New Content From Perspectives on Psychological Science
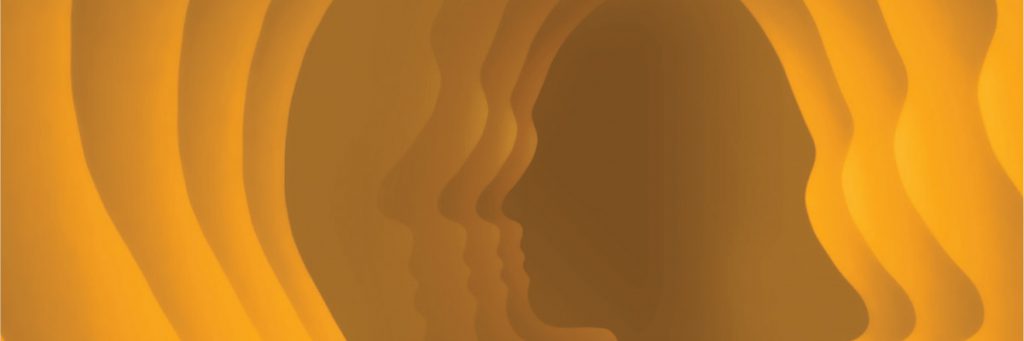
Crowds Can Effectively Identify Misinformation at Scale
Cameron Martel, Jennifer Allen, Gordon Pennycook, and David Rand
Identifying successful approaches for reducing the belief and spread of online misinformation is of great importance. Social media companies currently rely largely on professional fact-checking as their primary mechanism for identifying falsehoods. However, professional fact-checking has notable limitations regarding coverage and speed. In this article, we summarize research suggesting that the “wisdom of crowds” can successfully be harnessed to help identify misinformation at scale. Despite potential concerns about the abilities of laypeople to assess information quality, recent evidence demonstrates that aggregating judgments of groups of laypeople (“crowds”) can effectively identify low-quality news sources and inaccurate news posts: Crowd ratings are strongly correlated with fact-checker ratings across a variety of studies using different designs, stimulus sets, and subject pools. We connect these experimental findings with recent attempts to deploy crowdsourced fact-checking in the field, and close with recommendations and future directions for translating crowdsourced ratings into effective interventions.
Communities of Knowledge in Trouble
Nathaniel Rabb, Mugur Geana, and Steven Sloman
The community-of-knowledge framework explains the extraordinary success of the human species, despite individual members’ demonstrably shallow understanding of many topics, by appealing to outsourcing. People follow the cues of members of their community because understanding of phenomena is generally distributed across the group. Typically communities do possess the relevant knowledge, but it is possible in principle for communities to send cues despite lacking knowledge—a weakness in the system’s design. COVID-19 in the US offered a natural experiment in collective knowledge development because a novel phenomenon arrived at a moment of intense division in political partisanship. We review evidence from the pandemic showing that the thought leaders of the two partisan groups sent radically different messages about COVID, which were in turn reinforced by close community members (family, friends, etc.). We show that while actual understanding of the individual plays a role in a key COVID mitigation behavior (vaccination), it plays a smaller role than perceived understanding of thought leaders and beliefs about COVID-related behaviors of close community members. We discuss implications for theory and practice when all communities are in the same epistemic circumstance—relying on the testimony of others.
An Active-Inference Approach to Second-Person Neuroscience
Konrad Lehmann, Dimitris Bolis, Karl Friston, Leonhard Schilbach, Maxwell Ramstead, and Philipp Kanske
Social neuroscience has often been criticized for approaching the investigation of the neural processes that enable social interaction and cognition from a passive, detached, third-person perspective, without involving any real time social interaction. With the emergence of so- called second-person neuroscience, investigators have evinced the unique complexity of neural activation patterns in actual, real-time interaction. Social cognition that occurs during social interaction is fundamentally different from that unfolding during social observation. However, it remains unclear how the neural correlates of social interaction are to be interpreted. Here, we leverage the active-inference framework to shed light on the mechanisms at play during social interaction in second-person neuroscience studies. Specifically, we show how counterfactually rich mutual predictions, real-time bodily adaptation, and policy selection explain activation in the default mode, salience, and frontoparietal networks of the brain, as well as in the basal ganglia. We further argue that these processes constitute the crucial neural processes that underwrite bona fide social interaction. By placing the experimental approach of second-person neuroscience on the theoretical foundation of the active-inference framework, we inform the field of social neuroscience about the mechanisms of real-life interactions. We thereby contribute to the theoretical foundations of empirical second-person neuroscience.
