Cover Story
The Bias Beneath: Two Decades of Measuring Implicit Associations
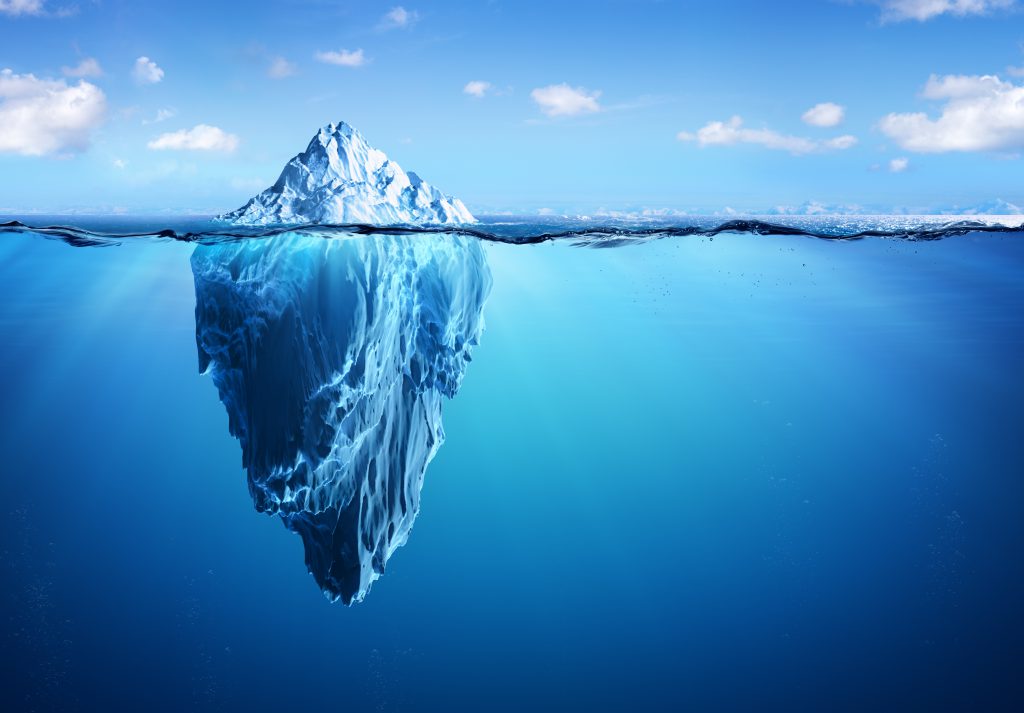
Over the last 20 years, millions of people have used an online test to probe attitudes they didn’t know they had.
Since its online debut in 1998, the Implicit Association Test (IAT) has allowed people to discover potential prejudices that lurk beneath their awareness — and that researchers therefore wouldn’t find through participant self-reports.
Basically, the IAT asks participants to categorize words or images that appear onscreen by pressing specific keys on a keyboard. The time it takes for participants to respond to different combinations of stimuli is thought to shed light on the mental associations they make, even when they aren’t aware of them.
The IAT is the brainchild of APS William James Fellow Anthony Greenwald (University of Washington), and he began working collaboratively on it with APS Past President Mahzarin Banaji (Harvard University) and APS Fellow Brian Nosek (University of Virginia) in the mid-1990s. Over time, the tool has led to the examination of unconscious and automatic thought processes among people in different contexts, including employers, police officers, jurors, and voters.
Perhaps the most salient examples of implicit bias involve race and gender across a variety of scientific perspectives. APS Past President Elizabeth Phelps has collaborated considerably with Banaji on IAT investigations using functional MRI (fMRI) to explore the brain’s role in the unconscious evaluation of racial groups. Developmental researchers have modified the IAT for use with children to discover some intergroup associations that form in the earliest years of life. (See related story on page 15.) And data from Project Implicit reveal that 75% of people who have taken the IAT have correlated men more strongly with work roles and women more strongly with family positions. A recent study showed that hiring managers whose scores on the IAT indicated gender bias tended to favor men over women in their hiring decisions.
But the IAT has also inspired a wealth of research on implicit biases related to age, weight, political leanings, disability, and much more.
