Research Preregistration 101
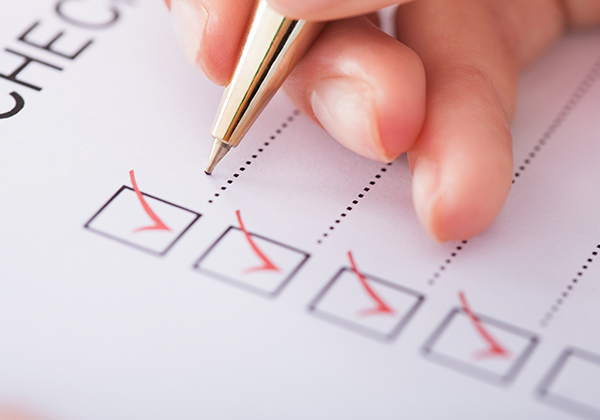
APS President Susan Goldin-Meadow recently published an Observer column titled “Why Preregistration Makes Me Nervous.” We suspect that many psychological scientists share Goldin-Meadow’s uncertainties about preregistration. In this article, we attempt to allay those concerns by explaining the rationale for and benefits of preregistration for researchers and for the field of psychology at large. We also include some explanations of different types of preregistration and answers to frequently asked questions.
A Problem: Reporting Flexible, Post hoc Analyses as if They Had Been Planned
Imagine you conduct a study testing whether symmetrical faces are more attractive than asymmetrical ones. Suppose that you find no overall difference in attractiveness, so you test whether the effect differed as a function of the gender of the participant and the gender of the face. Looking at the means, you see that men found symmetry attractive for faces of both genders, whereas women found symmetry attractive in women’s faces but asymmetry attractive in men’s faces. That interesting interaction pattern was not statistically significant. Examining the data more closely, though, you notice that some faces were rated as maximally attractive by almost everyone, so you drop those faces from the analysis because they might obscure a real effect. Moreover, some participants were older than the rest and their ratings don’t seem to fit the pattern, so you exclude their data, too. Now the interaction becomes statistically significant. “Eureka!” you cry.
In the past, results selected from such freewheeling exploratory analyses often were reported as if they were theory-driven and planned. Many researchers generated or changed their hypotheses after looking at the data but reported their research as if those hypotheses had motivated the study in the first place (a practice known as “hypothesizing after the results are known,” or HARKing; Kerr, 1998). Also, researchers often tweaked their measures or designs while analyzing their data and then selectively reported just those outcomes that best supported the story they wanted to tell. Similarly, they sometimes conducted a series of studies, reporting only the most “successful” ones and treating the others as failed pilot studies. This approach to analyzing data and reporting findings was recommended most famously by Daryl Bem (1987), but we believe it was widespread long before then.
Researchers taking this approach almost always believe that their selectively reported evidence is also the best evidence — they’re choosing results not because they are the prettiest, but because they truly believe those are the “right” analyses. But these flexible, post hoc approaches to data analysis and reporting have come under intense fire in the last 6 years. Different critics have used several terms and highlighted multiple aspects of the problem (in addition to HARKing, terms include researcher degrees of freedom, Simmons, Nelson, & Simonsohn, 2011; p-hacking, Simmons, Nelson, & Simonsohn, 2012; the garden of forking paths, Gelman & Loken, 2014; and questionable research practices, John, Loewenstein, & Prelec, 2012). What is hard to realize is that our intuitions about which analyses are “right” likely are influenced by the results of those analyses and by how much we want to believe in those results.
The problem is not data exploration. Exploration can lead to new ideas and discoveries — it’s a generative, creative, fun, and key component of science, and all researchers should fully examine and explore their data. Exploration often helps uncover unexpected patterns that merit further study. It helps researchers avoid overlooking effects (Type II errors). But exploration differs from planned hypothesis testing, and the results of standard inferential hypothesis tests (i.e., p values) are valid only when evaluated in the context of the full range of tests that were entertained
(Wasserstein & Lazar, 2016).
