First Person
Careers Up Close: Chris Street on Lie Detection, Truth Biases, and Developing an Adaptive Lie Detector Account
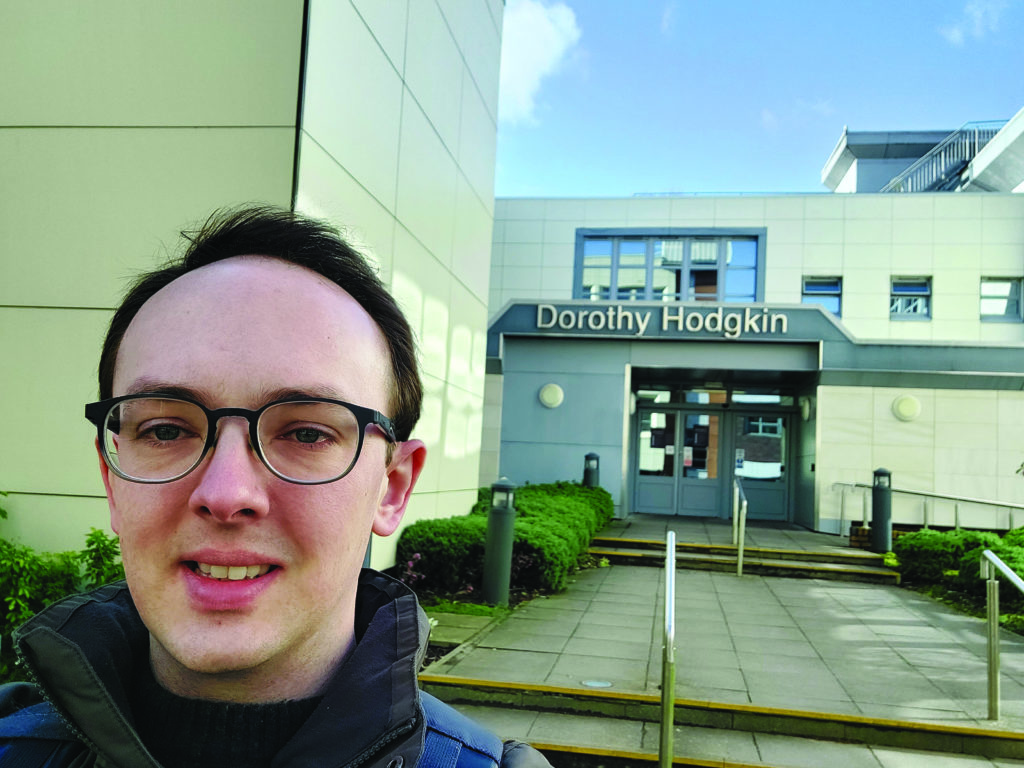
Photo Above: Chris Street stands outside the Dorothy Hodgkin building for the School of Psychology at Keele University, UK.
Chris Street is a senior lecturer in cognitive psychology at Keele University in the United Kingdom. His research focuses on lie detection and truth biases, and he is currently working to develop the first computational model of lie/truth judgments.
Current role: Senior lecturer in cognitive psychology, Keele University, 2021–present
Previously: Reader in cognitive psychology, University of Huddersfield, 2019–2021; senior lecturer, University of Huddersfield, 2017–2019; lecturer, University of Huddersfield 2015–2017
Terminal Degree: PhD in psychology, University College London, 2013
Recognized as an APS Rising Star in 2019
Landing on lying
I did my undergraduate study at the University of Dundee under Ben Tatler, a vision scientist. I volunteered in his lab for 3 years and even got a small summer grant to run my own research, and so I figured I would end up researching high-level visual perception.
But while attending University College London, my PhD supervisor, Daniel Richardson, and I were supervising an undergraduate project student who wanted to understand how people lie. Neither of us knew that literature, and so I did a bit of digging, finding that many of the questions around how people make a lie/truth judgment had not been explored.
I began my PhD study by asking, “What is the truth bias?” My answer, I think, is that there is no truth bias—at least not in the traditional sense.
I would argue that there is no built-in way of thinking that influences people toward believing others. Instead, my work is arguing that people are making informed judgments, and that this just happens to present itself as more judgments of truth than of lie.
