Featured
Rage Against the Machines
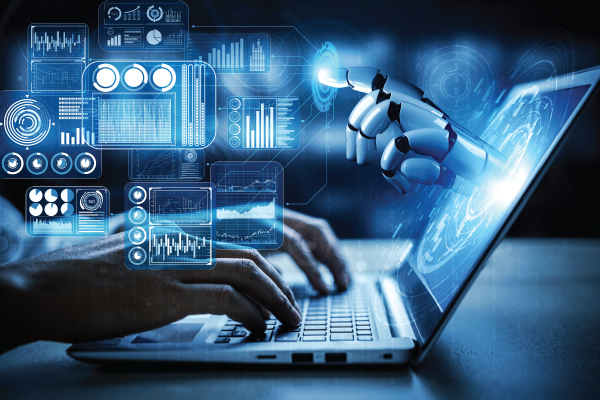
Since the dawn of the industrial revolution, laborers have battled the prospect of technology replacing them. The original “Luddites”—British weavers and textile workers—fought the advent of mechanized looms and knitting frames in the early 1800s. A century later, Belgian lamplighters smashed the electric streetlamps that were replacing the gaslights they fired up.
But in the 21st century, technology is penetrating the last vestige of the human work experience. The machines learn. They adapt. They not only handle blue-collar work but can mimic the skills of journalists, pharmacists, and surgeons.
People are indeed wary of artificial intelligence, and not just because they’ve been spooked by the murdering machines depicted in the Terminator movie franchise or 2001: A Space Odyssey. They view artificial intelligence as another threat to their jobs.
Learning Machines Can Learn Bias, Research Shows
Technology companies have been rolling out a bounty of machine learning tools to help employers eliminate human bias and prejudice from the hiring process. But do they work? Researchers are beginning to uncover evidence that computer algorithms are only as neutral as the people—mostly White men—who design them.
A team of computer scientists at Princeton University demonstrated this recently in an experiment rooted in the Implicit Association Test (IAT), a tool developed in the 1990s by APS Past President Mahzarin Banaji (Harvard University), APS William James Fellow Anthony Greenwald (University of Washington), and APS Fellow Brian Nosek (University of Virginia). In the IAT, participants categorize words or images that appear onscreen by pressing specific keys on a keyboard. Their response time to different combinations of stimuli is thought to shed light on the mental associations they make, even when they aren’t aware of them. The tool has led to the examination of unconscious and automatic thought processes among employers, police officers, jurors, voters, and people in many other contexts.
The research team used an artificial intelligence version of the IAT and set it loose on a wealth of web content, covering 840 billion words. Artificial agents examined sets of role-related words like “engineer” and “scientist” or “nurse” and “teacher” alongside gendered words such as “man” and “female.” The researchers found that the program associated female names more with words like “parent” and “wedding” compared with male names. Meanwhile, it associated male names with career words like “professional” and “salary.” It also manifested more negative associations with African American names than with European American names (Caliskan et al., 2017).
Evidence has suggested that computer algorithms exhibit racist or sexist tendencies based on patterns learned from public records and other humangenerated data. But a study by researchers at Cardiff University and Massachusetts
Institute of Technology psychological scientist David Rand revealed that learning machines could develop prejudicial groups all on their own.
The findings were based on computer simulations involving virtual agents. In a game of give-and-take, each agent decided whether or not to donate to somebody from their group or a different group.
As the game unfolded and a supercomputer racked up thousands of simulations, each actor began to learn new strategies by copying others—either members of their own group or the entire population (Whitaker et al., 2018). The findings showed that actors updated their prejudice levels by preferentially copying those that gained a higher short-term payoff.
The results demonstrate that prejudice transcends sophisticated human cognition and can manifest in “simple agents with limited intelligence,” the researchers wrote—a finding with “potential implications for future autonomous systems and human-machine interaction.”
