Featured
What’s in a Word? Computational Modeling Puts the Science of How We Learn Language to the Test
Since the cognitive revolution of the 1950s, psychological scientists have iterated on classic findings to advance a new understanding of how we learn to speak and read.
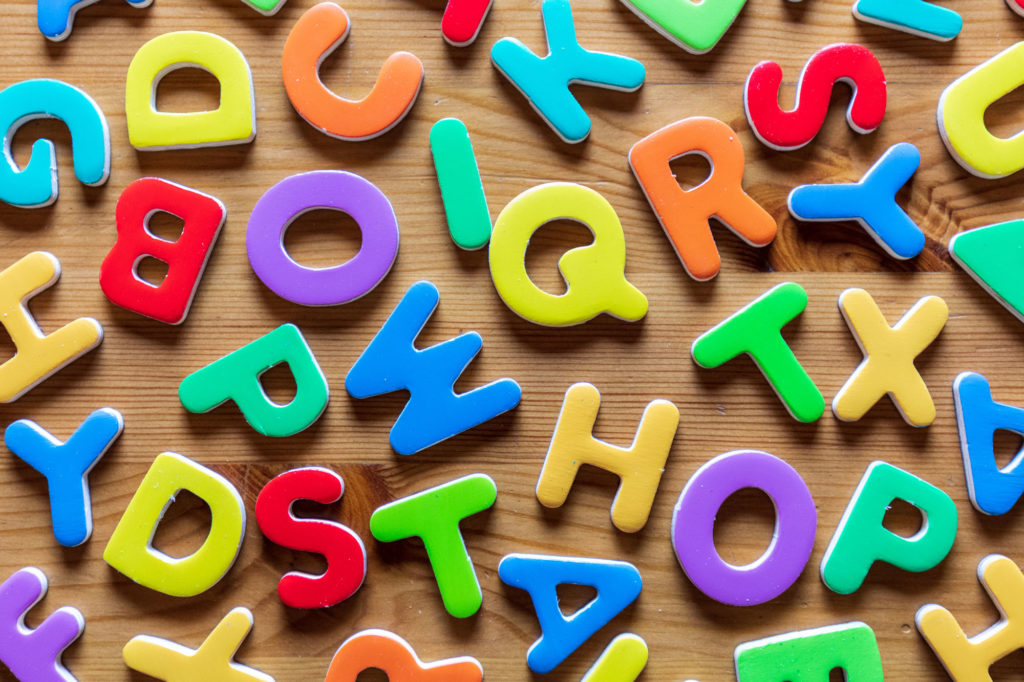
“What’s in a name? That which we call a rose / by any other name, would smell as sweet.” As Shakespeare recognized in this classic line from Romeo and Juliet, a name—or any other word, for that matter—is just a collection of sounds associated with a concept. Although languages may occasionally indulge in onomatopoeia, giving us the “boom” of an explosion or a cat’s “meow,” most words have a pretty arbitrary relationship with the concepts they represent, according to evolutionary linguist Kenny Smith (University of Edinburgh) in a 2022 Current Directions in Psychological Science article.
How then do we learn to call that thorny, soft-petaled flower a “rose” in English, and not some other random set of phonemes?
“Human languages persist through the cycle of learning and use: You learn a language through immersion in the language used in your linguistic community, and in using language to meet your communicative goals and get stuff done in the world, you produce further linguistic data, which other people in turn might learn from,” Smith explained.
This co-creative process allows languages to change and even split off from one another over time, and yet typically developing children become fluent in a continuously evolving spoken language with very little explicit instruction.
The cognitive revolution and linguistics
Classic theories of learning, including Pavlovian conditioning and Skinnerian behaviorism, focused on the relationship between stimuli and a person’s or animal’s outward responses without concern for how the learning is represented internally, explained Michael C. Frank, director of the Symbolic Systems Program at Stanford University, in an interview with the Observer.
By the 1940s, philosophical approaches to studying the mind—epitomized by Freudian psychoanalysis—had been supplanted by an empirical, experimental approach in the United States, according to George A. Miller (Princeton University) in a 2003 TRENDS in Cognitive Sciences review. This shift allowed psychology to evolve into an “objective science based on scientific laws of behavior,” Miller continued, but it also caused many researchers in the United States to distance themselves from the study of mental processes like perception and memory, which continued in other countries, including the United Kingdom, Switzerland, and Russia.
“The cognitive counter-revolution in psychology brought the mind back into experimental psychology,” Miller wrote, and with it the new promise of an interdisciplinary science that could account for both the representational and computational functions of the human brain. Central to this revolution in the 1950s were linguists like Noam Chomsky (The University of Arizona), who asserted that all languages emerge from an intuitive sense of “universal grammar” that sets certain cognitive constraints on what human language should sound like, enabling children to rapidly acquire their native tongue while also understanding language mathematically.
