Cover Story
Do We Need More Methods?
Let’s be honest: Methods and statistics are not the average student’s favorite aspects of psychological science. Many graduate and undergraduate students seem to hold the viewpoint that courses in methods and statistics are a necessary evil, a rite of passage needed to obtain an MA or a PhD. As a researcher and teacher in the field of methods and statistics ,I wish this would be different, but the fact is that most psychology students are much more interested in what goes on in the brain, in therapy, or in relationships, than in mastering the tools needed to actually figure out what is going on.
These students may be surprised to learn that there are many more methods than the ones they are being taught, and that new methods are being developed every day. These developments pertain to either the measurement phase of research or the data-analytic phase of research. Innovations for the measurement phase — such as new methods for measuring or manipulating psychological constructs — are typically developed by substantive researchers as part of their specialization. Due to the nature of these innovations, their purpose and utility are often easily recognized, especially by potential users who come from the same field as the developer, such that there is relatively little discussion about the need for new methods (although the need for a particular new method may be debated). Data-analytic innovations, however, are typically developed by psychometricians (including applied statisticians and quantitative psychologists) who specialize in techniques for the analysis of psychological data. Because statistics and other data-analytic innovations are often highly technical, the need for these new methods may be much less apparent to the average scientist, often leading them to ask, do we really need more data-analytical methods?
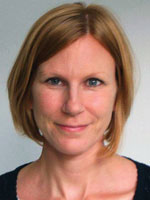
Ellen Hamaker
The short answer to this question is: “Yes, we do.” To elaborate, there are at least three reasons we continue to need new and different data-analytical methods in psychological science. First, while the value of traditional statistical methods, such as ANOVA and regression analysis, is beyond any doubt, these techniques are not appropriate for handling every interesting question that may arise in psychological science. In my own field of expertise, there is an ongoing debate regarding the value of between-person results when the interest is in within-person processes. For instance, if we want to know whether increases in stress lead to increases in negative affect at the process level (i.e., within an individual over time), how informative is it to know that people who reported more stress than did others are also reporting more negative affect? Although it has been shown time and again that the relationship between variables may differ across levels (Hamaker, 2012), the omission of cross-level generalizations is easily made and occurs all too often. Another example is the continuing debate between those who favor the frequentist approach to statistics (i.e., frequentists) and those who favor the Bayesian approach (i.e., Bayesians). Many Bayesian supporters claim that a Bayesian approach allows researchers to answer the actual questions we have (e.g., “Based on the observed data, can we conclude the manipulation had an effect?”), rather than questions that are related but far from identical to the actual questions (e.g., “Are these data, or more extreme data, likely to occur if the manipulation did not have an effect?”; see Wagenmakers, 2007). Due to recent technological developments, Bayesian alternatives are now being incorporated in mainstream software packages, such that more psychological researchers are confronted with this possibility. To be able to make an informed decision about whether or not to use these alternatives, researchers need to familiarize themselves at least to some extent with the arguments used by Bayesians and frequentists.
Second, when new methods of data collection are being developed, new forms of data arise that ultimately require new data analytical methods. An obvious example of this trend is the data that result from fMRI studies: The number of measurements — both in space and time — are huge and incomparable to the forms of data that psychological scientists encountered before. How to correctly handle such data has led to considerable debate (see, for instance, the many responses triggered by Vul, Harris, Winkielman & Pashler, 2009). Another example is the data obtained with experience sampling methods (ESM), which involve participants filling out questionnaires at random time points throughout the day to measure processes in real time. Such data are characterized by a relative high frequency, unequal intervals, sequential dependency, and circadian rhythms, and each of these characteristics may require specific attention when handling these data. A pragmatic approach to these new forms of data is to aggregate them over time and/or space so that they become more like our “traditional” data and allow us to use traditional methods. However, not only does aggregation lead to the elimination of a lot of valuable information, it also requires one to make decisions on how to aggregate. Suppose that a researcher’s interest is the individual differences in variability of affect in ESM data. An obvious measure to quantify variability would be the within-person variance, but one can also use mean squared successive differences (MSSD), which capture moment-to-moment variability (Jahng, Wood, & Trull, 2008). Clearly, these two summary measures represent different features of within-person variability, and it depends on the specific question at hand which measure is more appropriate.