Opinions on the IAT are mixed. Controversy about the test was evident in a 2013 meta-analysis by APS Fellows Fred Oswald and Phillip E. Tetlock and colleagues. They found weaker correlations between IAT scores and discriminatory behavior compared with what Greenwald, Banaji, and their colleagues found in a 2009 meta-analysis.
As researchers continue to explore how to use and interpret IAT findings (a new, larger meta-analysis is being prepared for publication), there’s no question that the test has shaped public discussions about race and discrimination. Hillary Clinton discussed implicit bias during one of the debates in the 2016 presidential election campaign. The US Department of Justice (DOJ) has integrated findings about implicit bias into training curricula for more than 28,000 DOJ employees as a way of combating implicit bias among law enforcement agents and prosecutors. And in a historic 2015 decision involving fair housing, the US Supreme Court referenced implicit bias in a ruling allowing federal action against housing policies that have a disparate impact as well as being overtly discriminating.
“The research of Mahzarin, Tony, and their collaborators has changed national and even international conversations about racism, sexism, classism, and other forms of bias, very much for the better,” says APS Fellow John Jost, Codirector of the Center for Social and Political Behavior at New York University and a former student of Banaji’s.
In this issue of the Observer, we mark the 20th anniversary of the IAT’s debut with examples of the studies it has spawned across numerous areas of psychological study.
Obesity
Studies have used the IAT to investigate how weight stereotypes affect people who are overweight or obese. In a 2011 psychological field experiment, for example, scientists at Linnaeus University in Sweden found evidence of hiring discrimination against heavier individuals. Experimenters sent out fictitious applications for a large number of actual advertised job openings. The applicants all included their photographs and had the same credentials, but some of the photos showed the job-seekers as obese and others as normal weight. The researchers then compared the number of callbacks received by the normal-weight applicants and the obese applicants. Later, the hiring managers who received the applications were invited to take an obesity IAT as well as measures of their explicit hiring preferences. The researchers found that recruiters who showed the most implicit versus explicit negative associations with obesity were the least likely to have invited an overweight applicant for an interview.
These biases about weight may also play a role in the way medical doctors view their patients, according to findings from a multidisciplinary research team that included UVA’s Nosek. The scientists tested nearly 400,000 participants, including more than 2,000 MDs. They found that doctors are just as biased against obesity as is the general public. Specifically, the MDs reported a strong preference for thin people over overweight people on measures of both explicit and implicit attitudes. But IAT results revealed that male MDs had a considerably stronger implicit bias against overweight individuals compared with their female counterparts. The scientists said the results called for further exploration into any link between provider biases about weight and patient reports of weight discrimination in their health care.
Suicide Risk
Even experienced clinical judgment often misses the marks of suicidal thinking. As a result, suicide experts have long hoped and searched for a behavioral marker of suicide risk. With Banaji, Harvard psychological scientist Matthew Nock and other clinical researchers decided to adapt the IAT to examine whether the test might reveal implicit signs of suicide risk.
Nock and colleagues tested 157 psychiatric patients, including those who were brought to the hospital following a suicide attempt. The scientists wanted to see if the IAT could distinguish those who had tried to kill themselves from those who had not.
While in the emergency room, the patients rapidly classified words related to “me” (e.g., I, me) and “not me” (e.g., they, them) as well as “life” (e.g., survive, live) and “death” (e.g., dead, dying). The researchers examined how quickly patients connected identity-related words to life-or-death words. They found that patients who had attempted suicide prior to admission responded more quickly to word pairs linking the self and death than they did to other word pairs, suggesting that the unconscious association between self and death was stronger for these patients.
Nock followed the patients for 6 months and found that those who showed a relatively strong self–death association in the hospital were significantly more likely to attempt suicide later compared with those who showed a weaker self–death association. The responses on the IAT predicted suicide attempts above and beyond the effects of commonly used predictors such as a depression diagnosis, previous suicide attempts, or the attending clinician’s intuition.
Romantic Attachment
Much of the research on relationship success has relied on self-reports, but some scientists have developed IAT-like tools to assess implicit appraisals of romantic partners. In a study reported in 2010, for example, University of Rochester researchers, including APS Fellow Harry Reis, recruited 222 volunteers involved in romantic relationships. Each volunteer supplied their partner’s first name and two other words, such as a pet name or a distinctive characteristic, which related to the partner. Then they watched a monitor as three types of words were presented one at a time — “good” words (such as peace, vacation, or sharing), “bad” words (such as death, tragedy, and criticizing), and partner-related words (e.g., names or traits).