Check out this roundup of new research on language acquisition, bilingualism, and speech perception.
Today, Frank explained, the broad consensus in the field is that children learn languages by creating internal representations that are linked to one another in complex associative networks. This applies not only to more concrete concepts like “dog” or “cookie” but also to words with more abstract meanings like “no” and “same,” which can take longer to learn, he explained.
Accumulator models position these representations as being learned through cumulative language exposure, Frank continued. Each exposure to a specific word, whether directed at the child or simply overheard by them, is often depicted as a drop of water falling into a bucket.
“Every time a child hears that word, that’s another drop in the bucket. And when the bucket fills up, the child has learned that word,” Frank said. Tracking how drops accumulate in those buckets, and how accumulation may differ between families because of socioeconomic status and other factors, can help researchers model how children acquire language.
Frank and colleagues George Kachergis and Virginia A. Marchman (Stanford University) elaborated on this learning process in a 2021 Current Directions in Psychological Science article. Very young children may appear to learn language slowly at first because they have received enough word exposure to fill only a few shallow buckets, but these exposures continue to accumulate until the deeper buckets of more difficult words—such as more complex verbs like “have”—are ready to overflow, resulting in an “explosion” of vocabulary in many toddlers around age two.
Accumulating computational models
Beyond these broad similarities, one way that accumulator models of language learning may differ from one another is the extent to which they distinguish between words that are spoken directly to a child and overheard speech, Frank noted. Whereas some models may position all speech as being of roughly equal value, others consider speaking directly to a child to result in a much larger drop in the bucket, allowing children to learn a larger vocabulary more quickly when the adults in their lives make them part of the conversation.
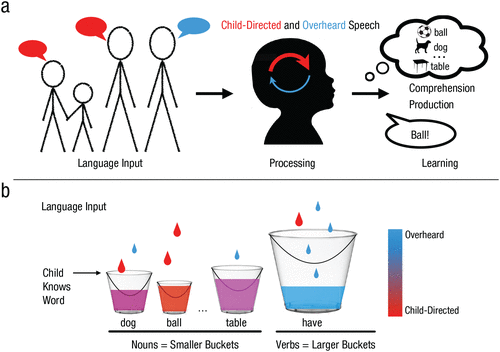
Conceptual conflicts like this are where mathematical modeling comes into the picture.
“In reading this literature, we found that everyone had some of the same basic ideas in mind, but they were talking past one another because there wasn’t a framework for describing different proposals in the same vocabulary,” Frank said. “Computational models can help formalize and consolidate proposals so they can be compared and tested.”
Language learning is uniquely suited to computational modeling, according to Frank and colleagues. That’s because it’s relatively easy for researchers to use techniques like environmental monitoring to get an objective measure of children’s language exposure, unlike more subjective psychological phenomena such as personality, which are generally studied using survey data. Knowing exactly how many and what kinds of words children are exposed to on a daily basis can help psychological scientists create a “standard model” of how these and other variables contribute to language learning, the researchers wrote.
“Early vocabulary sets the stage for reading success when children start school, so there has been a lot of interest recently in understanding the role of language input in children’s learning,” he said. “This area has been very controversial, with the ‘word gap’ narrative being described as stigmatizing, but it’s also an area where we could potentially help children thrive with the right interventions.”
Fighting for phonics
Another roiling controversy in the world of language learning is the question of how to most effectively teach children to read. In this case, researchers strongly advocate for the use of phonics, which involves explicitly instructing students on the sounds each letter makes before tackling entire words, sentences, and texts, whereas many educators continue to implement a “whole-language” approach to reading in the classroom, wrote Anne Castles (Macquarie University), Kathleen Rastle (University of London), and Kate Nation (University of Oxford) in a 2018 Psychological Science in the Public Interest article.
“The quality and scope of the scientific evidence today means that the reading wars should be over. But strong debate and resistance to using methods based on scientific evidence persists,” they wrote.
The whole-language approach, described by proponent Kenneth Goodman (University of Arizona) as a “psycholinguistic guessing game,” encourages students to infer the meaning of words from contextual clues, Castles and colleagues explained. This approach may make intuitive sense to adults who have been literate for decades, but just because reading entire newspapers and novels can seem effortless to experienced readers doesn’t mean that children are equipped to jump into the linguistic deep end.
“Such a conclusion is analogous to observing skilled concert pianists and concluding that piano instruction should involve putting a child in front of a Tchaikovsky score. The missing piece of the puzzle here is how these processes develop in children,” Castles and colleagues explained.
