification and image memorability—the systematic variation with which some images are remembered better than others. Important insights from this work include support for the idea that stacks of simple model neurons can recapitulate the core aspects of object-identification behavior, and the revelation that at least some component of image-memorability variation emerges from a system optimized for object categorization.
Searching for the Big Pictures
Stephen K. Reed
Perspectives on Psychological Science
Concerned with the impact of specialization in doctoral training, Reed described his attempt to discover new ways of organizing knowledge in psychological science that would have theoretical and practical implications. During this search, Reed wrote 10 integrative articles, five of which integrated advancements in artificial intelligence and cognitive psychology. Reed elaborated on his efforts to use formal ontologies to organize psychological knowledge and the strategies to write integrative articles as well as the role of integrations for making psychology relevant to a general audience.
Psychological Measurement in the Information Age: Machine-Learned Computational Models
Sidney K. D’Mello, Louis Tay, and Rosy Southwell
Current Directions in Psychological Science
Machine-learned computational models (MLCMs)—computer programs learned from data, typically with human supervision—are an emerging approach that combines computing and information sciences with real-world data and can be used to inform psychological science. D’Mello and colleagues compared MLCMs with traditional computational models and assessment in psychological science. They gave examples of MLCMs from cognitive and affective science, neuroscience, education, organizational psychology, and personality and social psychology. They also discussed the accuracy and generalizability of MLCM-based measures, privacy and security concerns associated with their use, and matters of data interpretability and fair use.
Machine Learning and Psychological Research: The Unexplored Effect of Measurement
Ross Jacobucci and Kevin J. Grimm
Perspectives on Psychological Science
Machine learning can benefit many areas of psychological science, such as those that use biological or genetic variables. However, more traditional areas of research have not benefited from machine learning. Jacobucci and Grimm suggested that this might be because of measurement errors that prevent machine-learning algorithms from accurately modeling the data. They provided simulated examples showing that measurement quality is very important for model selection in machine learning, and they advanced recommendations for better integration of machine learning with statistics in traditional psychological science.
A Conceptual Framework for Investigating and Mitigating Machine-Learning Measurement Bias (MLMB) in Psychological Assessment
Louis Tay, Sang Eun Woo, Louis Hickman, Brandon M. Booth, and Sidney D’Mello
Advances in Methods and Practices in Psychological Science
Machine-learning measurement bias (MLMB) can occur when a trained machine-learning model produces different predicted scores or score accuracy for different subgroups (e.g., race, gender) despite examining the same levels of the underlying construct (e.g., personality) in the groups. Both biased data and algorithms can be sources of MLMB. Tay and colleagues explained how these potential sources of bias may manifest and developed some ideas about how to mitigate them. The authors also highlighted the need to develop new statistical and algorithm procedures and put forward a framework for clarifying, investigating, and mitigating these complex biases.
Bringing AI to education: Intelligent tutoring systems
Learning by Communicating in Natural Language With Conversational Agents
Arthur C. Graesser, Haiying Li, and Carol Forsyth
Current Directions in Psychological Science
Tutoring is effective not simply because tutors are highly knowledgeable and can lecture students, but because tutors encourage students to generate answers to problems. Recently developed computer-based tutoring programs can simulate the conversation patterns used by human tutors and engage students in natural-language discussions. APS Fellow Graesser and his colleagues Li and Forsyth described the conversation patterns used in tutoring and how these have been implemented in intelligent tutoring systems.
Advancing the Science of Collaborative Problem Solving
Arthur C. Graesser, Stephen M. Fiore, Samuel Greiff, Jessica Andrews-Todd, Peter W. Foltz, and Friedrich W. Hesse
Psychological Science in the Public Interest
Graesser and colleagues encouraged the use of research findings in organizational and educational settings to inform possible approaches to collaborative problem solving in team training. They suggested that computer agents, which can be used to track and analyze conversation during collaboration, can be robust training tools. Emerging intelligent tutoring systems could automatically track the contributions of team members and the group as a whole and provide timely feedback and recommendations for improvement.
Advancing clinical psychology: From assessment to intervention
The Hitchhiker’s Guide to Computational Linguistics in Suicide Prevention
Yaakov Ophir, Refael Tikochinski, Anat Brunstein Klomek, and Roi Reichart
Clinical Psychological Science
Ophir and colleagues provided a comprehensive outlook on the integration of computational linguistics (CL) in suicide prevention. The authors focused on deep neural network models and described how CL methodologies (applied, for instance, to social-networking platforms) may contribute to early detection of suicide risk and hence prevent it. Research using CL may also deepen knowledge about suicide behaviors and promote personalized approaches to psychological assessment. Ophir and colleagues also discussed ethical and methodological concerns about the use of CL in suicide prevention, such as the difficulty of ensuring individuals’ privacy.
