Featured
Experimental Methods Are Not Neutral Tools
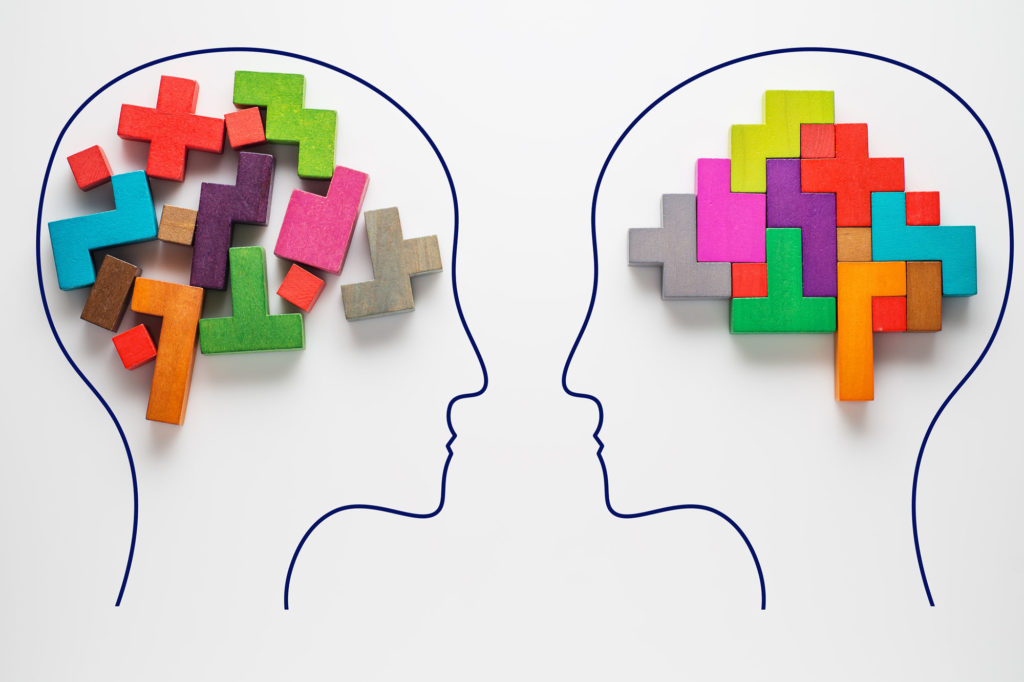
For more than 50 years, psychological scientists have conducted countless experiments demonstrating, time and time again, just how hopeless people seem to be at statistical reasoning.
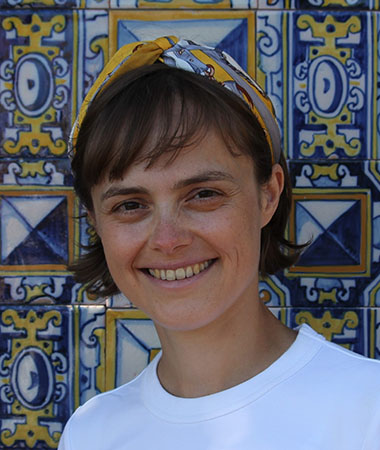
Researchers have found that people tend to overestimate the degree to which others agree with them (Ross et al., 1977). They have also discovered that people tend to believe it is more likely that two events will occur simultaneously than that just one will occur (Tversky & Kahneman, 1983). And they have shown that people tend to overestimate the likelihood of events that come to mind easily, such as tragic but rare events that burn themselves into memory despite their low frequency (Tversky & Kahneman, 1973).
Such findings have persuaded psychologists and economists that people are incapable of sound statistical reasoning and decision-making—that our mental software is dysfunctional and hopelessly irrational. According to Nobel laureate Richard Thaler (1994), people are influenced by so many cognitive biases or mental illusions that these shortcomings “should be considered the rule rather than the exception.”
This view has potentially significant social and political implications, and we believe it is mistaken. We argue that many experimental psychologists have painted too negative a picture of human rationality, and that their pessimism is rooted in a seemingly mundane detail: methodological choices.
