First Person
Back Page: Dumb (but Useful) AI, Smart Teams, and the Promise of Predictive Analytics
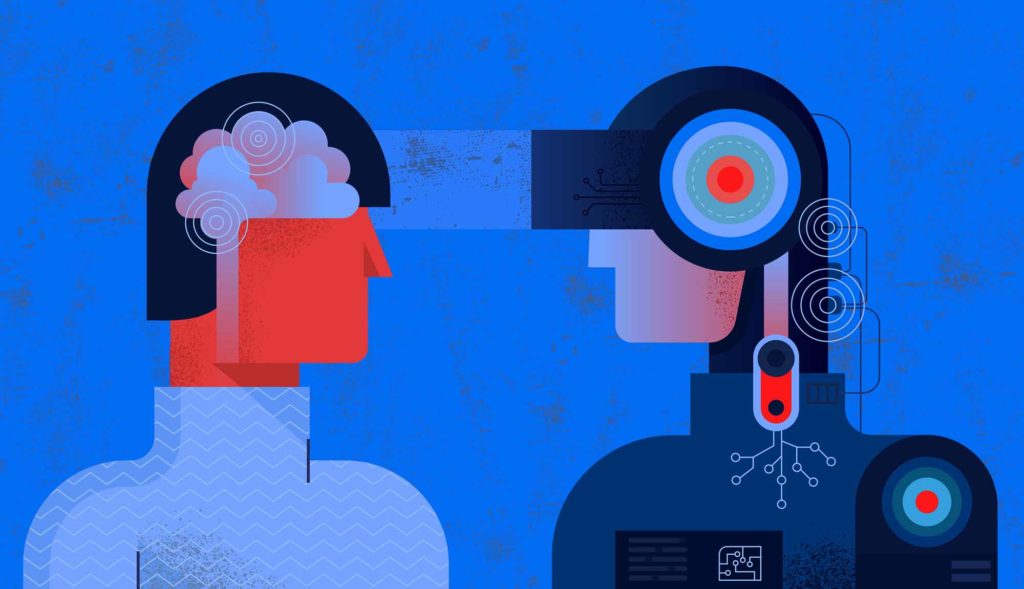
APS Fellow Steven W. J. Kozlowski is a World Class Scholar and professor of psychology at the University of South Florida. His research team at the ARCAS (Advanced Research on Complex Adaptive Systems) project uses computational modeling, a component of artificial intelligence, to study complex adaptive human organizational systems.
You began studying IO psychology as a graduate student in the late 1970s. Do you remember when you began thinking about the implications of AI for the workplace?
As a graduate student, I’d heard of AI; the concept was formed in the mid-1950s, so I was aware of it, along with information theory and cybernetics, but it wasn’t really central to anything that I was studying in organizational psychology. There was even a period where AI fell from favor because the technology didn’t advance nearly as quickly as some of the folks who coined the term thought it would.
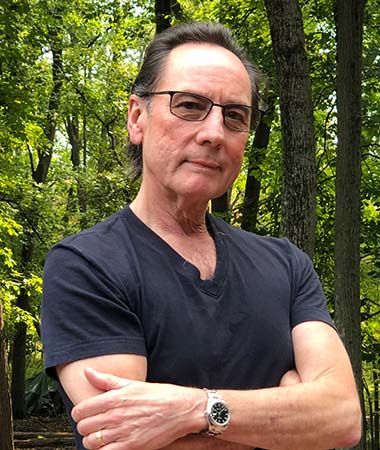
I think what’s gotten AI back on everyone’s radar is the internet, which creates lots of data that didn’t exist before. A lot of what we refer to as artificial intelligence today is really the application of statistical tools and techniques that are able to sift through massive amounts of data to look for nonlinear patterns and can find things that psychologists wouldn’t find with the typical statistics they use in their experiments or research studies. Computational modeling, the work I’m doing, is a methodology that generates data consistent with theories to see if the theories can explain what we observe in the real world—things that are difficult to make sense of using conventional psychological research methods.
