If Neuroscience Needs Behavior, What Does Behavioral Science Need?
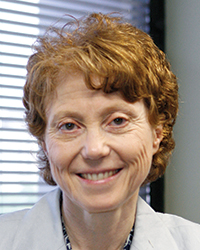
“Neuroscience needs behavior.”
That’s the remarkably direct title of a recent article in Neuron by Krakauer, Ghazanfar, Gomez-Marin, MacIver, and Poeppel. For most psychological scientists, the article’s message probably seems uncontroversial and obviously true. But the journal’s primary audience, the neuroscience community, instantly began to debate the Krakauer et al. perspective in various venues, such as on Twitter and in journal clubs.
This disciplinary disconnect has important implications for the pace of discovery and reliability of science, and for that reason alone we should work to overcome it. But this disconnect also plays out in the institutions and policies that govern federal funding for psychological science, with the unfortunate result that psychological science is often not adequately recognized and supported. For example, the Brain Research through Advancing Innovative Neurotechnologies (BRAIN) Initiative is overwhelmingly focused on advances in technology and innovation at the level of the genome, the connectome, and molecular and cellular neural mechanisms. In the same vein, the National Institute of Mental Health (NIMH) Strategic Plan continues to emphasize understanding mental health at the level of cellular and circuit analyses. And there are other examples as well.
Krakauer et al. provide great arguments for the role of psychological science in these kinds of initiatives (for the sake of space, I won’t go into the detailed arguments that are presented in the article, but I encourage you to read it). But we need to take the discussion a step further and identify what’s missing from large-scale federal initiatives that focus on neuroscience to the exclusion of behavior.
In this column, I discuss five examples of the kind of potentially high-payoff areas involving questions that are in the domain of both neuroscience and psychological science but illustrate clear needs that would correct some of the current imbalance in federal support. The first three are examples of the kinds of major infrastructure projects needed in the behavioral sciences, while the fourth and fifth address the behavioral science–neuroscience relationship more directly.
Big Questions, Big Data
In an era of Big Data, it will come as no surprise that one of my nominees for investment is collecting and using appropriate Big Data sets. Given that I am a developmentalist, it’s probably also no surprise that I would advocate for longitudinal studies. But I’m not talking about just any large-sample longitudinal project where the default assumption is “The bigger the better.” Data-collection projects, large or small, need to be targeted to key outcome variables, with samples and measures selected to illuminate a particular issue. In short, data collection is most useful when it tests a hypothesis.
One example of a fruitful effort that is now complete is the Eunice Kennedy Shriver National Institute of Child Health and Human Development’s (NICHD) Study of Early Childcare and Youth Development, which definitively answered a central question, showing that there is little need to worry about nonmaternal care if that concern is based simply on the fact that it is nonmaternal. A second example of an investment with ample returns is a much smaller study, the longitudinal study of language development in typically developing children and children with brain damage, led by APS Immediate Past President Susan Goldin-Meadow at the University of Chicago. This study yielded rich information on the role of parental speech and gesture in development of both kinds of children, leading to recommendations regarding new diagnostic tools and interventions for children at risk. A currently ongoing example is the National Institute on Drug Abuse’s (NIDA) Longitudinal Study of Adolescent Brain and Cognitive Development, which holds immense promise to elucidate the developmental pathways leading to substance-use disorders.
Many more such studies are possible to imagine, including those on topics of interest to NIMH, such as suicide, one of the institute’s current priority areas. Without clear hypotheses, however, payoffs are reduced, as we saw in the case of the proposed National Children’s Study, which suffered from a very diffuse focus and from trying to do everything at once, thus doing nothing well. Nearly all large longitudinal data sets end up having secondary uses once they are made public, but they benefit immensely from initial design with clear goals. Big Data can answer Big Questions, but it requires carefully formulated hypotheses from the outset.
A National Behavioral Data System
In an era of open science, a second obvious nominee for what psychological science needs is data sharing. There are compelling examples of how data sharing supports progress in understanding behavior; I’ll just cite two efforts in developmental
psychology. The field of child language has benefited immeasurably from the Child Language Data Exchange System (CHILDES), spearheaded by Brian MacWhinney at Carnegie Mellon University and now part of a wider data set called TalkBank. More recently, Karen Adolph of New York University and Rick Gilmore at Pennsylvania State University have led the effort to create Databrary, a resource that provides the means for sharing video data sets easily, along with free analytic tools.
Such efforts allow researchers to conduct analyses relevant to understanding disorders such as language delay (CHILDES) or to analyze the parenting of depressed mothers and fathers (Databrary). But both CHILDES and Databrary, and other projects like them, are possible only when stable funding is provided. We urgently need a federal effort to bring the best-curated and most-used data sets in behavioral science into some sort of national “data library” system.