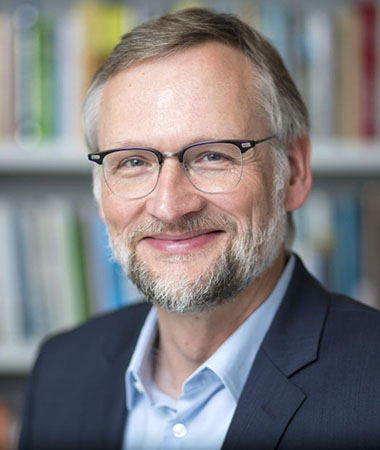
Experimental methods are not neutral tools. They influence participants’ statistical reasoning and, by extension, the conclusions experimenters draw about human rationality. For years, the research community has relied mostly on a single experimental protocol—one that by design precludes practice and learning from experience. This homogeneity has contributed, in our view, to a lopsided perspective on people as irrational beings.
Different methods lead to different views
The view of the irrational mind is linked to psychology’s most influential research program of the last 50 years. The heuristics-and-biases program started in the 1970s, spurred by Amos Tversky and Daniel Kahneman. The key idea is that people have cognitive limitations that make them unable to perform rational calculations. Instead, people rely on heuristics, which are easier to apply but can lead to systematic errors. The program produced a long list of ways that people’s judgments systematically deviate from norms of rational reasoning.
But previously, another research program—the intuitive statistician program—had reached a very different conclusion. Just like the findings from the heuristics-and-biases program, the intuitive statistician program’s findings were not a matter of opinion; they were also based on experimental data.
This intuitive statistician program found enough correspondence between people’s judgments and the predictions of normative models (i.e., rules from probability theory and statistics) to conclude that the models could provide a basis for theories of how people reason about probabilities. Indeed, in a 1967 review of more than 160 experiments, psychologists Cameron Peterson and Lee Roy Beach concluded that people can make reasonably accurate inferences about probabilities. People, the researchers argued, are intuitive statisticians.
How could psychology change its conclusions about human statistical reasoning so swiftly and dramatically? After all, people did not suddenly become inept at experimental tasks.
An analysis from our lab examined the methods of more than 600 experiments from both lines of research (Lejarraga & Hertwig, 2021). The analysis showed that Tversky and Kahneman established a completely new experimental protocol to measure statistical intuitions that was subsequently adopted throughout the research community.
In the 1960s, researchers used an experiential protocol that allowed people to learn probabilities from direct experience. Usually, people could practice, sample information sequentially, and adjust responses continually with feedback.
But Tversky and Kahneman replaced this experiential protocol with a descriptive one. Their experiments presented people with descriptive scenarios and word problems and tended to ask for a one-off estimate or judgment. Participants in their studies had little opportunity to practice or learn from feedback.
We believe that this change in experimental protocol was a key factor in the move from seeing people as intuitive statisticians to seeing them as irreparably irrational.
The case of Bayesian reasoning
Consider how the two protocols have been used to study Bayesian reasoning—the ability to update the predicted probability of an event (e.g., having a disease) after acquiring new information (e.g., a positive test result).
Under the experiential protocol, people were shown a sample of real poker chips, drawn one at a time. The poker chips could be red or blue. After seeing each chip, people were asked for their estimate of the probability that the sample of poker chips had been taken from one of two bags: one bag in which 70% of the chips were red and 30% were blue, and another bag in which 70% were blue and 30% were red. Participants were allowed to revise their answer after seeing each of the poker chips. This procedure was repeated many times using different samples and different base rates of blue and red chips.
Tversky and Kahneman, by contrast, used the descriptive protocol to study Bayesian reasoning. For instance, they had participants read five descriptions of fictitious individuals, allegedly drawn at random from a population of 30 engineers and 70 lawyers (or 70 engineers and 30 lawyers). Some of the descriptions evoked the stereotype of an engineer, others evoked the stereotype of a lawyer. For each description, the participants were asked to indicate the probability that the person was an engineer.
Unlike the experiential protocol, all relevant information about the sample was verbally described and delivered in one go. Learning from experience was neither necessary nor possible, and no feedback was provided.
The two experiments were purported to measure the same competence but yielded conflicting results. The experiential protocol found that people were essentially Bayesian reasoners, except that they gave too much weight to base rates in revising their beliefs. The descriptive protocol, in contrast, found that people’s reasoning was not at all Bayesian. People neglected the base rates, instead judging the probability that someone was an engineer on the basis of how closely their description matched the stereotype of an engineer—a phenomenon known as the base-rate fallacy.