What are some examples?
When I started as a grad student in organizational psychology, I was really interested in the idea of how individuals working together in organizations seem to create something that has collective properties but is not the property of any one person. Industrial and organizational (IO) psychology at the time was all about individual differences—my personality, my ability or physical characteristics or thoughts, my feelings and perceptions—and how that might relate to things that are relevant on the job: my motivation, my performance, my job satisfaction, my commitment, which was all about individuals. It didn’t tell me anything about the organization. That was my journey as a grad student: How do we study organizations in a way consistent with how we think about them, which is as dynamic systems?
My early career was devoted to something called multi-level theory. How do we theorize about individuals nested in groups or teams, and teams nested in departments or business units that comprise organizations that are linked together in some ways? This work began to get tractable around the turn of the century, coinciding with the advent of big data and the shift in the organization of work from individual jobs to more team-based or group-based workflows. That’s where interest in big data, artificial intelligence, and modeling began to develop. How do we look not just at these static nestings of people in larger entities now, but also how they play out dynamically over time? For instance, how might your feelings when you interact with your teammates influence others? If you’re in a good mood, how might that influence other people who are in sour moods? Could it cause them to develop a good mood and end up having a productive day?
The opposite can happen too when there’s that one person in your group who always has a sour attitude. Most people are able to insulate themselves from others’ moods, but sometimes it gets to you, and in fact that attitude can run through a team. That’s the stuff I’m interested in studying, and it’s hard to do with conventional psychological methods.
A more cutting-edge area of research in my field involves robots as teammates. I don’t know if I would think of a robot as anything more than a tool—a machine I can use—or as teammate. That depends on whether it embodies a variety of characteristics. But whether that AI is a software-based entity inside your computer that isn’t embodied, without a face or form, or a robot that is mobile and tangible and assistive, how we create those entities will impact how people respond to them. It could determine whether they’re seen as useful or threatening tools or in fact friendly things that we want to name and treat like they’re our teammates.
You wrote this in your response to our questionnaire: “AI represents an opportunity to advance the future of work, reducing dangerous work and drudgery and enabling support and coaching to enhance human well-being and effectiveness.” Can you elaborate on enabling support and coaching to enable well-being and effectiveness?
A couple of decades ago, I was doing research on complex skill acquisition. How do you help people learn complicated things better? Let’s say you create software agents that can monitor how people are working. They can give feedback, corrective suggestions. An example is intelligent tutors—a fairly well-developed technology for well-defined domains where we want people to learn knowledge and skills. Using computer-based entities to help people learn how to do things is one application of what I call a “dumb AI”—but a very useful AI.
A lot of what is now called AI is also being used for decision support. Some of my research is funded by the military, which tends to be interested in teams and high reliability, as people are put in very stressful situations where mistakes can be dangerous. Imagine you’re a weapons operator and you’ve got a lot of information to sort through. We know that people are not as good at that as computers. An AI agent could help you identify the high-priority things you should be tracking. It could also give you advice about different options or alternatives to consider. And it might monitor your decisions to see if they make reasonable sense given the data that’s available.
Increasingly that decision support could be relevant to more and more of us who are doing tech-based work. Even simple things like Zoom meetings, where everybody’s on a virtual team. I know something about running team meetings, and a lot of the Zoom meetings that I’m on are horrible because they’re poorly run by the folks who organize them. Suppose there was a little agent in Zoom who could give the meeting convener tips of the trade, so to speak, about how to set up a meeting, how to run an agenda, how to keep tabs on the meeting, how to make sure it’s productive.
That wouldn’t be really hard to do, and it wouldn’t take a great deal of “AI.” A lot of this would be pretty dumb AI that can be basically built into a tool. Although a lot of those rollouts are branded as AI, I would just say, well, they’re not really intelligent, but they are useful. They’re very useful tools to help people do what they’re doing better.
Do you think the use of the word intelligence is overused in the context of AI?
