APS Spotlight
2020 APS Janet Taylor Spence Awards for Transformative Early Career Contributions
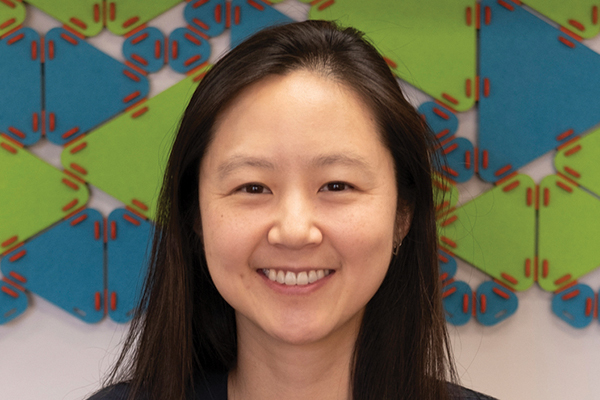
Eight psychological scientists have been recognized with the 2020 APS Janet Taylor Spence Awards for Transformative Early Career Contributions. Named for APS’s first elected president, the award honors the most creative and promising researchers who embody the future of psychological science. This year’s recipients were selected for their innovative research impacting areas from advocacy related to migrant children facing family separation to using machine learning and cognitive modeling to understand why errors occur in medical image-based decision making.
The Observer asked recipients to share their proudest achievements and ongoing research.
Learn more about the Spence Awards, and see past recipients, at psychologicalscience.org/aps-spence-award.
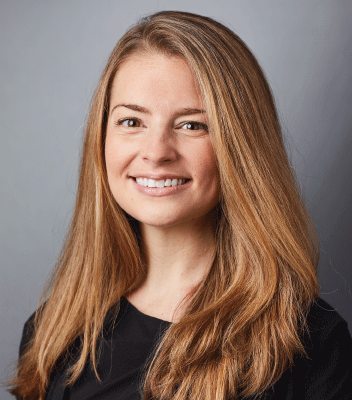
Dylan Gee
Yale University
Clinical Affective Neuroscience & Development Lab (CANDLab)
My program of research focuses on anxiety and stress-related disorders in childhood and adolescence. My lab examines how early experiences such as caregiving and trauma shape the development of threat and safety learning, emotion regulation, and risk for anxiety disorders and PTSD. A central focus of this work is on sensitive periods and developmental changes in frontolimbic circuitry and emotional behavior, with the goal of translating this knowledge to optimizing treatments for children and adolescents.
I am really proud of the impact that my lab and I have had on policy and advocacy related to migrant children facing family separation and other atrocities at the US/Mexico border. At various critical points, my lab has come together to support justice for migrant children, including leading efforts to communicate science to the public and policymakers, disseminate resources for migrant families, and generate recommendations for best practices and treatment. I am particularly proud to have contributed a legal declaration detailing findings on early-life trauma that was cited in the judge’s ruling that the US government must provide mental health screening and care to all children and their caregivers who were separated.
I am particularly excited about two lines of research that my lab is beginning to tackle. First, we have been thinking deeply about how to parse the vast heterogeneity in early environments to more precisely delineate how key features of stress exposure influence the developing brain and behavior. Second, we are examining how individuals learn about safety in their environments (e.g., in the context of caregivers) and apply that learning to regulate fear.
Read the full interview with Dylan Gee.
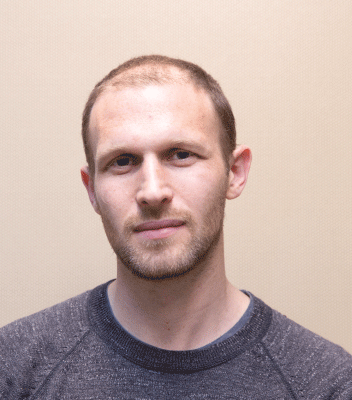
Samuel Gershman
Harvard University
Computational Cognitive Neuroscience Lab
My interests are broad: My lab works on a wide variety of topics ranging from synaptic plasticity and Pavlovian conditioning in animals to language, perception, and reasoning. The main focus of my lab’s research has been on reinforcement learning and decision making. We’re interested in what representations
and algorithms the brain uses to act adaptively in the world. Of particular interest
has been the question of structure: How do we extract the latent structure underlying our observations, and how do we harness this structure to improve decision making?
