The Curious Construct of Active Learning
Psychological Science in the Public Interest (Volume 22, Number 1)
Read the Full Text (PDF, HTML)
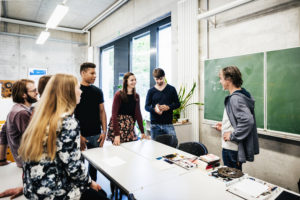
Active learning has become a ubiquitous construct in education—particularly undergraduate education in science, technology, engineering, and mathematics (STEM). However, despite its prevalence, active learning remains an unclear construct. Different researchers and educators may have different definitions of what active learning is and which activities foster active learning and improve learning outcomes.
In this issue of Psychological Science in the Public Interest (Volume 22, Issue 1), Doug Lombardi, Thomas F. Shipley, and teams of researchers in STEM synthesize findings on STEM learning to provide a clear and coherent conceptualization of active learning and offer guidance on research and practice. The authors come from the fields of cognitive and educational psychology and from discipline-based education research (DBER) in astronomy, biology, chemistry, engineering, geography, geoscience, and physics. This innovative multidisciplinary collaboration allowed the authors to examine active learning from the perspectives of psychology and DBER to build knowledge that does not currently exist in a single domain.
What is active learning?
Active learning is commonly used as an umbrella term to communicate an alternative to the traditional lecture in which students sit down, listen to the instructor, and take notes. Multiple activities have been described as active learning, including participation in flipped classrooms (in which students read or watch educational material outside of class and use class time to engage in interactive activities), use of clickers or other student response systems, and engagement with computerized conversational agents. The variety of learning methods categorized as active learning makes it difficult to know exactly what active learning is and how it might foster learning. Despite this lack of clarity, some studies have reported that, compared with traditional lectures, active learning appears to increase performance on tests and decrease failure rates (e.g., Freeman et al., 2014). Moreover, active-learning strategies appear to provide more equitable outcomes in STEM domains for students from underrepresented groups.
Many researchers have defined active learning “as a generalized process (a) for constructing knowledge and (b) for deepening engagement,” Lombardi and colleagues write. The conceptualization of active learning as antithetical to passive learning or lecture might overlook characteristics of lecture that promote learning, they state. For instance, people can learn by observing others or without being fully engaged in a learning task. Overall, the current view of active learning is underdeveloped, according to the researchers.
In this review, each of the DBER teams synthesizes active learning in its domain, acknowledging the difficulty in defining active learning within each domain. The authors then draw from each domain to construct a working definition of active learning: “Active learning is a classroom situation in which the instructor and instructional activities explicitly afford students agency for their learning.” Building on that definition, they present a framework that situates active learning in an ecosystem, capturing different definitions of active learning and the complex interactions in undergraduate STEM learning.
The construction-of-understanding ecosystem
In Lombardi and colleagues’ framework, an active-learning environment consists of students being agents in making sense of domain practices, data about phenomena, and scientific models together with their peers. Students also consider the science they have personally experienced, and teachers use their expert knowledge of science content (practices, data, and models) to facilitate students’ sense making. Finally, students self-reflect on their understanding. Lombardi and colleagues contrast this framework with the traditional approach to knowledge construction, in which the teacher has expert knowledge of scientific practices, data, and models, along with direct experiences, and transmits this information to the students, who passively receive it.

Figure 1. Illustration of an active-learning environment, according to the construction-of-understanding ecosystem.
This framework of active learning does not rely on a dichotomy between active and passive learning. Instead, it reflects “a continuum of more or fewer, richer or poorer, opportunities to construct meaning.”
How to employ active learning in STEM undergraduate research and practice
The construction-of-understanding ecosystem framework can provide coherence and utility for directing future research and practice. Lombardi and colleagues hope that the framework will lay the groundwork for theoretical developments and more rigorous research by “highlighting the variety of points in the system where learning could be influenced… and the potential for interactions among those influences over time.” The authors add that better measures of what students know and can do are needed to increase the understanding of the impact of active learning on students. A critical factor in assessing the effects of active learning is aligning the assessment with STEM learning objectives and the methods of instruction (e.g., constructed response assessments, in which students predict or explain phenomena). Moreover, assuming that active learning simply increases learning, as if learning were a single dimension, is an oversimplification that might not capture higher-order learning.
Nevertheless, it appears important to explicitly encourage students to be active agents in their learning, because doing so allows them to be more self-reflective and aware of what they understand and how that understanding was constructed. Not only can such an approach increase students’ scientific knowledge in a domain, but it also promotes the use of efficient strategies in new learning domains. Lombardi and colleagues add that “interpreting active-learning situations, in which meaning is constructed both cognitively and socially, offers an organizational framework for the science of learning, design-based research, and scholarship of teaching and learning to integrate theory and observations toward understanding learning across the life span.” Therefore, active-learning environments must be viewed as those in which knowledge-construction processes play a role for the learner and the science-learning community as well as for the scientist and the scientific community.
How Can We Make Active Learning Work in K–12 Education? Considering Prerequisites for a Successful Construction of Understanding
By Garvin Brod,
Leibniz Institute for Research and Information in Education (DIPF) and Department of Psychology, Goethe University, Germany
Prerequisites for active learning
In an accompanying commentary, Garvin Brod (Leibniz Institute for Research and Information in Education [DIPF] and Department of Psychology, Goethe University, Germany) expands Lombardi and colleagues’ construction-of-understanding ecosystem. Brod adds students’ beliefs about their own agency, prior knowledge, cognitive capacities, and metacognitive capacities as prerequisites for active learning to foster learning and understanding. The evidence for these prerequisites comes from research in developmental psychology, which has shown that, for instance, the benefit of giving students control over their learning emerges only around age 6 and continues to increase across the elementary-school years. By understanding how the prerequisites develop across childhood and adolescence, researchers and educators can tailor active-learning activities to younger students. For example, providing additional guidance and using tasks that do not require advanced analogical thinking (which develops only in secondary school) might facilitate active learning in elementary school.
See related news release.
*DISCIPLINE-BASED EDUCATION RESEARCH TEAMS
Astronomy Team Members
Janelle M. Bailey, Temple University
Paulo S. Bretones, Universidade Federal de São Carlos
Edward E. Prather, University of Arizona
Biology Team Members
Cissy J. Ballen, Auburn University
Jennifer K. Knight, University of Colorado, Boulder
Michelle K. Smith, Cornell University
Chemistry Team Members
Ryan L. Stowe, University of Wisconsin-Madison
Melanie M. Cooper, Michigan State University
Engineering Team Member
Michael Prince, Bucknell University
Geography Team Members
Kinnari Atit, University of California, Riverside
David H. Uttal, Northwestern University
Geoscience Team Members
Nicole D. LaDue, Northern Illinois University
Peggy M. McNeal, Towson University
Katherine Ryker, University of South Carolina
Kristen St. John, James Madison University
Kaatje J. van der Hoeven Kraft, Whatcom Community College
Physics Team Member
Jennifer L. Docktor, University of Wisconsin-LaCrosse
APS regularly opens certain online articles for discussion on our website. Effective February 2021, you must be a logged-in APS member to post comments. By posting a comment, you agree to our Community Guidelines and the display of your profile information, including your name and affiliation. Any opinions, findings, conclusions, or recommendations present in article comments are those of the writers and do not necessarily reflect the views of APS or the article’s author. For more information, please see our Community Guidelines.
Please login with your APS account to comment.