How the Complexity of Psychological Processes Reframes the Issue of Reproducibility in Psychological Science
Christophe Gernigon, Ruud Den Hartigh, Robin Vallacher, and Paul Van Geert
In the past decade, various recommendations have been published to enhance the methodological rigor and publication standards in psychological science. However, adhering to these recommendations may have limited impact on the reproducibility of causal effects, as long as psychological phenomena continue to be viewed as decomposable into separate and additive statistical structures of causal relationships. In this paper, we show that (a) psychological phenomena are patterns emerging from non-decomposable and non-isolable complex processes that obey idiosyncratic nonlinear dynamics; (b) these processual features jeopardize the chances of standard reproducibility of statistical results; and (c) these features call on researchers to reconsider what can and should be reproduced, namely the psychological processes per se, and the signatures of their complexity and dynamics. Accordingly, we argue for a greater consideration of process causality of psychological phenomena reflected by key properties of complex dynamical systems (CDSs). This implies developing and testing formal models of psychological dynamics, which can be implemented by computer simulation. The scope of the CDS paradigm and its convergences with other paradigms are finally discussed regarding the reproducibility issue. Ironically, the CDS approach could account for both reproducibility and non-reproducibility of the statistical effects usually sought in mainstream psychological science.
Talking About the Absent and the Abstract: Referential Communication in Language and Gesture
Elena Luchkina and Sandra Waxman
Human language permits us to call to mind objects, events, and ideas that we cannot witness directly, either because they are absent or because they have no physical form (e.g., people we have not met, concepts like justice). What enables language to transmit such knowledge? We propose that a referential link between words, referents, and mental representations of those referents is key. This link enables us to form, access, and modify mental representations even when the referents themselves are absent (“absent reference”). In this review we consider the developmental and evolutionary origins of absent reference, integrating previously disparate literatures on absent reference in language and gesture in very young humans and gesture in non-human primates. We first evaluate when and how infants acquire absent reference during the process of language acquisition. With this as a foundation, we consider the evidence for absent reference in gesture in infants and in non-human primates. Finally, having woven these literatures together, we highlight new lines of research that promise to sharpen our understanding of the development of reference and its role in learning about the absent and the abstract.
Incomparability and Incommensurability in Choice: No Common Currency of Value?
Lukasz Walasek and Gordon Brown
Models of decision-making typically assume the existence of some common currency of value, such as utility, happiness, or inclusive fitness. This common currency is taken to allow comparison of options and to underpin everyday choice. Here we suggest instead that there is no universal value scale, that incommensurable values pervade everyday choice, and hence that most existing models of decision-making in both economics and psychology are fundamentally limited. We propose that choice objects can be compared only with reference to specific but nonuniversal “covering values.” These covering values may reflect decision-makers’ goals, motivations, or current states. A complete model of choice must accommodate the range of possible covering values. We show that abandoning the common-currency assumption in models of judgment and decision-making necessitates rank-based and “simple heuristics” models that contrast radically with conventional utility-based approaches. We note that if there is no universal value scale, then Arrow’s impossibility theorem places severe bounds on the rationality of individual decision-making and hence that there is a deep link between the incommensurability of value, inconsistencies in human decision-making, and rank-based coding of value. More generally, incommensurability raises the question of whether it will ever be possible to develop single-quantity-maximizing models of decision-making.
Personality Science in the Digital Age: The Promises and Challenges of Psychological Targeting for Personalized Behavior Change Interventions at Scale
Sandra Matz, Emorie Beck, Olivia Atherton, Mike White, John Rauthmann, Dan Mroczek, Minhee Kim, and Tim Bogg
With the rapidly growing availability of scalable psychological assessments, personality science holds great promise for the scientific study and applied use of customized behavior-change interventions. To facilitate this development, we propose a classification system that divides psychological targeting into two approaches that differ in the process by which interventions are designed: audience-to-content matching or content-to-audience matching. This system is both integrative and generative: It allows us to (a) integrate existing research on personalized interventions from different psychological subdisciplines (e.g., political, educational, organizational, consumer, and clinical and health psychology) and to (b) articulate open questions that generate promising new avenues for future research. Our objective is to infuse personality science into intervention research and encourage cross-disciplinary collaborations within and outside of psychology. To ensure the development of personality-customized interventions aligns with the broader interests of individuals (and society at large), we also address important ethical considerations for the use of psychological targeting (e.g., privacy, self-determination, and equity) and offer concrete guidelines for researchers and practitioners.
A Critical Perspective on Neural Mechanisms in Cognitive Neuroscience: Towards Unification
Sander van Bree
A central pursuit of cognitive neuroscience is to find neural mechanisms of cognition, with research programs favoring different strategies to look for them. But what is a neural mechanism, and how do we know we have captured them? Here I answer these questions through a framework that integrates Marr’s levels with philosophical work on mechanism. From this, the following goal emerges: What needs to be explained are the computations of cognition, with explanation itself given by mechanism—composed of algorithms and parts of the brain that realize them. This reveals a delineation within cognitive neuroscience research. In the premechanism stage, the computations of cognition are linked to phenomena in the brain, narrowing down where and when mechanisms are situated in space and time. In the mechanism stage, it is established how computation emerges from organized interactions between parts—filling the premechanistic mold. I explain why a shift toward mechanistic modeling helps us meet our aims while outlining a road map for doing so. Finally, I argue that the explanatory scope of neural mechanisms can be approximated by effect sizes collected across studies, not just conceptual analysis. Together, these points synthesize a mechanistic agenda that allows subfields to connect at the level of theory.