“Such a conclusion is analogous to observing skilled concert pianists and concluding that piano instruction should involve putting a child in front of a Tchaikovsky score. The missing piece of the puzzle here is how these processes develop in children.”
Castles, Rastle, and Nation
Unlike phonics, the whole-language approach leads children to merely memorize individual words instead of teaching them to “crack the alphabetic code” necessary to read words they’ve never seen before—an essential component of literacy, Castles and colleagues wrote. By way of example, Castles offered a series of studies by psycholinguist Brian Byrne (University of New England) and colleagues. They found that preschool-age children taught to sight-read the words “fat” and “bat” were not able to apply that knowledge to distinguishing between the words “fun” and “bun” in a text unless experimenters explicitly taught them the letters “f” and “b” in addition to the complete written words.
Numerous meta-analyses have demonstrated the value of using phonics to teach reading in classroom settings, the researchers noted. In the 1990s, a meta-analysis of 38 studies conducted by the National Reading Panel, a government body formed in 1997 at the request of the U.S. Congress, found that students’ pronunciation, spelling, and reading comprehension abilities were all significantly improved by phonics instruction.
More recently, Castles and colleagues added, the U.K. Department for Education reported that mandatory phonics instruction in England increased the percentage of 5- and 6-year-old students who passed phonics screenings from 58% in 2012 to 81% in 2017. Similarly, in a 2016 study of students from more than 1,000 schools, Stephen Machin (London School of Economics and Political Science) and colleagues found that students in the general population who received this instruction demonstrated improved reading comprehension up to age 7, and students who spoke English as a second language or came from lower-income families did so up to age 11.
“These results are consistent with the view that explicit teaching of phonics assists all children to access text material relatively early in reading instruction and that this explicit instruction is particularly vital for some children,” Castles and colleagues wrote.
Much of the disconnect between the scientific consensus and classroom practices comes down to issues with how the science of reading has been communicated to the public, the researchers noted. Although there is a deep well of research demonstrating the utility of phonics, those findings have rarely been presented in a lay-friendly manner that makes it clear why phonics works and how this learning strategy supports literacy.
“Reading scientists, teachers, and the public know that reading involves more than alphabetic skills,” Castles and colleagues explained. “We believe that the relative absence of discussion of processes beyond phonics has contributed to ongoing resistance to the use of phonics in the initial stages of learning to read.”
Phonics isn’t intended to be the final destination for children learning to read, the researchers wrote. Instead, it provides a sound-to-symbol foundation upon which students can progress to higher-level word recognition and comprehension of entire texts.
In addition to observing children’s actual reading behavior in experimental settings, as in the study by Byrne and colleagues described above, psychological scientists are also using computational modeling to analyze how different theories of reading compare to each other and to data from participants. These computer programs are coded to recognize letter strings using different “transformations” that represent cognitive processes that support reading, including a mix of whole-word recall and phonetic pronunciation.
“All of the models converge in that they represent two key cognitive processes in word reading: one that involves the translation of a word’s spelling into its sound and then to meaning, and one that involves gaining access to meaning directly from the spelling, without the requirement to do so via phonology,” Castles and colleagues wrote.
A child becomes a fluent reader when they are able to rely primarily on direct meaning, the researchers continued, though even adults rely on phonetic pronunciation when they encounter a new or unfamiliar word.
Digging into dyslexia
Learning these reading skills can be especially difficult for children with dyslexia, a developmental disorder that interferes with the ability to recognize letters and words, present in up to 10% of the general population, wrote Johannes C. Ziegler (Aix-Marseille University), Conrad Perry (Swinburne University of Technology), and Marco Zorzi (University of Padova) in a 2020 Current Directions in Psychological Science article. Fortunately, Ziegler and colleagues’ work suggests that computational modeling can be used not only to investigate how dyslexia impairs reading, but also to identify which interventions are likely to be most effective for each student.
“Children come to the task of learning to read with large interindividual differences in vocabulary, phonology, and orthographic skills,” the researchers wrote. “We believe that personalized computational modeling will play an important role not only in the early detection of dyslexia but also in the context of evidence-based interventions.”
Similar to the models described above, the phonological-decoding self-teaching theory of reading suggests that children begin learning to read an alphabetic language by being explicitly taught to map sounds onto letters, at which point they are ready to start teaching themselves words more independently, Ziegler and colleagues wrote. However, new readers may still require assistance learning words that don’t follow the standard rules of pronunciation, the researchers added. For example, in English, about 20% of words—such as yacht and choir—have irregular pronunciations that readers may need to be taught explicitly.
Ziegler and colleagues’ computational model represents this process as consisting of a reader’s understanding of letters and phonemes, which are fed through a decoding network as the new reader develops the phonological (pronunciation) and orthographic (spelling) lexicons required to become a fluent reader.