Flexible analytic practices — exploring your data to decide which hypotheses to test and how to test them — dramatically increase the chances of erroneously rejecting null hypotheses (Type I errors). Even though the interaction between gender of faces and gender of participants was “significant” in the example we’ve described, the odds that it was just a fluke are high as a result of flexibility in analyzing the data. When several such strategies are combined, the Type I error rate can soar from the ostensible .05 level to a staggering .61 or more (Simmons et al., 2011). Thus, flexibly choosing which statistical tests to conduct after inspecting your data makes it easy to obtain statistical significance when, in reality, there is no effect at all. It also leads to exaggerated estimates of the size of real effects, which increases the likelihood that subsequent replication attempts will be underpowered and at high risk of failure (Button et al., 2013) and renders meta-analytic summaries of the literature less accurate. More generally, in the words of Wasserstein and Lazar (2016) of the American Statistical Association, “proper [statistical] inference requires full reporting and transparency.”
Research projects unfold across months or years, so even with the best of intentions, you might not remember which predictions, analyses, exclusions, transformations, covariates, and so on were planned and which were post hoc. When you finally get around to writing a paper, it’s easy to forget that you had planned to analyze means rather than medians, or that you hadn’t planned to analyze men’s and women’s faces separately. You might even forget that you conducted another study similar to the one you’re reporting — a study that, for some reason, did not yield the predicted effects. Moreover, even if you do remember and report all and only the statistical tests that were planned in advance as such, how can you demonstrate that to others?
A Solution: Preregistration of Research Plans
Preregistering a research project involves creating a permanent record of your study plans before you look at the data. The plan is stored in a date-stamped, uneditable file in a secure online archive. You can give others (e.g., reviewers) access to the
preregistered plan, and you can do so while maintaining your and the reviewers’ anonymity. The main purpose of preregistration is to make clear which hypotheses and analyses were specified a priori and which were more exploratory and driven by the data.
Preregistered research plans supplement our malleable, imperfect memories (Nosek, Spies, & Motyl, 2012). Good documentation of your original plan can help ensure that your report accurately represents what you actually planned. If editors or reviewers ask about flexibility in your data-analysis choices or in your predictions, your preregistered research plan will put any concerns to rest. Your preregistered plans also provide a long-term record of your laboratory’s workflow — you can return to your plans years later to review your original hypotheses and analysis plans. And, if you upload your materials, plans, and results to the same site as your preregistered plan, you can see the entire research process for a completed study in one place, making it easy for you to share with others.
You can preregister every detail of your study, uploading all of your research scripts, coding schemes, data-collection plans, analysis code, and so on. Alternatively, you can preregister a more minimal plan. You can preregister competing hypotheses, and you can plan tests without having a specific prediction regarding the outcomes. You also can preregister your lab’s “standard operating procedures” (e.g., how you define outliers, standard transformations) and refer to that documentation to simplify the process of registering individual studies. Even if you are collecting a set of measures for entirely exploratory purposes, you can preregister that plan. Such a preregistration will remind you and inform reviewers that you conducted the study without specific analyses and predictions in mind. That said, the more detail you provide and the better the documentation that your analyses and predictions were made a priori rather than post hoc, the more confidence readers can have in your interpretation of the results of the inferential statistical tests you report.
Just because you have preregistered a plan does not mean that you must stick to that plan regardless of what happens. In many cases, it makes sense to examine your data in ways that you did not anticipate. Preregistration does not stifle exploration, and it does not preclude changing your mind after you look at your data. It does not prevent you from examining your data fully, testing additional hypotheses inspired by your data, or trying out different analyses. In fact, it may encourage further exploration of your data because you no longer need to pretend that any discoveries were predicted. Any analyses that you preregistered are truly a priori, planned ones. If you deviate from your plan, you can report what you did and why you did it to provide full transparency. You should always report your preregistered analyses even if you also report other analyses — doing so provides an accurate accounting of the analyses that were planned versus unplanned. Patterns observed in more exploratory analyses can be put to more rigorous tests in subsequent preregistered research. With reference to our face-attractiveness example, you could preregister a follow-up study in which you plan to exclude older subjects and faces with ceiling-level attractiveness and predict the specific interaction that emerged in your exploratory work.
In our own research, we have found that working on a preregistration pushes us to be clear and precise about our study plans, what we expect to find, and what we think our findings will mean. The first time you preregister a detailed analysis plan, you will realize how many flexible decisions you could have made when choosing your analyses after seeing the data and how those decisions could have misled you about the results of your study. Working with students on the preregistration process can be deeply rewarding for all parties. In our own labs, engaging in this thoughtful planning process has enhanced our understanding of projects and has saved us from running some poorly conceived studies. For a terrific (and more extensive) treatment of preregistration, see van ‘t Veer and Giner-Sorolla (2016).
