New Research From Clinical Psychological Science
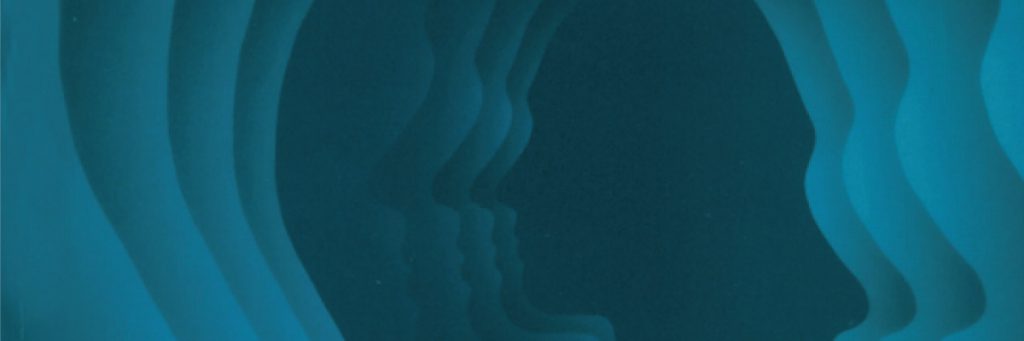
Antagonism in Daily Life: An Exploratory Ecological Momentary Assessment Study
Colin E. Vize, Whitney R. Ringwald, Elizabeth A. Edershile, and Aidan G. C. Wright
Vize and colleagues explored how antagonistic traits (e.g., manipulativeness, callousness, deceitfulness, grandiosity) manifest in daily life. In this exploratory study, participants completed measures of personality disorders, negative affectivity, detachment, disinhibition, and antagonism. Using ecological momentary assessment (EMA) for periods ranging from 7 to 21 days, participants also rated their positive and negative affect, empathy, loneliness, and warm/cold and dominant/submissive perceptions of the self and the other in daily social interactions. Results indicated heterogeneity within antagonism and robust relations of antagonism with some manifestations (e.g., negative affect) but not with others (e.g., empathy).
An Electrocortical Measure Associated With Metarepresentation Mediates the Relationship Between Autism Symptoms and Theory of Mind
Erin J. Libsack et al.
This study suggests that autism spectrum disorder (ASD) symptom severity and impairments in theory of mind (ToM; ability to make inferences about others’ state of mind) might be associated with distinct brain activity. Participants with and without ASD viewed vignettes and made mental-state inferences about the characters’ behavior while the researchers used electroencephalography to measure their brain activity. Participants with more accurate ToM and less severe ASD symptoms tended to show a late positive complex (LPC) event-related-potential. The LPC, thought to indicate cognitive metarepresentation, may help to explain the heterogeneity in ToM performance in individuals with ASD.
The Hitchhiker’s Guide to Computational Linguistics in Suicide Prevention
Yaakov Ophir, Refael Tikochinski, Anat Brunstein Klomek, and Roi Reichart
In this review, Ophir and colleagues provide a comprehensive outlook on the integration of computational linguistics (CL) in suicide prevention. The authors focus on deep neural network models and describe how CL methodologies (applied, for instance, to social-networking platforms) may contribute to early detection of suicide risk and hence prevent it. Research using CL may also deepen knowledge about suicide behaviors and promote personalized approaches to psychological assessment. Ophir and colleagues also discuss ethical and methodological concerns about the use of CL in suicide prevention, such as the difficulty of ensuring individuals’ privacy.
High Predictive Accuracy of Negative Schizotypy With Acoustic Measures
Alex S. Cohen et al.
Acoustic measures of one’s speech might effectively predict negative schizotypal traits, such as social anhedonia, lack of close friends, and constricted affect, this research suggests. Participants reported their schizotypal traits and provided speech data for analysis. Cohen and colleagues used machine learning to analyze the data and test a model linking the schizotypal traits with acoustic features. They found that the model’s predictions were more than 80% accurate, especially when speaking task and participants’ sex were considered. However, the features most predictive of traits were not the ones usually considered critical to the definition of schizotypal symptoms (e.g., poverty of speech).
A Hierarchical Taxonomy of Psychopathology (HiTOP) Primer for Mental Health Researchers
Christopher C. Conway, Miriam K. Forbes, Susan C. South, and the HiTOP Consortium
The Hierarchical Taxonomy of Psychopathology (HiTOP) deconstructs diagnostic categories and replaces them with dimensions. Rethinking mental health in terms of hierarchical dimensions with broad and specific components can help to explain individual differences in mental health as a matter of degree and why some conditions tend to co-occur. The HiTOP model can also be used to advance research on mental health. Conway and colleagues demonstrate how to use HiTOP to formulate and test research questions. They provide a series of tutorials and annotated code for each one, so researchers can use HiTOP to generate novel insights.
How the Discrepancy Between Prior Expectations and New Information Influences Expectation Updating in Depression—The Greater, the Better?
Tobias Kube, Lukas Kirchner, Gunnar Lemmer, and Julia Anna Glombiewski
In two studies involving false feedback on a performance task (analyzing other people’s emotions) and a social-interaction task (imagining going to a party and socially interacting with other people), Kube and colleagues varied the extent to which new information deviating from prior expectations led to updated expectations. They found that participants deemed new information most valid if it was moderately positive. Moreover, they updated their expectations the most when new information only moderately violated their previous expectations. The pattern of results defies traditional learning models that suggest that learning increases in accordance with the size of discrepancies between expectations and reality.
Sibling Alcohol Use Disorder Is Associated With Increased Risk for Suicide Attempt
Mallory E. Stephenson et al.
Stephenson and colleagues used a sample of Swedish siblings to examine the associations between having a sibling with alcohol use disorder (AUD) and elevated risk for attempting suicide. Results indicated that individuals who had a sibling with AUD were more likely to have elevated risk for attempting suicide, even when researchers controlled for the individuals’ own AUD. Moreover, exposure to a sibling with AUD appeared to be an environmental risk factor for attempting suicide, not solely a genetic risk factor. Because the risk was higher immediately following a sibling’s AUD diagnosis, clinical outreach may be an effective approach for reducing the likelihood of suicide attempts among individuals whose siblings have AUD.
APS regularly opens certain online articles for discussion on our website. Effective February 2021, you must be a logged-in APS member to post comments. By posting a comment, you agree to our Community Guidelines and the display of your profile information, including your name and affiliation. Any opinions, findings, conclusions, or recommendations present in article comments are those of the writers and do not necessarily reflect the views of APS or the article’s author. For more information, please see our Community Guidelines.
Please login with your APS account to comment.