References Caliskan, A., Bryson, J. J., & Narayanan, A. (2017). Semantics derived automatically from language corpora contain human-like bias. Science, 356(6334), 183–186. http://doi:10.1126/science.aal4230
Whitaker, R. M., Colombo, G. B., & Rand, D.G. (2018). Indirect reciprocity and the evolution of prejudicial groups. Scientific Reports, 8, Article 13247. https://doi.org/10.1038/s41598-018-31363-z
In 2017, 85% of Americans responding to a Pew Research survey said they favored policies that limited robots to performing hazardous duties. Other studies have validated those sentiments. In a 2020 study out of the Massachusetts Institute of Technology, two economists found that artificial intelligence is hitting the automobile, electronics, plastics, and chemical industries and metals manufacturers the most. And they found a direct link between automation and declining blue-collar income.
Psychological scientists Timo Gnambs from Johannes Kepler University Linz in Austria and Markus Appel of Julius Maximilian University of Würzburg in Germany recently explored the rising wariness many people, particularly blue-collar workers, feel toward artificial intelligence in the workplace.
For their study, Gnambs and Appel analyzed data from the Eurobarometer, a representative survey of more than 80,000 European residents. The data came from interviews conducted in 2012, 2014, and 2017. The researchers found attitudes toward artificial intelligence souring over the 5 years, with especially negative opinions about robots assisting at work. Gnambs and Appel also found that blue-collar workers were more likely than people with office jobs to harbor negative feelings toward artificial intelligence (Gnambs & Appel, 2019).
Blame the bot
As workers witness the emergence of autonomous robots in factories and offices, they start treating the machines like social actors, research indicates. That includes holding the robots accountable for mistakes, results of a 2019 study suggested. Researchers led by Douglas J. Gillan, a psychology professor at North Carolina State University, recruited 164 participants from Amazon’s Mechanical Turk and presented them with several hypothetical errors involving both a human and a robot. In one of the stories, a wobbling operating table jeopardized a heart procedure performed jointly by a surgeon and an autonomous robot. In another, the operator of a non-autonomous military robot made an error during a critical threat response. And in yet another, an autonomous robot misinterpreted an operational command at an auto parts warehouse, resulting in a delayed shipment.
When participants were told that the human controlled the robot, they blamed that individual for the accident. When told that the human was simply monitoring an autonomous robot, they placed most of the blame on the machine. In the surgery scenario involving both a human and an autonomous robot working in tandem, both shared the blame (Furlough et al., 2019).
The findings signal the complexities that artificial intelligence creates for workplace accountability.
“The study… raises questions about how quickly autonomous robots may be assimilated into the workplace,” Gillan said in a press release. “Do employers want to buy robots that may be more efficient, but can be blamed for errors—making it more difficult to hold human employees accountable? Or do employers want to stick to robots that are viewed solely as tools to be controlled by humans?”
Resistance to automation also correlates with antipathy toward immigrants, an empirical report published in Psychological Science suggests. Across 12 studies, Monica Gamez-Djokic and Adam Waytz, both of Northwestern University’s Kellogg School of Management, found that people who perceive automation as a threat to employment also tend to hold negative perceptions about immigrants. The researchers found support for that link across seven of the studies, involving data stretching from 1986 to 2017 across the United States and Europe. The link held over 3 decades, even after the researchers adjusted for political beliefs and perceptions of other employment-related threats, such as inflation and outsourcing.
Four of the other studies used correlational and experimental methods to examine automation’s influence on individuals’ perceptions of the group threat posed by immigrants and support for restrictive immigration policies. Two of those studies assessed 265 participants’ perceptions of immigrants by using both realistic-threat subscales (e.g., “Immigrants should be eligible for the same health care benefits received by Americans who cannot pay for their health care”) and symbolic-threat subscales (e.g., “The values and beliefs of immigrants regarding moral and religious issues are not compatible with the beliefs and values of most Americans”).
Finally, Gamez-Djokic and Waytz presented individual participants with one of two scenarios involving a company planning layoffs to cut costs. In the first, the company planned to restructure and downsize certain departments to reduce expenses. In the second, new technology was assuming many of the employees’ work duties. Participants faced with the second scenario decided to lay off a greater percentage of immigrants in the workforce (Gamez-Djokic & Waytz, 2020).