I’m sure you can find folks to support one side or the other, but to my sense, these entities and software aren’t really creating anything new. They’re basically applying patterns they’ve seen before that work well in a particular situation. This is the application of predictive analytics, and I’m not saying that isn’t an aspect of intelligence. Somebody who is shrewd may be able to read a room and evaluate information more accurately and more quickly than folks who are disinterested. We might consider that to be intelligence, but it’s really harnessing a lot of information in order to make some choices about words to string together and how to respond in a conversation.
Tell us about your work with the ARCUS project. Can you describe some ways in which computational modeling has advanced understanding of complex adaptive human organizational systems?
Arcus is pretty new, so I don’t have a good example to report from that project. However, in prior work our research group was looking at small groups of problem-solving experts to see how they pull information together to address problems when they arise. Experts have knowledge of particular domains and access to certain assets, tools, or potential solutions. This is a very common situation in organizations such as the military, medicine, and management, where there might be three different experts pulled together to solve one particular problem. They’re under time pressure, so what can we do to help them?
Click here for a related article on the dynamics of team cognition.
There are a couple of key steps in this process. One is getting all the right information out of the problem space so that it can be dealt with. Another is the experts sharing their specialized takes on what they know, given the information they collected, to create a common understanding and hence a basis for a solution.
Using computational modeling, we built a fairly simple model that helped us look at how agents—software entities—went about learning about a decision space. We found that there were particular points in the process where they tended to get stuck. Using the insights from doing modeling with agents, we were able to build some interventions that were programmed into a computer to allow three humans working on a task in a lab go into a problem space, extract information, collect it, share it, and make a decision. We were able to use the insights from the modeling to rectify a lot of the bottlenecks in this process. They were dumb entities, but they were built into the system in a way that helped these teams make better decisions.
Your lab at USF takes a team science approach, assembling experts with different but complementary multidisciplinary capabilities. Can you provide an example of how this approach can advance understanding in ways that less diverse teams cannot?
Well, I’m not a computational modeler. I’m a theoretician who is interested in modeling dynamics. I can identify the methodology and how to use it, but I need to be able to collaborate with people who can code. So, I work with computer scientists, because they have a different suite of tools. Another example is that I can generate data that helps me get at team dynamics over lengthy periods of time, but the analytics needed to extract meaning from that data are not available from the analytical tools that are typical in psychology.
Similarly, in a project with NASA, we were collaborating with computer scientists and engineers to build a technology you might think of as a badge—a wearable socio-meter that keeps track of who you’re interacting with and assesses your heart rate. We could essentially track on a near real-time basis how well the team was interacting. It got to the point of a well-developed prototype that allowed us to essentially replicate the data we were collecting by asking people questions. People would go to a mission simulation—a stressful environment—for a year, and on a daily basis would give us information about how cohesive the team was or how complex their interactions were.
These are difficult studies to run because they’re small groups of six people over 8 to 12 months. You’re collecting a lot of data on very few cases. But for folks in these environments, things can start well and deteriorate across the mission. These simulations are intended to answer, “How can we help astronauts go into Mars for three-year missions?” They suggest that things will get difficult right about the time we get to Mars. We were essentially able to detect the same pattern that we observed in the daily rating data with the data we had gotten from the badge. And, with further development, we would have been able to build agents into the system to coach and advise team members to better maintain their interpersonal relationships under very stressful conditions. Even agents that aren’t particularly intelligent can be leveraged to do very useful things.
Back Page showcases particularly interesting work by a wide variety of psychological scientists. Know of a good candidate for a future profile? Contact the Observer at [email protected].
Feedback on this article? Email [email protected] or login to comment. Interested in writing for us? Read our contributor guidelines.
APS regularly opens certain online articles for discussion on our website. Effective February 2021, you must be a logged-in APS member to post comments. By posting a comment, you agree to our Community Guidelines and the display of your profile information, including your name and affiliation. Any opinions, findings, conclusions, or recommendations present in article comments are those of the writers and do not necessarily reflect the views of APS or the article’s author. For more information, please see our Community Guidelines.
Please login with your APS account to comment.