Neither of APS’s empirical journals, Psychological Science and Clinical Psychological Science, require preregistration, although they encourage it. Of the three authors of this piece, only Simons has already published preregistered research from his laboratory, but we all have begun preregistering our new and ongoing research. In our work as editors and reviewers, we put more stock in submissions that report preregistered studies (all else being equal). In the words of Bob Dylan, “the times, they are a-changin’.” In our view, the adoption of preregistration means they’re changing for the better. Preregistration is like washing your hands — it is good hygiene and good for the herd, and everyone should do it.
Types of Preregistration
Preregistration (a.k.a. unreviewed preregistration)
The researcher creates as detailed a description of his or her plans for a study as possible and saves those plans in a time-stamped, uneditable archive. This record can be shared with reviewers, editors, and other researchers.
Registered Reports (a.k.a. reviewed preregistration)
The researcher submits a detailed proposal for a study to a journal before conducting the study. These registered reports have the same virtues as preregistration, but they also address the problem of publication bias because the studies are published regardless of their outcomes. Registered Reports are most useful for well-defined research domains in which reviewers can reasonably assess the likelihood that a proposed study will be informative regardless of its outcome. (Click here for a list of such journals and for a more extensive discussion.)
Registered Replication Reports (RRR)
A variant of Registered Reports, RRRs are focused on direct replication of one or more original findings. Many labs follow the same preregistered plan, and the results from all of those independent studies are published collectively regardless of the outcomes of individual studies. Variants of such reports include RRRs in Perspectives on Psychological Science and the ManyLabs project, among others.
FAQs About Preregistration
Q: Where and how can I preregister my studies?
A: You can preregister your study plans in many ways, all of which are accessible and free. Sites like AsPredicted.org make preregistration quick and easy using a Web form that works well for many simple experimental designs. The Open Science Framework (OSF) allows for both simple preregistration and complete specification of all aspects of your study — you can upload files, scripts, images, and so on. The OSF site provides a detailed example of how to do a preregistration. That example uses a template (OSF provides a number of those), but you don’t have to use one. In fact, you can type your plans in a Word file, upload that to OSF, and preregister it. You can use these tools to create a view-only link or a PDF that allows an anonymous reviewer to see your plans. Other options for preregistration include ClinicalTrials.gov, AEA Registry, Evidence in Government and Politics, and trial registries in the World Health Organization Registry Network. See van ‘t Veer and Giner-Sorolla (2016) for additional resources related to preregistration.
Q: Doesn’t preregistration take a lot of time and effort?
A: It takes some time before you start your study, but it can save you time later. Think of it as writing the core of your method section in advance of conducting the study. Once you have preregistered one study, subsequent preregistration plans for follow-up studies can be completed quickly and easily. If you post all of your materials as part of your preregistration documentation, you also will have a permanent record of what you did that will make it easier for you and others to reproduce your procedure years later.
Q: I can see preregistering laboratory experiments but not field work, archival analyses, longitudinal research, etc. Does it make any sense to preregister those sorts of research?
A: Yes. Any quantitative research should be preregistered if the results are to be analyzed with standard inferential statistical tests. Preregistration just means describing what you plan to do; even field studies are based on plans. You can preregister your plans for archival research, field observations, longitudinal studies, survey research, meta-analyses, and so on. Indeed, preregistration may be especially valuable in correlational research (e.g., large data sets provide vast opportunities for HARKing). For an exploratory study, the preregistration would be brief — perhaps just listing the measures you will collect and the sorts of analyses you might try. For a long-term longitudinal study, you could produce incremental preregistrations (e.g., an initial preregistration might spell out the first phase in detail and leave the second phase more open). For a field study, you might preregister your plans for developing a coding scheme.
Q: Does the preregistration have to be completed before data collection begins?
A: No. As long as you have not examined the data, you can create a preregistration after data collection starts or even after it is complete (in the case of archival data, it might be years later).
Q: Do I have to conduct the study exactly as described in the preregistration?