Chris Street
While working as a postdoctoral fellow at the University of British Columbia in Vancouver, my work was largely around my supervisor’s area of interest, so I carried out studies on how people hide and find items and whether these actions share overlapping functionality. I did manage to write up a number of my PhD studies while there, though, and this is where I first published my Adaptive Lie Detector account (ALIED).
Contributing to the field
When I was recognized as an APS Rising Star in 2019, I was working on understanding how people decide if someone is lying or telling the truth. It turns out that people tend to guess that others are telling the truth. At the time, I was working on the ALIED account of this “truth bias.” The account argues that the bias is not an error or cognitive default. Instead, the truth bias can be seen as functional and adaptive when there is no reliable information about the speaker’s statement. In such a situation, it is reasonable to rely on how frequently deception and honesty are typically encountered to make an informed guess—and given that people tend to tell the truth most of the time, it is reasonable to be biased toward guessing that others are telling the truth.
ALIED claims that when people try to assess whether someone is lying, they try to rely on the more reliable information about the specific statement. There could be CCTV footage confirming the statement, for example, which would be quite reliable, or the speaker’s nonverbal behavior as they deliver their statement could appear to be unreliable. These cues that relate to the specific statement being evaluated are referred to as individuating cues.
As these individuating cues become less reliable, context-general information weighs more heavily into the decision. Context-general information is information that does not causally relate to the current statement, but rather generalizes across statements. In principle, ALIED is a Bayesian updating account.
Since being recognized as an APS Rising Star, I have been working with Professor David Peebles at the University of Huddersfield to develop the first computational model of lie/truth judgments. We developed the ALIED account under the ACT-R cognitive architecture, a framework that instantiates the basic assumptions of cognition (e.g., that memory exists, has a decay rate that can be mathematically specified, etc.). I am currently working with colleagues to try to find ways to falsify the claims of the account.
Developing lie-detection research
The ACT-R model of ALIED instantiates core cognition: perceiving the world, retrieving memories about the world, having goals to achieve, and being able to act upon the world. In brief, the model functions by observing the world, retrieving similar past experiences from memory, and using those experiences to decide whether someone is lying or telling the truth.
Initially, the model is shown a set of liars and truth-tellers exhibiting a behavior (e.g., scratching one’s nose). In this way, the model comes to learn the frequency with which behavioral cues are associated with honesty and deception. These behaviors are the individuating cues in ALIED. In a later test phase, the model sees a set of liars and truth-tellers displaying a behavior but is not told if the speakers are lying or telling the truth: It is for the model to decide. The model attempts to retrieve from memory two “chunks” of information: (a) “this behavior indicates honesty” and (b) “this behavior indicates deception.” Only one of those chunks of information will be retrieved, and this will be the judgment that the model makes. The retrieval of the behavior’s association with either honesty or deception depends on the frequency with which the behavior has been associated with honesty or deception in the past, noise in the cognitive system, the recency with which the association has been observed, and so on. Each of these affects how “active” the chunk is in memory. The most active chunk in memory is retrieved, and this is the decision made by the decision-maker.
However, the above only takes into account individuating cues. ALIED also posits a role for context information. The current context in which the decision is being taken will impact which chunk is retrieved. When a chunk in memory is inconsistent with the current context, its activation is penalized, making it less likely to be retrieved from memory. When an individuating cue is highly reliable (e.g., the CCTV example above), context has relatively little effect in influencing the judgment outcome. But when an individuating cue is less reliable (e.g., avoiding eye contact, which is shown no more by liars than truth-tellers), there will be a larger effect of context, such that the chunk that is consistent with the context will be more likely to be recalled. That is, judgments are more likely to be aligned with the context-general belief when the individuating cues are unreliable.
We have developed the model on the basis of historical data, which provides a very high fit to the data, and then tested novel predictions that result from this computational model with new data. The model was not falsified by the new data testing those predictions, which we believe offers credibility and strength to our model.
The ACT-R model of ALIED is grounded in core cognitive principles and is generating novel predictions that are standing up to the test of novel data. The model is currently the only explanation of lie/truth judgments that can explain the cognitive processing from receiving information to the eventual judgment, which makes it, in my opinion, the most useful account of lie/truth judgments that we currently have. I say “useful” and not “accurate” because I hope there will be strong falsification attempts that lead us to revise our understanding and develop the account further.