In one kind of test, volunteers pressed the space bar whenever they saw either good words or partner-related words. In the other, they responded when they saw bad words paired with partner words. The expectation was that participants who had generally positive associations with their partners should be able to complete the first task more easily than the second.
The results showed that volunteers who were relatively quick to respond to bad word–partner pairings and relatively slow to respond to good-word–partner pairings were more likely to separate from their partner over the next year. Furthermore, the test results were a stronger predictor of later breakup than were the volunteers’ own evaluations of their relationship quality.
In a typical IAT, a person sits at a computer screen and views a series of words and images. She’s told to press the I key on the keyboard when she sees an upbeat word such as happy or pleasant and the E key for negative words such as dangerous or tragic. The person then is told to press I when she sees the face of a Black man and E when she sees a White man’s face. Next she presses I when she sees a positive word or a Black face and E when she sees a negative word or a White face. The process then reverses to Black face/negative word versus White face/positive word. All the while, the computer records the person’s response times to each stimulus and, at the test’s conclusion, calculates an IAT score based on these data.
Attitudes About Sexuality
Researchers have also been able to use IAT data to track shifts in implicit intergroup attitudes over time, including attitudes toward homosexuality. Public opinion polls have indicated that acceptance of gay men and women has increased as they have gained more legal rights and protections, but those polls only capture explicit attitudes. IAT cocreator Nosek and psychological scientist Erin Westgate of UVA, along with Rachel Riskind of Guilford College in North Carolina, investigated how implicit biases toward gay people have shifted.
The scientists examined test data from nearly 684,000 visitors to the Project Implicit site between February 2006 and August 2013. Eighty percent of the participants identified as heterosexual.
When taking the IAT, participants had to sort positive words (e.g., beautiful, good) into the “good” category and negative words (e.g., bad, terrible) into the “bad” category. They then did the same kind of sorting for words and images related to gay people (e.g., pictures of same-sex wedding cake toppers or the word homosexual) and straight people (e.g., the word heterosexual). Participants who had negative implicit associations with gay people reacted more slowly when positive words were paired with words related to gay people than did those who had positive implicit associates with gay people.
The researchers found that not only did explicit preferences for straight people over gay people decline by 26% over the 7.5 year period, implicit preferences fell by more than 13% during that same time period. That change was largest among people who were younger, White or Hispanic, and liberal. But nearly every demographic group in the sample showed signs of an attitude shift.
Political Preferences
Voters have increasingly eschewed the Democrat and Republican labels and have opted to identify themselves as Independents. But Nosek and UVA psychological scientist Carlee Beth Hawkins decided to use the IAT to explore the associations that churn inside the Independent mind.
In one study, a random sample of more than 1,800 volunteers participated on the Project Implicit website, where they read a mock newspaper article comparing two competing welfare proposals. One plan was generous in its benefits, the other much more stringent. Some of the volunteers read an article that said the Democrats were supporting the generous plan; Republicans, the stringent plan. Other participants read the same article but with the parties switched around.
The researchers then asked the volunteers to record which proposal they preferred and describe their political ideology and party identification; those who selected Independent were asked if they leaned toward either of the two major parties. Next, the volunteers took a version of the IAT designed to measure partisan identities and policy preferences.
The participants who identified as Independents varied greatly in the implicit associations they showed, and they made political judgments in line with these implicit associations. Those Independents who implicitly identified with Democrats preferred the liberal welfare plan, while those who implicitly identified with Republicans preferred the stringent plan. Furthermore, the Independents who showed implicit associations that favored Republican politics preferred whatever plan was proposed by Republicans — regardless of the values underlying the plan — more than they favored any plan proposed by Democrats. The same was true for those who showed an implicit preference for Democrats.
The findings suggest that self-identified Independents appeared to be influenced both by ideology and by partisanship, the researchers concluded.
References
Agerström, J., & Rooth, D. O. (2011). The role of automatic obesity stereotypes in real hiring discrimination. Journal of Applied Psychology, 96, 790–805. doi:10.1037/a0021594.
