How Researchers Can Find Different Results Using the Same Data
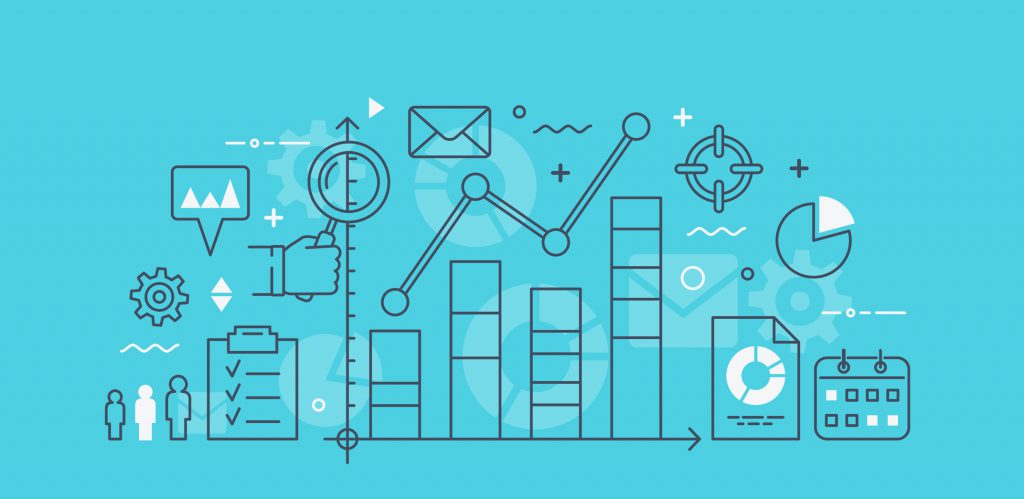
We might expect results to vary when research teams looking at the same question use different data sets, but how much variation is there when the data is the same? Quite a bit, according to a study published in Advances in Methods and Practices in Psychological Science. Research teams used one data set to answer one question: Are soccer referees more likely to give red cards to dark-skin-toned players than to light-skin-toned players? Their analyses produced varying effect sizes, and 20 teams found a statistically significant relationship while nine did not.
Data analysis may seem like a straightforward process that follows standard rules in a given order. However, psychological scientists are beginning to recognize that analysts have to make certain decisions, such as choosing how to test a given hypothesis and identifying which statistical assumptions are appropriate, that can fundamentally influence the results of a study.
For this study, the authors wanted to determine just how much variation in outcomes would emerge if independent teams analyzed the same data using the analytical strategy of their choosing.
The study included 29 teams from 13 different countries, with a total of 61 analysts representing various academic fields, degrees received, and professional status (i.e., associate professor, full professor, postdoc, doctoral student). Each team received identical data on soccer players in the first male division in England, Germany, France, and Spain for the 2012-2013 season.
The dataset contained demographic information on each player, the interactions they had with referees across their professional career, the number of games in which a referee and player interacted, and the number of yellow and red cards each player had received in total. Each player also had a rating for skin tone, ranging from very light skin to very dark skin, which was calculated from two independent blind raters’ coding of player photos.
The research teams had to make certain statistical assumptions and analytical decisions, including how to account for factors such as the seniority of the referees, the proportion of dark skin-toned players to light skin-toned players in a league, referees’ familiarity with a player, and the fact that some referees give out more red cards than others.
Each team decided on the type of statistical approach to take and which covariates to include, and reported their analytic strategy and results. In a round-robin of peer evaluations, each team viewed the analytical approach of every other team without seeing their results and provided feedback. That way, each team had the opportunity to improve their analytic strategy.
In a second round, teams could change their analytic strategy and form new conclusions. All the teams then participated in an internal peer review. Each analyst was assigned to assess the success of one to three other analytic strategies, based on his or her area of statistical expertise.
The final reports demonstrated a range of effect sizes for the relationship between skin tone and number of red cards received. The effect sizes ranged from .89 odds-ratio unit, a small negative effect indicating that players with darker skin tones were less likely to receive red cards than their peers with lighter skin, to 2.93 odds-ratio unit, a moderate positive effect indicating that players with darker skin tones were more likely to receive red cards.
Twenty teams found a statistically significant positive effect, while the other nine found no significant effects; no teams found a significant negative effect. Overall, the 61 analysts used 21 unique combinations of covariates, and logistic models tended to find larger effect sizes than linear models.
At multiple stages of analysis and reporting, the authors asked analysts to report their expectations about the magnitude of the relationship. The authors found that analysts’ prior beliefs about the effect did not explain the variation in outcomes, nor did a team’s level of statistical expertise or the peer ratings of analytical quality.
The authors emphasize that crowdsourcing protects against selection bias, or choosing certain data to analyze to produce a desired result, because one team’s results will not influence the overall likelihood of the findings being published.
They also suggest that variations in analytic results might be difficult to avoid. As crowdsourcing becomes a more common method of analyzing datasets, policymakers and other leaders will need to decide how much variability is too much, setting guidelines about when to trust (or not trust) certain analyses.
Future crowdsourcing projects should investigate the effect of open-ended research questions and greater choice in the measures of interest. For example, how would the results change if the researchers were able to choose the types of referee decisions that are most useful instead of just using data on red cards?
Crowdsourcing is an extensive project that takes vast resources, the authors note. For researchers who do not have the means to crowdsource data, the authors recommend using a specification curve or multiverse analysis to model the outcomes of every defensible analysis of a dataset and compute the likelihood of significant results.
Reference
Silberzahn, R., Ujlmann, E. L., Martin, D. P., Anselmi P., Aust, F., Awtrey, E., … & Nosek, B. A. (2018). Many analysts, one data set: Making transparent how variations in analytic choices affect results. Advances in Methods and Practices in Psychological Science, 1(3). https://doi.org/10.1177%2F2515245917747646
APS regularly opens certain online articles for discussion on our website. Effective February 2021, you must be a logged-in APS member to post comments. By posting a comment, you agree to our Community Guidelines and the display of your profile information, including your name and affiliation. Any opinions, findings, conclusions, or recommendations present in article comments are those of the writers and do not necessarily reflect the views of APS or the article’s author. For more information, please see our Community Guidelines.
Please login with your APS account to comment.