As an undergrad, I remember learning about the hypothesis that dopamine encodes a reward prediction error. This struck me not only as an extremely important idea, but also as a case study in how computational models could contribute to the advancement of neuroscience and psychology. As I delved deeper in graduate school, and then later as a postdoc and professor, I realized that the idea, despite its appealing simplicity, was incomplete. There were many gaps in the experimental literature, so one of the tasks that I set for myself was to enrich this idea, to equip it with the theoretical accoutrements necessary to explain the empirical data. I predicted that under certain circumstances, dopamine responses would be a nonmonotonic function of reward magnitude. This turned out to be correct, and it built a trust in our theoretical models that we built upon in future collaborations.
I’m very interested in how high-level cognition, particularly “intuitive theories” (theory of mind, intuitive physics, etc.), contribute to reinforcement learning. We are now trying to tackle human video game learning as a problem of theory induction and theory-based planning.
Read the full interview with Samuel Gershman.
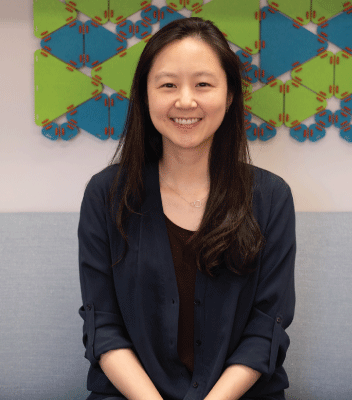
Hyowon Gweon
Stanford University
Social Learning Lab
I am interested in how we learn from others and help others learn. While humans are not the only species that engages in social learning, only humans incrementally build knowledge over generations and create cultural institutions (e.g., schools) to facilitate this process. What makes human social learning so distinctive, powerful, and effective? Our studies use a range of methods (developmental, computational, neuroimaging) to better understand the cognitive processes that support our abilities to learn from others (as learners) and help others learn (as teachers).
One example is our latest published work (Bridgers, Jara-Ettinger, & Gweon, 2020). It’s one of the first projects we launched as I set up my own lab at Stanford, with one of my first graduate students, Sophie Bridgers. We started with a seemingly simple question: How do we know what to teach? With Julian Jara-Ettinger (Yale), we turned the question into a project that combines computational and developmental methods. We presented a computational model of how we choose what to teach and empirically tested its quantitative predictions with young children. The take-home message is that humans, even as young children, readily consider what is rewarding or difficult for someone to learn and choose to teach what maximizes the learner’s expected utilities.
With postdoc Yang Wu, and graduate students Mika Asaba and Aaron Chuey, we are extending our work on social learning in a few directions, including what counts as data in learning from others, how children learn about the self from others, and children’s intuitive theories about knowledge transfer.
Read the full interview with Hyowon Gweon.
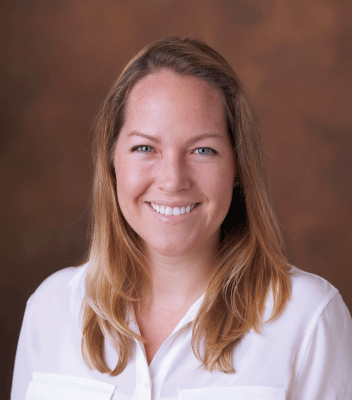
Kathryn Humphreys
Vanderbilt University
Stress and Early Adversity (SEA) Lab
My research focuses on early experiences, based on an understanding that early development is a period of increased brain plasticity and that caregiving environments are essential for supporting positive developmental trajectories. This research typically falls into three interrelated lines: 1) characterizing how early environments vary (e.g., differentiating neglect from abuse as well as assessing the consistency, cognitive, and emotional enrichment of environments), 2) linking variations in the early environment to child outcomes (e.g., psychopathology, cognitive and adaptive functioning), and 3) identifying predictors of children’s environments (e.g., caregiver characteristics). The broader goal is to identify targets for interventions aimed at improving caregiving experiences, and, in turn, promoting positive long-term outcomes in children.