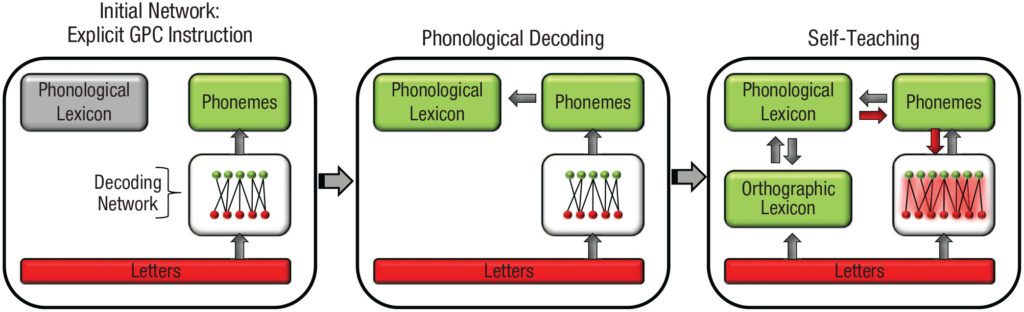
In a 2019 study, the researchers used these factors to predict how 388 children with dyslexia would perform on a series of reading-aloud tasks. Ziegler and colleagues input data on each child’s performance on pronunciation, spelling, and vocabulary tasks. Using this input, the custom computational models identified the correct pronunciation of a word set with a similar level of accuracy as the child.
Whereas previous models of dyslexia have suggested reading is impaired by a deficit in either pronunciation or spelling, Ziegler and colleagues found a multideficit model to be much more predictive of children’s actual reading behavior.
“Importantly, although the human data are only correlational, the relation between deficits and outcomes in the model is a causal one and can be used to derive empirical predictions,” Ziegler and colleagues added. “Such personalized models can be used to explore how changing the efficiency of one component through intervention is likely to change reading performance for an individual child.”
Language, whether spoken or read, is a tool that is constantly changing and growing, but the work of Ziegler and other researchers demonstrates that it may still be possible to pin down how it functions at its core. Decades from now, the flower we call a rose could go by another name entirely, but psychological scientists will know more than ever about the processes involved in how we learn to say it.
Relevant Research
-
Counting Ability May Emerge From the “Cognitive Technology” of Number Words
Humans’ ability to count may be limited by our knowledge of number words, according to a study of an isolated indigenous group in the Bolivian Amazon.
-
Literacy
Psychology researchers are identifying how we build strong reading skills in early childhood and the factors that contribute to difficulty with reading comprehension.
-
Studying and Learning
Psychological scientists delve into study strategies, math anxiety, reading comprehension, and more.
Feedback on this article? Email [email protected] or log in to comment.
References
Carroll, P., & Traylor, J. (2020). The history of psychology—The cognitive revolution and multicultural psychology [MOOC chapter]. Introduction to Psychology. Lumen Learning. https://courses.lumenlearning.com/waymaker-psychology/chapter/reading-the-cognitive-revolution-and-multicultural-psychology
Castles, A., Rastle, K., & Nation, K. (2018). Ending the reading wars: Reading acquisition from novice to expert. Psychological Science in the Public Interest, 19(1), 5–51. https://doi.org/10.1177/1529100618772271
Decision Lab. (n.d.) The Whorf hypothesis. https://thedecisionlab.com/reference-guide/linguistics/the-whorf-hypothesis
Kachergis, G., Marchman, V. A., & Frank, M. C. (2021). Toward a “standard model” of early language learning. Current Directions in Psychological Science, 31(1), 20–27. https://doi.org/10.1177/09637214211057836
Miller, G. A. (2003). The cognitive revolution: A historical perspective. Trends in Cognitive Science, 7(3), 141–144. https://doi.org/10.1016/S1364-6613(03)00029-9
Smith, K. (2022). How language learning and language use create linguistic structure. Current Directions in Psychological Science, 31(2), 177–186. https://doi.org/10.1177/09637214211068127
Ziegler, J. C., Perry, C., & Zorzi, M. (2020). Learning to read and dyslexia: From theory to intervention through personalized computational models. Current Directions in Psychological Science, 29(3), 293–300. https://doi.org/10.1177/0963721420915873
APS regularly opens certain online articles for discussion on our website. Effective February 2021, you must be a logged-in APS member to post comments. By posting a comment, you agree to our Community Guidelines and the display of your profile information, including your name and affiliation. Any opinions, findings, conclusions, or recommendations present in article comments are those of the writers and do not necessarily reflect the views of APS or the article’s author. For more information, please see our Community Guidelines.
Please login with your APS account to comment.