Predicting Imminent Suicidal Thoughts and Nonfatal Attempts: The Role of Complexity
Jessica D. Ribeiro, Xieyining Huang, Kathryn R. Fox, Colin G. Walsh, and Kathryn P. Linthicum
Clinical Psychological Science
Most of the past research on suicidal thoughts and behaviors (STBs) relied on long follow-ups and examined risk factors in isolation, but predicting suicide is complex. Ribeiro and colleagues recruited individuals worldwide who were at elevated risk of STBs, evaluating them in a first session and again 3, 14, and 28 days later. The researchers also used machine-learning algorithms to examine the complexity underlying suicide risk. Results indicated that machine-learning algorithms were better than individual follow-ups at predicting STBs. Some predictive factors, such as suicide ideation, were already strong predictors in more standard analyses, but complex models in this research were used to better predict imminent suicidal thoughts and nonfatal suicide attempts. Taken together, these findings support the use of complex models and inclusion of artificial intelligence in clinical decision-making.
Related content: The Emerging Science of Suicide Prevention
Digital Technologies for Emotion-Regulation Assessment and Intervention: A Conceptual Review
Alexandra H. Bettis, Taylor A. Burke, Jacqueline Nesi, and Richard T. Liu
Clinical Psychological Science
Bettis and colleagues examined the use of digital technologies to assess emotion regulation and create interventions. They reviewed technologies such as ecological momentary assessment, wearables and smartphones, smart-home technology, virtual reality, and social media. The use of these technologies allows researchers to study the dynamic nature of emotion regulation and its dependence on context and a person’s internal state, which the traditional methods of static self-report measurement do not allow. This capability has already led to changing the definition of emotion regulation to reflect the importance of flexibility across contexts. Bettis and colleagues discussed challenges, ethical considerations, and future research.
Perceiving AI: Improving AI’s usefulness
Artificial Intelligence and Persuasion: A Construal-Level Account
Tae Woo Kim and Adam Duhachek
Psychological Science
Kim and Duhachek found that messages by nonhuman artificial agents (AAs) are more persuasive when they highlight how an action is performed (e.g., “Apply sunscreen before going out”) rather than why an action is performed (e.g., “Using sunscreen means healthy skin”). Participants likely judged an AA’s actions as more appropriate when they represented how (i.e., low-level construals) as opposed to why (i.e., high-level construals) because they perceived the AA as lacking goals. However, when the AA showed learning capabilities, participants were more open to persuasion from high-level construal messages than low-level messages. This indicates that perceptions of learning capabilities may change people’s assumptions about AAs.
Artificial Intelligence and the Future of Work: A Functional-Identity Perspective
Eva Selenko, Sarah Bankins, Mindy Shoss, Joel Warburton, and Simon Lloyd D. Restubog
Current Directions in Psychological Science
Selenko and colleagues proposed a functional-identity framework to examine the effects of AI on people’s work-related experiences, including self-understandings and the social environment at work. They argued that the conditions for AI to either enhance or threaten workers’ sense of identity derived from their work depend on how the technology is functionally deployed (by complementing tasks, replacing tasks, and/or generating new tasks) and how it affects the social fabric of work. Selenko and colleagues proposed that AI-related changes to work affect how workers understand work, themselves in relation to work, and their social environment. See a related article here.
Concerns About Automation and Negative Sentiment Toward Immigration
Monica Gamez-Djokic and Adam Waytz
Psychological Science
The rise of sophisticated technology that can automate certain tasks not only threatens jobs but also appears to have social and psychological consequences, such as increasing negative attitudes toward immigration. In 12 studies, Gamez-Djokic and Waytz found that, both in the United States and in Europe, people who perceived automation as a greater threat to employment also tended to hold negative perceptions about immigrants. Automation concerns were also linked to support for restrictive immigration policies and, in the context of layoffs, an increase in discrimination against immigrants. See a related article here.
Feedback on this article? Email [email protected] or login to comment. Interested in writing for us? Read our contributor guidelines.
APS regularly opens certain online articles for discussion on our website. Effective February 2021, you must be a logged-in APS member to post comments. By posting a comment, you agree to our Community Guidelines and the display of your profile information, including your name and affiliation. Any opinions, findings, conclusions, or recommendations present in article comments are those of the writers and do not necessarily reflect the views of APS or the article’s author. For more information, please see our Community Guidelines.
Please login with your APS account to comment.