So much of what we assume about children’s caregiving environments comes from either self-report measures or laboratory-based observations. While both have been extremely valuable in knowledge generation, there remains a large gap in what we know about children’s experiences in their natural environments. I am working with computer engineers to develop wearable devices to assess the ways in which children, parents, and family networks physically interact throughout their days. If we can get this to work, we will be able to gain a better appreciation of how interactions change across development stages, how children vary in their interactions with their families, and what those interactions might mean for specific areas of functioning (e.g., language development, attachment security).
Read the full interview with Kathryn Humphreys.
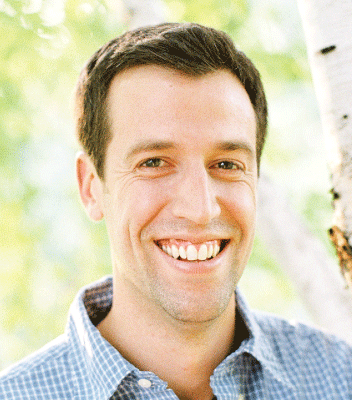
Luke Hyde
University of Michigan
Michigan Neurogenetics and Developmental Psychopathology (MiND) Lab
I am broadly interested in understanding brain and behavioral development in the context of risk and resilience. Specifically, my lab has focused on 1) the development of antisocial behavior, 2) the development of psychopathy and callous-unemotional traits, 3) the risk and protective roles of parents and neighborhoods, 4) the impact of poverty-related adversity on brain and behavioral development, and 5) how youth and families succeed when faced with adversity (i.e., resilience).
I’m proud of our work showing that parenting is an environmental/non-heritable risk factor in the development of callous-unemotional traits, because I think it counters iatrogenic beliefs that callous-unemotional traits are not treatable or that “psychopaths are born” and thus there is little we can do to promote positive development in these youth. I am also proud of the work we are doing now to take a more population-level approach to developmental neuroscience. In both cases, the factors that have led to success have to do with incredible mentees and team members in my lab, and incredible and generous collaborators.
I hope that my lab will focus more on resilience in the near future. We already know that poverty and its related adversities are harmful for youth. We need now to better understand why so many youth and families still thrive, even in the context of adversity. Beyond a near-term focus on resilience, I hope that my lab will eventually be doing personalized interventions, preventative interventions, or policy initiatives in the long-term that are informed by the work we’re doing now. Ultimately, I feel strongly that our work must be translated to be impactful at the societal level.
Read the full interview with Luke Hyde.
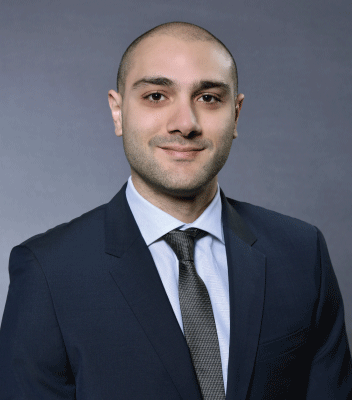
Nour Kteily
At the broadest level, I’m interested in the psychological forces that help to explain why human societies are so reliably organized into relatively stable social hierarchies, with some groups enjoying privileges at the top and others languishing without at the bottom. In my work, I try to better understand the mechanisms by which hierarchy is maintained (and sometimes challenged). As one of the mechanisms that has historically contributed to the domination and exploitation of disadvantaged groups, the topic of blatant dehumanization has interested me in recent years.
I’m particularly proud of a pair of papers that explored the psychological experience of feeling dehumanized by another group (which we call meta-dehumanization). Despite its importance, little research had considered the experience of being on the receiving end of dehumanization. In one paper, we examined the extent to which Muslim-Americans and Latinx-Americans felt dehumanized in the context of the rhetoric surrounding the 2016 election, which we felt was a perspective that needed illuminating.
One of the new and ongoing research projects I’m working on that’s especially exciting for me these days asks how groups formerly occupying disadvantaged positions in a social hierarchy act when the tables turn and their group rises to the top. Specifically, we consider how they treat their former oppressor and third-party disadvantaged groups, depending on a variety of contextual factors prior to shifts in the hierarchy. This research could help us better understand the processes by which hierarchies tend to reproduce over time.
Read the full interview with Nour Kteily.