Third, the development of new methods may also guide the formulation of new questions that we would not have been able to think of before. For instance, multilevel analysis was primarily developed to handle the dependencies in nested data. However, the fact that all kinds of effects may be random and may be related to each other or be predictable from other person/cluster characteristics has added an entirely new perspective to many research areas. One way in which this is currently being explored is in affect regulation research, in which it has been shown that the strength by which current affect depends on preceding affect (e.g., previous day, hour, or second) differs across individuals, and is related to individuals’ levels of neuroticism, depressiveness, and self-esteem (e.g., Kuppens, Allen, & Sheeber, 2010). This approach is providing exciting new insights in regulatory processes and maladaptive forms of coping.
If we acknowledge the continuing need for new data-analytical methods, the question is: How should a psychological scientist — who is already juggling teaching, management, and substantive research obligations — balance his or her resources between developing and exploring new data-analytical methods, and applying tried and established ones? Clearly, it would be unreasonable to expect a researcher to be an expert on his/her particular topic as well as to be aware of all the ins and outs of methods and statistics, including how to develop and evaluate new techniques.
The solution to this problem is to organize knowledge by investing in a solid and creative force of well-trained psychometricians who develop and evaluate new data-analytical methods and communicate their findings to potential users in an ongoing discourse. One way to contribute to this solution is by having a number of psychometricians within each psychology department, who not only teach methods and statistics but who are also engaged in innovative research. Having such a group in each department ensures that there are regular contributions to psychometric developments and allows students with an interest in and talent for methods and statistics to be trained and encouraged to pursue a career in this area.
Furthermore, both psychometricians and substantive psychological researchers should invest in a dialogue to bridge the gap between theory and practice. This really should be a mutual endeavor in which both parties bring their specific expertise to the table and develop a language to communicate about the subject matter. Ideally, psychometricians should be closely involved in all research lines that are conducted in a psychology department, and they should be involved at every stage (rather than just at the beginning to do some power calculations for a grant application or at the end to do some post-hoc consultation once all the data have been gathered). That way, psychological scientists — and psychological science — can benefit maximally from the unique and valuable expertise of psychometricians, and psychometricians will be well-informed on the specific problems that substantive researchers would like to see solved.
Finally — and this may sound a little patronizing — it is important for psychological scientists to regularly take courses and workshops on methods and statistics in order to keep their knowledge up to date and to familiarize themselves with new developments. (Note that the workshops offered by APS at the annual convention are an excellent way to get introduced to diverse specialized methods.) Clearly, one does not need to jump on every bandwagon that comes along, but when certain innovations have been around for a while and have proven their utility in a specific area, researchers should be given (and should take!) the opportunity to master them. Whether you love methods and statistics, or dread them, it is important to acknowledge that there are too many developments in this area to assume that the few courses taken to obtain one’s PhD will be enough for the rest of one’s scientific career. And besides, as I tell my students, methods and statistics are like olives: Most of us do not like them initially, but they can certainly grow on you.
Comments
Every contribution that challenges the terrible subordination of psychological research to the tyranny of Fisherian Null-hypothesis testing is to be applauded and supported, and this article is a very informative summary of new and useful developments.
Another useful article to consider is
Orlitzky, M. (2011). How can significance tests be deinstitutionalized? Organizational Research Methods. Published on line 12 December, 2011. DOI: 10.1177/1094428111428356.
APS regularly opens certain online articles for discussion on our website. Effective February 2021, you must be a logged-in APS member to post comments. By posting a comment, you agree to our Community Guidelines and the display of your profile information, including your name and affiliation. Any opinions, findings, conclusions, or recommendations present in article comments are those of the writers and do not necessarily reflect the views of APS or the article’s author. For more information, please see our Community Guidelines.
Please login with your APS account to comment.