Here’s the rub: The experimental methods researchers use in the lab are not neutral tools. They can shape people’s judgments. People do not seem to be very good at solving word problems about probabilities—at least not without explicit instruction. But they do seem to be reasonably capable of intuitive statistical reasoning when given the opportunity to learn through practice and direct experience, adjusting their judgments with each new observation. Experience matters.
Methods are not neutral—and neither are scientists
Given these two experimental protocols, one of which yields a more positive view of human rationality than the other, one might expect scientists to reckon with both streams of evidence and investigate the benefits and limitations of acquiring statistical information from description or experience.
This is not what happened. An analysis of scientific articles published between 1972 and 1981 showed that articles reporting poor performance were almost six times more likely to be cited than articles reporting good performance (Christensen-Szalanski & Beach, 1984).
One reason why negative evidence may have won out is that the descriptive protocol of Tversky and Kahneman, with its minimal setup and implementation costs, made it easier to collect data—ultimately overshadowing the findings of the 1950s and ’60s.
What’s more, the way negative evidence has been interpreted reveals a tendency among psychologists to overattribute people’s behavior in experiments to dispositional characteristics (namely, people’s cognitive limitations) while deemphasizing the role of the experimental context (e.g., how statistical information is represented and whether sequential learning is allowed).
The tendency to interpret people’s behavior in this way corresponds to a phenomenon studied in social psychology called the fundamental attribution error. Psychologists are human beings, and so they may not be immune to the cognitive biases they study.
The selective emphasis on poor performance and cognitive limitations has spilled over from psychology into economics and even public policy. Policymakers take for granted that people’s cognitive illusions are so pervasive and so persistent that it is more efficient to work with those errors (using simple nudges) than to try to overcome them.
Most nudges involve changing aspects of the choice environment that people typically claim not to care about in order to steer people’s behavior in the right direction. Examples of classic nudges include the redesign of cafeterias to display healthier food at eye level and the automatic enrollment of individuals in pension plans unless they specifically choose to opt out.
Whereas nudges steer people’s behavior while leaving their competences unchanged, the research program of the intuitive statistician sheds a more optimistic light on people’s capacity to learn.
Related content from the March/April 2022 issue: How a Nudge Can Make a Habit: The Subversive Nonchalance of Small Changes
Learning from the past
The conflicting views on rationality that stem from different experimental protocols highlight the risk of relying exclusively on a single approach. We believe that a combination of descriptive and experiential protocols would yield a more complete understanding of people’s competences and rationality than either protocol could alone.
Researchers should investigate the wide variety of situations in which people deal with probabilities in domains such as health and finance, and take into consideration aspects such as practice, feedback, learning opportunities, and financial incentives.
Researchers should also continue to use descriptive protocols, side by side with experiential ones, to investigate how different ways of representing statistical information influence people’s reasoning. Changing the representation of statistical information—from probabilities to natural frequencies, from relative to absolute risks, from numerical to graphical representations, from descriptive to experiential formats—can make people more competent at reasoning in a Bayesian way.
The combined use of descriptive and experiential protocols would also help shed light on the development of statistical reasoning across the lifespan and across species. Developmental psychologist and APS President Alison Gopnik once asked, “Why are grown-ups often so stupid about probabilities when even babies and chimps can be so smart?” (Gutíerrez, 2014). A review article from our lab suggests that experimental protocols may be a key factor in understanding this discrepancy (Schulze & Hertwig, 2021). Whereas studies with adults typically use descriptive protocols, the protocols used with infants and, similarly, with nonhuman animals tend to involve firsthand experience of information.
In our view, a combination of descriptive and experiential protocols is preferable to either protocol used alone. Moreover, the rationality debate must not be dissociated from the choice of experimental protocol. When researchers draw conclusions from behavioral data, they must take their choice of experimental protocol and the resulting limits on generalizability into account. Whether or not it is legitimate to generalize findings from a descriptive to an experiential protocol and vice versa must be empirically established, not simply assumed.