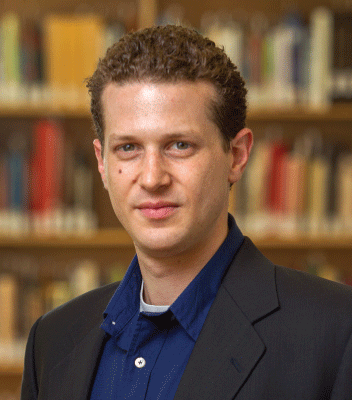
Amitai Shenhav
Brown University
Shenhav Lab
I’m interested in what makes thinking hard, and why we still do it anyway. More specifically, my lab studies the neural and computational mechanisms that intersect decision making (determining the right course of action) and cognitive control (pursuing the right action despite competing inclinations). We use a combination of computational modeling and measures of behavior and neural activity to understand what makes cognitive tasks effortful, including what makes decision making effortful; when and how people determine that mental effort is “worth” the investment; and what makes some people more willing to invest cognitive effort than others.
One of the reasons we want to understand the mechanisms that drive decisions to engage in cognitive effort is to understand how these mechanisms differ for individuals who have difficulty motivating themselves to perform cognitively demanding tasks. Such motivational impairments are pervasive across psychiatric and neurological disorders, including individuals with depression, schizophrenia, and Alzheimer’s, and can be severely debilitating. Our goal is to identify which of a variety of deficits determine these impairments (and/or their subtypes), by combining our modeling with behavioral and neural data we collect using tasks that target different aspects of how people process incentives in their environment.
In addition to understanding how these mechanisms differ across individuals, we are also eager to understand how they develop within an individual over time. In particular, we want to better understand how one’s childhood environment helps shape perceptions of the incentives to engage in different forms of effort. We have started to look at how these early experiences shape adult cognition, but in the coming years we hope to extend this research into earlier stages of development as well.
Read the full interview with Amitai Shenhav.
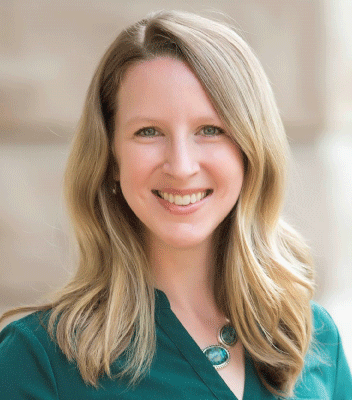
Jennifer Trueblood
Vanderbilt University
Computational Decision-Making Lab
My research is aimed at understanding how people make decisions when faced with multiple alternatives, how changing information affects decision processes, and how people reason about complex events. To address these questions, I use computational cognitive modeling to formalize hypotheses and then test these with behavioral experiments.
My proudest accomplishments have been in applying the theories developed in the lab to real-world decision problems. For example, my colleagues and I have been using a combination of machine learning and cognitive modeling to understand why errors occur in medical image-based decision making. In partnership with the pathology department at the Vanderbilt University Medical Center, we’ve discovered that prior information (including irrelevant information) can impact the interpretation of cell images. This work has important implications for AI and automated systems that are being developed to support human medical image classification and decision making.
In another line of research, I’ve been using quantum cognition to provide a new perspective on legal decision making. Even though “quantum probability theory” might sound like a strange thing to apply to human behavior, it is a powerful framework for developing psychological theories because it naturally accommodates contextual and sequential effects.
Lately, I’ve been thinking broadly about how we build better theories of human decision making. Over the past decades, there have been numerous formal theories of decision making proposed. Right now, we don’t have a good sense of how these theories are related. Are there common principles shared by multiple theories? Can ideas from different theories be combined to build better ones? In addition, can we look to advances in machine learning to construct joint cognitive and machine-learning models to further advance theories of human decision making and to build better predictive models of human behavior?
APS regularly opens certain online articles for discussion on our website. Effective February 2021, you must be a logged-in APS member to post comments. By posting a comment, you agree to our Community Guidelines and the display of your profile information, including your name and affiliation. Any opinions, findings, conclusions, or recommendations present in article comments are those of the writers and do not necessarily reflect the views of APS or the article’s author. For more information, please see our Community Guidelines.
Please login with your APS account to comment.