Bringing Precision Treatment to Mental Health Care
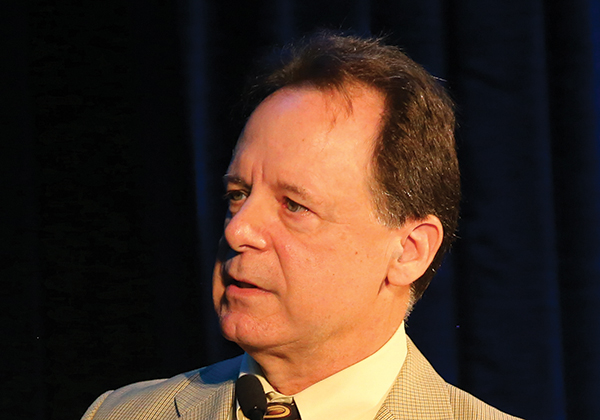
Ours is the era of customization — the idea that goods and services should be tailored to individual needs is woven into our daily lives. The most competitive employers now emphasize job crafting and flexible work arrangements to recruit top talent and ensure a good fit. And medical practitioners are paying increasing attention to individual differences, down to the genetic level, to maximize treatment benefits.
Only now are researchers discovering how much of an impact this individual-differences approach could have in treating mental health. APS James McKeen Cattell Fellow Robert J. DeRubeis (University of Pennsylvania) detailed his cutting-edge investigations into the factors that affect mental health treatment outcomes in his award address at the 2017 APS Annual Convention in Boston.
Across several decades, DeRubeis and colleagues have carefully unpacked several assumptions that have long prevailed in the clinical world. To do this, they start by asking a simple question: If this assumption is actually true, how would we know? This methodical approach has led them to some critical discoveries, including the idea that a steady trajectory of symptom improvement doesn’t necessarily lead to the best outcomes.
Examining patients who received cognitive therapy to treat depression, DeRubeis and then-graduate student Tony Tang found that some patients remained fairly stable across several treatment sessions and then showed sudden and noticeable improvement — a phenomenon the researchers called “sudden gain.” When they listened to audio recordings from the treatment sessions, they found that patients began talking about how they were reconsidering things and taking a different perspective in the sessions right before this sudden gain.
Although patients may have relatively similar scores on the Beck Depression Inventory at the end of treatment, additional findings revealed that those who showed a sudden gain were more likely to sustain those gains and even show further improvement compared with those who followed a steadier trajectory.
Another study showed that the severity of a patient’s symptoms also plays a role in whether a treatment is effective. Comparing medications with placebo, DeRubeis and coauthors found that medications worked well for patients who had severe symptoms, in line with previous research. But medications and placebo appeared to be similarly effective for the patients who are most likely to show up at a clinic seeking treatment: those with moderate symptoms.
The findings, published in JAMA in 2010, upended the popular notion that medications are necessarily beneficial in treating depression. The paper garnered considerable press, and DeRubeis even received threatening emails from doctors and patients who believed the findings should not have been published.
Despite this, the researcher has continued to push the boundaries of clinical science.
Clinical research has historically been focused on main effects, either of a treatment or a patient group, investigating which treatment is most effective or which patient group will fare best. Often, these main-effects studies indicate that two treatments produce very small differences in outcomes or that specific therapy mechanisms appear to have a tiny effect.
These findings could be due to problems with treatment delivery or outcome measurement — or it could be that our intuitions about the processes involved are just wrong. But an equally likely explanation, DeRubeis said, is that our intuitions don’t account for variation between patients.
Data show that, in a given population, some patients spontaneously remit and others have symptoms that seem intractable. The type of treatment these patients receive will matter little if at all — strong and weak treatments will be similarly effective or similarly ineffective.
Between these two extremes lie so-called “easy patients,” “pliant patients,” and “challenging patients.” The easy patients inevitably will show some improvement and will reach maximum improvement if they receive a minimal treatment. Pliant patients, on the other hand, mirror their treatment: They show no improvement if left untreated, moderate improvement with weak treatments, and maximum improvement with strong treatments.
Recognizing these different response patterns is essential in drawing conclusions about treatment effectiveness. Using computer simulations, DeRubeis and colleagues have shown that the distribution of these types of patients in a study comparing two treatments makes a huge difference in the study’s results.
Imagine, for example, that a hypothetical strong treatment produced a 55-point change on some outcome measure, on average, and a hypothetical weak treatment produced an average change of 45 points. If you were to compare the two treatments, with 60 patients in each group, you would expect a standardized mean difference of 10 points and a pooled standard deviation of 15 — a study with these basic features would yield a sizeable effect of d=.67.