A: No. A preregistration is not binding, and you are free to change your mind about your plans. When your procedures depart from your preregistered plan, though, you must acknowledge such differences and recognize that your hypothesis tests might not be truly a priori. Reviewers and readers can make informed assessments of the appropriateness of your departures from the plan. The key is to be transparent about which aspects were entirely planned and which were determined at least in part by your knowledge of the data.
Q: Most researchers are honest, so why do we need preregistration?
A: Preregistration does not assume dishonesty. HARKing and similar practices that preregistration cures typically are unintentional rather than deliberate. Preregistration helps us remember (and show others) what we actually planned.
Q: I have always distinguished between exploratory research and planned research in my writing. Why should I bother formally preregistering?
A: Why not? It documents your adherence to best practices and serves as a crucial check on your memory.
Q: In my lab, we check results obtained in exploratory analyses by replicating them in follow-up studies.
A: Terrific (especially if you report all of your follow-up studies, not just those that yielded the prettiest results). But all the more compelling if you can show reviewers your preregistered plans for those replications.
Q: What are the criteria for qualifying for a Preregistration Badge for manuscripts accepted for publication in the APS journals Psychological Science and Clinical Psychological Science?
A: Click here.
Q: Won’t preregistering cramp my style?
A: Only if your style relies on presenting exploratory analyses as though they had been planned.
Q: Couldn’t a researcher intent on cheating produce a faux preregistration by collecting and analyzing data first and then “preregistering” that “plan” later?
A: Yes. Preregistration helps honest researchers do good work, but it is not designed to prevent fraud (although it may make it harder).
Q: Are there other advantages to preregistering?
A: Preregistration can make your research more efficient. With preregistration, you can adopt sequential testing procedures that can dramatically reduce the number of participants needed to test a hypothesis (Lakens, 2014). Without preregistration, deciding whether to collect more data after looking at the results (optional stopping) is a form of p-hacking that inflates your chances of accidentally obtaining a false-positive result. With preregistration, you can divide your alpha level to account for peeking-and-deciding, keeping your false-positive rate constant while stopping sooner if you find a significant result. But it is valid only with a preregistered plan that specifies when you will peek at your data and how you will adjust your alpha level to keep your error rate constant. (Note, though, that sequential testing even with preregistration will inflate your effect-size estimates, so its value is limited to maintaining a fixed false-positive rate.)
Q: Can I win money by preregistering?
A: Yes! The Center for Open Science will pay 1,000 researchers $1,000 each for publishing projects preregistered on the Open Science Framework. Click here for more information.
References
Bem, D. J. (1987). Writing the empirical journal article. In Darley, J. M., Zanna, M. P., & Roediger, III, H. L. (Eds.), The compleat academic: A career guide (pp. 171–201). Washington, DC: American Psychological Association.
Button, K. S., Ioannidis, J. P. A., Mokrysz, C., Nosek, B. A., Flint, J., Robinson, E. S. J., & Munafò, M. R. (2013). Power failure: Why small sample size undermines the reliability of neuroscience. Nature Reviews Neuroscience, 14, 365–376. doi:10.1038/nrn3475
Gelman, A., & Loken, E. (2014). The statistical crisis in science: Data-dependent analysis — a “garden of forking paths” — explains why many statistically significant comparisons don’t hold up. American Scientist, 102, 460. doi:10.1511/2014.111.460
Lakens, D. (2014). Performing high‐powered studies efficiently with sequential analyses. European Journal of Social Psychology, 44, 701–710. doi:10.1002/ejsp.2023
Nosek, B. A., Spies, J. R., & Motyl, M. (2012). Scientific utopia: II. Restructuring incentives and practices to promote truth over publishability. Perspectives on Psychological Science, 7, 615–631. doi:10.1177/1745691612459058
Simmons, J. P., Nelson, L. D., & Simonsohn, U. (2011). False-positive psychology: Undisclosed flexibility in data collection and analysis allows presenting anything as significant. Psychological Science, 22, 1359–1366. doi:10.1177/0956797611417632
Simmons, J. P., Nelson, L. D., & Simonsohn, U. (2012). A
21-word solution. Retrieved from http://ssrn.com/abstract=2160588
van ‘t Veer, A. E., & Giner-Sorolla, R. (2016). Pre-registration in social psychology — A discussion and suggested template. Journal of Experimental Social Psychology, 67, 2–12. doi:10.1016/j.jesp.2016.03.004