Indeed, people often vent their frustrations over automation onto other humans rather than the technology itself, recent research indicates. A team of business researchers, including psychological scientist Armin Granulo of the Technical University of Europe, conducted surveys with more than 2,000 people. Their sample encompassed students and laborers—including workers who had lost their jobs within the prior 2 years. They presented the participants with a variety of scenarios involving job losses to other people and to robots.
In the abstract, the idea of people’s jobs being taken over by robots and software was more palatable to the participants than the idea of jobs being taken by other workers (Granulo et al., 2019). Yet when faced with the prospect of their own jobs being cut, they preferred being replaced by a robot rather than a human.
In explaining the paradoxical results, Granulo and colleagues noted that people measure themselves against other people, not machines—so being displaced by automation packs less of a blow to their sense of self-worth. Participants indicated that threats to their self-worth would be reduced even if they were replaced by other employees who relied on technological abilities, such as artificial intelligence, in their work.

The role of education and personality
Psychological scientists also are learning the factors that help people avoid losing their jobs to technology. It comes down to personality traits, intelligence, and vocational interests, as a study led by personality psychologist Rodica Damian of the University of Houston showed.
Using longitudinal data from the American Institutes of Research, Damian and colleagues measured the social background, IQ, personality traits, and vocational interests of 346,660 high school students. They looked at follow-up data for those individuals from 11 and 50 years later, recording their occupations and coding the probability of those jobs becoming automated.
Their analysis showed that the students who were more intelligent, mature, and interested in arts and sciences were less likely to lose a job to automation years later, regardless of their socioeconomic background (Damian et al., 2017).
“On average, a one standard deviation increase in each of these traits predicted an average of 4 percentage points drop in the probability of one’s job of being computerized,” they reported. “At the U.S. population level, this is equivalent with saving 5.8 million people from losing their future careers to computerization.”
The findings signal that traditional education may fall short of addressing upcoming changes in the labor market, Damian wrote. While policymakers talk of the need to make college accessible for more people, machine learning is spreading so fast that a university degree may not be enough to secure a job, she noted. The education system may also need to nurture social skills to help future adults thrive in their vocations.
“The edge,” she said, “is in unique human skills.”
Scott Sleek is a freelance writer in Silver Spring, Maryland, and the former Director of News and Information at APS.
Feedback on this article? Email [email protected] or scroll down to comment.
References
Damian, R., Spengler, M., & Roberts, B.W. (2017). Whose job will be taken over by a computer? The role of personality in predicting job computerizability over the lifespan. European Journal of Personality, 31(3): 291–310. https://doi.org/10.1002/per.2103
Furlough, C., Stokes, T., & Gillan, D.J. (2019). Attributing blame to robots: I. The influence of robot autonomy. Human Factors. Advance online publication. https://doi.org/10.1177/0018720819880641
Gamez-Djokic, M., & Waytz, A. (2020). Concerns about automation and negative sentiment toward immigration. Psychological Science, 31(8), 987–1000. https://doi.org/10.1177/0956797620929977
Gnambs, T., Appel, M. (2019). Are robots becoming unpopular? Changes in attitudes towards autonomous robotic systems in Europe. Computers in Human Behavior, 93: 53-61. https://doi.org/10.1016/j.chb.2018.11.045
Granulo, A., Fuchs, C., & Puntoni, S. (2019). Psychological reactions to human versus robotic job replacement. Nature Human Behaviour, 3, 1062–1069. https://doi.org/10.1038/s41562-019-0670-y
Smith, A., & Anderson, M. (2017). Automation in everyday life. Pew Research Center. pewresearch.org/internet/2017/10/04/automation-in-everyday-life/
APS regularly opens certain online articles for discussion on our website. Effective February 2021, you must be a logged-in APS member to post comments. By posting a comment, you agree to our Community Guidelines and the display of your profile information, including your name and affiliation. Any opinions, findings, conclusions, or recommendations present in article comments are those of the writers and do not necessarily reflect the views of APS or the article’s author. For more information, please see our Community Guidelines.
Please login with your APS account to comment.