But when DeRubeis and colleagues simulated a total of 1,000 studies, using different patient distributions, the results varied wildly.
In a study with patients who are all pliant, for example, the difference in outcomes between a strong treatment and a weak treatment remained clear, producing an effect size of d=.67.
Clinical practices will never have a pool of all-pliant patients, however. When the researchers shifted the distribution so that it contained predominantly pliant patients with some other patients mixed in, they saw the effect size decrease to d=.48. With a more skewed sample, the effect size dropped further, to d=.24.
“With .24, you probably don’t get that study published,” DeRubeis noted. “First of all, because it’s not going to be significant. And you’re going to look and say, ‘Well, those treatments are pretty much the same — .24, who cares?’”
Another study with actual patient data showed that these findings aren’t merely hypothetical. In collaboration with Dutch researchers Annemieke van Straten (VU Amsterdam) and Bea Tiemens (Radboud University), DeRubeis and then-graduate student Lorenzo Lorenzo-Luaces examined data from a randomized-control trial of depression treatments that was conducted in community settings.
At the aggregate level, the treatments appeared to be similarly effective.
The team then constructed a multivariable model in which they quantified different response patterns as a dimension that incorporated individual-level factors such as employment status, recurrence of major depression, and sleep quality.
As they predicted, the interaction between response type and treatment type was significant. For those patients who seemed relatively well-off on the response-type dimension, the mode of treatment didn’t seem to matter. But for those who were faring the worst on that dimension, cognitive behavior therapy was noticeably more effective than the other treatments.
This shows, in pretty unequivocal terms, that “it really does matter who the patients are, not just what the treatments are,” said DeRubeis.
In general, this actuarial approach entails examining a dataset, identifying multiple potential moderators, and using those moderators to generate and test predictive models. Building on previous work, DeRubeis and colleagues have developed a “personalized advantage index,” or PAI, which quantifies different interactions between treatment options and patient characteristics. The researchers take care to avoid overfitting these models, and they aim to include only those moderators that make sense from a clinical perspective.
With the PAI, the researchers can identify patients who are predicted to show very different outcomes on different treatments. It also can identify those who are predicted to show fairly similar outcomes across different treatments. Examining data from a study whose main findings were published in 2016, DeRubeis and frequent collaborator APS Fellow Steven D. Hollon (Vanderbilt University) found that the PAI approach can generalize across patient samples.
In this study, the researchers wanted to identify those patients who would benefit most from adding cognitive therapy to their existing medication-based treatment plan.
“Most guidelines say that the combination treatment is really needed for patients with severe symptoms,” DeRubeis said. “We were interested in whether we could do better than that with a multivariable profile.”
DeRubeis, Hollon, and colleagues developed a model using data from patients in Nashville and Chicago. Included in the model were factors that generally reflect the difficulty of a case, such as number of prior episodes, early onset, and loss of interest. The question was: Would the model work for a separate group of patients in Philadelphia?
Some patients had low scores on the Hamilton Depression Inventory (HDI) and were also identified by the model as needing less-intensive treatment. The study results showed that these patients improved without adding cognitive therapy to their treatment plan. Other patients had high HDI scores and were identified as faring poorly — and these people showed noticeable improvement when they started receiving cognitive therapy.
But the most interesting outcome occurred when a patient’s depression score and the model prediction indicated different treatment approaches.
The data revealed that patients who had a severe HDI score but were predicted to improve by the model showed no benefit from the additional cognitive therapy. And patients who had a lower HDI score but were predicted to fare poorly showed a huge benefit from combining cognitive therapy with medication.
These data show “the promise of putting information together,” DeRubeis said. “We’re finding that it’s not just trivial. There are these differences that would be helpful in directing patients to the better treatment.”
This is especially important given that some treatment options are much more intensive or more costly than others. Knowing that a more accessible approach — such as starting with lifestyle changes or e-therapy — can be effective for some patients can help clinicians in devising appropriate treatment plans.
DeRubeis continues to expand the scope of this work, reaching out to and collaborating with researchers around the world. And he hopes to put these actuarial models to the most rigorous test by conducting prospective studies.
Previous work “gives us confidence that these things aren’t just going to wash away when we transport them,” he said. “But the real test — and the one that’s going to make it so that health systems might even think about implementing these kinds of things — is the one where we would test these things prospectively and preferably in a site other than the ones where they’ve been developed.”