Let us conclude with one last call: The debate about people’s statistical intuitions has important implications for how citizens can be supported in making better decisions. Research based on the experiential protocol justifies more optimism toward interventions designed to improve people’s competences than has been assumed by the nudging approach. It is time for policymakers to fully explore the potential of boosting, a policy approach informed by behavioral science that aims to foster people’s competences to make good choices while respecting their agency and autonomy (see, e.g., Hertwig & Grüne-Yanoff, 2017). Both approaches, nudging and boosting, should have their place in the policymaker’s toolbox.
Acknowledgments:
The authors thank Deb Ain, Gerd Gigerenzer, Susannah Goss, Tomás Lejarraga, Lael Schooler, and Jolene Tan-Davidovic for many helpful comments on an earlier version of this article.
Feedback on this article? Email [email protected] or login to comment.
References
Christensen-Szalanski, J. J. J., & Beach, L. R. (1984). The citation bias: Fad and fashion in the judgment and decision literature. American Psychologist, 39(1), 75–78. https://doi.org/10.1037/0003-066X.39.1.75
Gutíerrez, L. (2014, January 10). The surprising probability gurus wearing diapers. The Wall Street Journal. https://www.wsj.com/articles/SB10001424052702303393804579308662389246416
Hertwig, R., & Grüne-Yanoff, T. (2017). Nudging and boosting: Steering or empowering good decisions. Perspectives on Psychological Science, 12(6), 973–986. https://doi.org/10.1177/1745691617702496
Lejarraga, T., & Hertwig, R. (2021). How experimental methods shaped views on human competence and rationality. Psychological Bulletin, 147(6), 535–564. https://doi.org/10.1037/bul0000324
Peterson, C. R., & Beach, L. R. (1967). Man as an intuitive statistician. Psychological Bulletin, 68(1), 29–46. https://doi.org/10.1037/h0024722
Ross, L., Greene, D., & House, P. (1977). The “false consensus effect”: An egocentric bias in social perception and attribution processes. Journal of Experimental Social Psychology, 13(3), 279–301. https://doi.org/10.1016/0022-1031(77)90049-X
Schulze, C., & Hertwig, R. (2021). A description–experience gap in statistical intuitions: Of smart babies, risk-savvy chimps, intuitive statisticians, and stupid grown-ups. Cognition, 210, 104580. https://doi.org/10.1016/j.cognition.2020.104580
Thaler, R. H. (1994). Quasi rational economics. Russel Sage Foundation.
Thaler, R. H., & Sunstein, C. R. (2021). Nudge: The final edition. Yale University Press.
Tversky, A., & Kahneman, D. (1973). Availability: A heuristic for judging frequency and probability. Cognitive Psychology, 5(2), 207–232.
Tversky, A., & Kahneman, D. (1983). Extensional versus intuitive reasoning: The conjunction fallacy in probability judgment. Psychological Review, 90(4), 293–315. https://doi.org/10.1037/0033-295X.90.4.293
Comments
DGPS
Retired - No Journals
Member Since 01/20/2010
An excellent contribution to a special field of psychology that has, after all, been awarded Nobel Prizes. The conclusions drawn from experimental results are not consistent with substantively comparable results from other empirical studies, even when Nobel Prizes are awarded for the experimental studies. So which results are correct? Is it the experimental method that falsified the results? If different methods lead to different results and this is consistent in itself, then both results can be “true”, they just tested different things. Unfortunately, we don’t validate experimental settings, so we don’t know what was varied. It’s more about the validity of the setting in general. (This is true for all other experimental conditions as well.) To do this, we need theory, a more comprehensive validation strategy, and valid measurements of theoretical constructs. Only then can we derive relevant conclusions and make recommendations. At the same time, the statements and recommendations must also be externally valid. So, it is not the tool that is the problem but the lack of validation and theory. The following applies: Different tools can have different validity, the same tools can have different validity, different tools can have the same validity, and the same tools should have the same validity. You must check this intensively. The manipulation check is not a validity check.
APS regularly opens certain online articles for discussion on our website. Effective February 2021, you must be a logged-in APS member to post comments. By posting a comment, you agree to our Community Guidelines and the display of your profile information, including your name and affiliation. Any opinions, findings, conclusions, or recommendations present in article comments are those of the writers and do not necessarily reflect the views of APS or the article’s author. For more information, please see our Community Guidelines.
Please login with your APS account to comment.