Preventing the initial credibility of misinformation
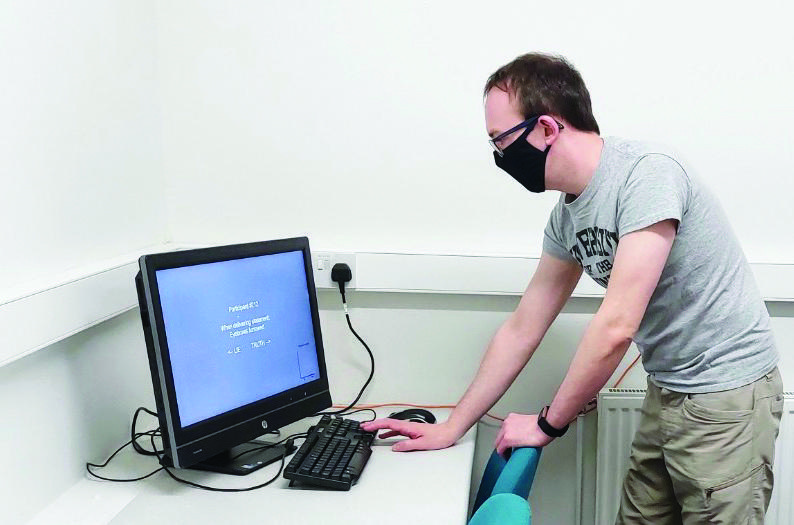
People tend to believe that others are telling the truth. The traditional explanation in the lie-detection field is that we have some built-in bias or default that makes us believe things are true. But ALIED takes the perspective that our beliefs are informed and sensible, and not the result of some cognitive machinery that we do not have control over. We attempt to use information that we perceive to be reliable, and what we mean by this is that in the past, this information has been a good predictor of reality (e.g., what is said in a particular magazine matches my experiences of the world in the past).
Of course, people may be incorrectly making those associations as a result of, for example, memory errors. The issue, from a lie-detection perspective, is not so much one of combatting a built-in error, but rather preventing misinformation from being presented in ways that make it seem that it came from credible and reliable sources. I have not done work in this specific area, so I am being somewhat speculative here.
Solving puzzles
What I most enjoy about my work is those days where I have time to sit down and think through ideas. Playing around with concepts and theories and reading new and different interpretations into findings and predictions makes me feel engaged and gives that sense of flow that you get from solving a Sudoku or logic puzzle.
Biggest challenges so far
COVID! I had two externally funded research projects running during the span of COVID-19 lockdowns in the United Kingdom. These were also my very first funded projects. The lockdowns prevented data collection and attendance at conferences and training workshops. They also forced me to put my research assistants’ posts on hold, which had knock-on effects for collaborator engagement, data extraction and analysis, producing outputs, and more.
The importance of forming a network
I think an important part of inspiring early-career researchers is developing a friendly community ethos. Feeling like you are part of a strong group that works together, can socialize, and makes collective decisions can give people the space and confidence to explore and be creative.
Forming a network of collaborators is also becoming more and more important as research is becoming more interdisciplinary. Develop your skill sets and try to network when you can. I am not a social butterfly, so I guess that’s an area for me to work on.
Viewing research questions without presumption and with creativity is a must, in my opinion. You have to understand the field you are working in, of course, but being able to put aside the views of the vocal minority in the field and taking a more holistic approach that reaches across disciplinary boundaries gives you a real opportunity to generate new and robust insights.
Life beyond academia
I am afraid to say that I have been considering leaving academia. It is such a shame to say that because working as a researcher and educator has been inspiring and allowed me to explore some of the important questions in my area. Hopefully I will remain in academia, but time will tell. Should I continue here, I want to be able to say that I have developed a predictive theoretical account that can explain how people decide what to believe and what to trust and that has stood up to falsification attempts.
Feedback on this article? Email [email protected] or scroll down to comment.
APS regularly opens certain online articles for discussion on our website. Effective February 2021, you must be a logged-in APS member to post comments. By posting a comment, you agree to our Community Guidelines and the display of your profile information, including your name and affiliation. Any opinions, findings, conclusions, or recommendations present in article comments are those of the writers and do not necessarily reflect the views of APS or the article’s author. For more information, please see our Community Guidelines.
Please login with your APS account to comment.