Banaji, M. A., & Greenwald, A. G. (2013). Blindspot: Hidden biases of good people. New York, NY: Delacorte Press.
Greenwald, A. G., & Banaji, M. R. (1995). Implicit social cognition: Attitudes, self-esteem, and stereotypes. Psychological Review, 102, 4–27.
Greenwald, A. G., Banaji, M. R., & Nosek, B. A. (2015). Statistically small effects of the Implicit Association Test can have societally large effects. Journal of Personality and Social Psychology, 108, 553–561.
Greenwald, A. G., McGhee, D. E., & Schwartz, J. L. (1998). Measuring individual differences in implicit cognition: The implicit association test. Journal of Personality and Social Psychology, 74, 1464–1480.
Greenwald, A. G., Poehlman, T. A., Uhlmann, E. L., & Banaji, M. J. (2009). Understanding and using the implicit association test: III. Meta-analysis of predictive validity. Journal of Personality and Social Psychology, 97, 17–41. doi:10.1037/a0015575.
Hawkins, C.B., & Nosek, B.A. (2012). Motivated independence? Implicit party identity predicts political judgments among self-proclaimed independents. Personality and Social Psychology Bulletin, 38, 1441-1455, doi: 10.1177/0146167212452313.
Lee, S., Rogge, R.D., Reis, H.T. (2010). Assessing the seeds of relationship decay: Using implicit evaluations to detect the early stages of disillusionment. Psychological Science, 21, 857-864. doi.org/10.1177/0956797610371342
Nock, M.K., Park, J.M., Finn, C.T., Deliberto, T.L., Dour, H.J., Banaji, M.R. (2010). Measuring the suicidal mind: implicit cognition predicts suicidal behavior. Psychological Science, 21(4), 511-517, doi:10.1177/0956797610364762.
Oswald, F. L., Mitchell, G., Blanton, H., Jaccard, J., & Tetlock, P. E. (2013). Predicting ethnic and racial discrimination: A meta-analysis of IAT criterion studies. Journal of Personality and Social Psychology, 105, 171–92. doi:10.1037/a0032734.
Sabin, J.A., Marini, M., & Nosek, B.A. (2012). Implicit and explicit anti-fat bias among a large sample of medical doctors by BMI, race/ethnicity and gender. PLoS ONE, 7(11), e48448. doi: 10.1371/journal.pone.0048448.
Reuben, E., Sapienza, P., & Zingales, L. (2014). How stereotypes impair women’s careers in science. Proceedings of the National Academy of Sciences, 111, 4403-4408. doi:10.1073/pnas.1314788111
Westgate, E. C., Riskind, R. G., & Nosek, B. A. (2015). Implicit preferences for straight people over lesbian women and gay men weakened from 2006 to 2013. Collabra, 1. doi:10.1525/collabra.18
Comments
Interesting that all of the research demonstrating the poor reliability and poor validity of the measure is missing from this article. Any research using the IAT must be interpreted with these things in mind: a) IAT is poorly correlated with other measures of prejudice including other implicit measures, b) test-retest reliability is low, c) IAT doesn’t predict behavior
I think the meta-analysis by Oswald, Mitchell, Blanton, Jaccard, & Tetlock (2013) refutes (a), depending on what you consider “poorly” correlated. It also refutes (c), although the prediction of behavior is not large. I think what we can confidently say about IAT/Explicit/Discrimination is that there is a lot of error variance and unexplained variance that we need to understand better.
Is there a reference for the Banaji & Nock work on suicidal intent, please?
Nock, M. K., Park, J. M., Finn, C. T., Deliberto, T. L., Dour, H. J., & Banaji, M. R. (2010). Measuring the suicidal mind: Implicit cognition predicts suicidal behavior. Psychological Science, 21(4), 511–517. https://doi.org/10.1177/0956797610364762
I actually did the word association thing with the black and white faces for a class once. I totally forgot about it till I read this. What a small world.
APS regularly opens certain online articles for discussion on our website. Effective February 2021, you must be a logged-in APS member to post comments. By posting a comment, you agree to our Community Guidelines and the display of your profile information, including your name and affiliation. Any opinions, findings, conclusions, or recommendations present in article comments are those of the writers and do not necessarily reflect the views of APS or the article’s author. For more information, please see our Community Guidelines.
Please login with your APS account to comment.