Open Toolboxes
My third nominee for a Big Ask in the study of behavior is for much more work on developing well-standardized and freely available tools for evaluating individual differences. The National Institutes of Health (NIH) Toolbox was an excellent step in this direction, but it’s far from comprehensive. One tool it lacks is resources for assessing spatial skill, my own favorite domain. Apparently, spatial thinking just missed the cutoff in a popularity vote concerning which tools to develop for the toolbox! New funding is needed to expand the toolbox so that it truly covers the full range of constructs we need to assess. We also need to address the fact that behavioral testing often requires materials and tools that go beyond paper-and-pencil questionnaires or easily reproducible props. For example, virtual reality environments are needed to quantify individual differences in navigation. Wandering is a prominent and troubling symptom in Alzheimer’s disease; indeed, difficulties in navigating can serve as one useful marker for elderly individuals at risk.
But sharing VR paradigms across labs requires standardization, curating, and a stable platform on which to access them.
Translating Across Species
Looking more directly at the linkage between neuroscience and behavior, my fourth example is support for translational cross-species research involving both areas. The majority of research in basic neuroscience involves animal models. But how do we know if the models translate across species? Paradigms that work with rats don’t always work with mice, at least not without adjustment, and translating work across species to include humans seems even more of a stretch. So, for example, is a technique that evaluates attention in mice a way to examine human attention? Or is it a measure of what a cognitive researcher would call vigilance?
In research on substance-use disorders, NIDA now prioritizes research in which animals come to self-administer a drug of abuse, rather than passively receiving it from experimenters. After all, humans don’t typically develop substance-use disorders because someone has been injecting them against their will. Similarly, in listening to talks on rodent models of addiction, I’ve been struck by the fact that animals differ among themselves in their acquisition curves and in how they react to parameters such as delays or varied delivery schedules. Taking these variations as a potential model of individual differences in humans, rather than as annoying error variance, seems to me a desirable goal. But it would be expensive — many more animals would need to be studied in order to get adequate statistical power. A focus on human behavior would underline the importance of pursuing this line of inquiry and could build on well-established individual and developmental differences in human behavior such as risk preferences, reward responsiveness, and impulsivity and inhibition that are known to be related to addiction. But the initial need is to establish the translatability of paradigms across species. Tackling this question will require major investment.
The Right Stuff
My final example concerns the Research Domain Criteria (RDoC) effort at NIMH. My own research does not concern psychopathology, but the effort to delineate types of psychopathology using behavioral, neuroscientific, and computational methods strikes me as exciting. However, these efforts can’t succeed if they use the wrong constructs or imperfect measures. When I look at the RDoC recommendations for methods to study behavior, I worry that the effort has not drawn on the best conceptualization of human cognition and social behavior.
Let’s just take the case of declarative memory, one area in which I work. The constructs targeted in this area by an RDoC working group are relational memory, associative inference, paired-associates learning, and pattern separation. It’s an interesting list, proposed by an expert panel, but not one on which the wider memory community would necessarily converge. There are a number of unsolved research questions in this domain. Consider just the issue of pattern separation, which has been operationalized as distinguishing between two perceptually and conceptually similar items, one of which was seen before and the other novel: It’s currently unclear whether pattern separation is the opposite of its hypothesized complement, pattern completion, or if pattern completion is a distinct process that would require separate assessment. Many other issues concerning pattern separation are unsettled, and the development of pattern separation is only beginning to be understood.
A Grand Challenge for RDoC is determining whether we even possess the right analytic concepts and the right methodological tools to make the effort pay off. And if not, as I suspect, we’ll need to invest in research dedicated to getting us where we want to be.
There are surely many more examples, but the point is that as psychological scientists we can’t wait to be invited to the party. We need to advocate for the infrastructure and other support needed to ensure that the connections between behavioral science and neuroscience are balanced and productive.
Reference
Krakauer, J. W., Ghazanfar, A. A., Gomez-Marin, A., MacIver, M. A., & Poeppel, D. (2017). Neuroscience needs behavior: Correcting a reductionist bias. Neuron, 93, 480–490.
APS regularly opens certain online articles for discussion on our website. Effective February 2021, you must be a logged-in APS member to post comments. By posting a comment, you agree to our Community Guidelines and the display of your profile information, including your name and affiliation. Any opinions, findings, conclusions, or recommendations present in article comments are those of the writers and do not necessarily reflect the views of APS or the article’s author. For more information, please see our Community Guidelines.
Please login with your APS account to comment.