Discrepancies in the Definition and Measurement of Human Interoception: A Comprehensive Discussion and Suggested Ways Forward
Olivier Desmedt, Olivier Luminet, Pierre Maurage, and Olivier Corneille
Interoception has been the object of renewed interest over the past two decades. The involvement of interoception in a diverse range of fundamental human abilities (e.g., decision-making and emotional regulation) has led to the hypothesis that interoception is a central transdiagnostic process causing and maintaining mental disorders as well as physical diseases. However, interoception has been inconsistently defined and conceptualized. In the first part of this article, we argue the widespread practice of defining interoception as the processing of signals originating from within the body and limiting it to specific physiological pathways (lamina I spinothalamic afferents) is problematic. This is because, in humans, the processing of internal states is underpinned by other physiological pathways generally assigned to the somatosensory system. In the second part, we explain that the consensual dimensions of interoception are empirically detached from existing measures, the latter of which capture loosely related phenomena. This is detrimental to the replicability of findings across measures and the validity of interpretations. In the general discussion, we discuss the main insights of the current analysis and suggest a more refined way to define interoception in humans and conceptualize its underlying dimensions.
Understanding Collective Intelligence: Investigating the Role of Collective Memory, Attention, and Reasoning Processes
Anita Woolley and Pranav Gupta
As society has come to rely on groups and technology to address many of its most challenging problems, there is a growing need to understand how technology-enabled, distributed, and dynamic collectives can be designed to solve a wide range of problems over time in the face of complex and changing environmental conditions—an ability we define as “collective intelligence.” We describe recent research on the Transaction Systems Model of Collective Intelligence (TSM-CI) that integrates literature from diverse areas of psychology to conceptualize the underpinnings of collective intelligence. The TSM-CI articulates the development and mutual adaptation of transactive memory, transactive attention, and transactive reasoning systems that together support the emergence and maintenance of collective intelligence. We also review related research on computational indicators of transactive-system functioning based on collaborative process behaviors that enable agent-based teammates to diagnose and potentially intervene to address developing issues. We conclude by discussing future directions in developing the TSM-CI to support research on developing collective human-machine intelligence and to identify ways to design technology to enhance it.
Human and Algorithmic Predictions in Geopolitical Forecasting: Quantifying Uncertainty in Hard-to-Quantify Domains
Barbara Mellers, John McCoy, Louise Lu, and Philip Tetlock
Research on clinical versus statistical prediction has demonstrated that algorithms make more accurate predictions than humans in many domains. Geopolitical forecasting is an algorithm-unfriendly domain, with hard-to-quantify data and elusive reference classes that make predictive model-building difficult. Furthermore, the stakes can be high, with missed forecasts leading to mass-casualty consequences. For these reasons, geopolitical forecasting is typically done by humans, even though algorithms play important roles. They are essential as aggregators of crowd wisdom, as frameworks to partition human forecasting variance, and as inputs to hybrid forecasting models. Algorithms are extremely important in this domain. We doubt that humans will relinquish control to algorithms anytime soon—nor do we think they should. However, the accuracy of forecasts will greatly improve if humans are aided by algorithms.
Blinding to Circumvent Human Biases: Deliberate Ignorance in Humans, Institutions, and Machines
Ralph Hertwig, Stefan Herzog, and Anastasia Kozyreva
Inequalities and injustices are thorny issues in liberal societies, manifesting in forms such as the gender–pay gap; sentencing discrepancies among Black, Hispanic, and White defendants; and unequal medical-resource distribution across ethnicities. One cause of these inequalities is implicit social bias—unconsciously formed associations between social groups and attributions such as “nurturing,” “lazy,” or “uneducated.” One strategy to counteract implicit and explicit human biases is delegating crucial decisions, such as how to allocate benefits, resources, or opportunities, to algorithms. Algorithms, however, are not necessarily impartial and objective. Although they can detect and mitigate human biases, they can also perpetuate and even amplify existing inequalities and injustices. We explore how a philosophical thought experiment, Rawls’s “veil of ignorance,” and a psychological phenomenon, deliberate ignorance, can help shield individuals, institutions, and algorithms from biases. We discuss the benefits and drawbacks of methods for shielding human and artificial decision makers from potentially biasing information. We then broaden our discussion beyond the issues of bias and fairness and turn to a research agenda aimed at improving human judgment accuracy with the assistance of algorithms that conceal information that has the potential to undermine performance. Finally, we propose interdisciplinary research questions.
A Cognitive Computational Approach to Social and Collective Decision-Making
Alan Tump, Dominik Deffner, Tim Pleskac, Pawel Romanczuk, and Ralf Kurvers
Collective dynamics play a key role in everyday decision-making. Whether social influence promotes the spread of accurate information and ultimately results in adaptive behavior or leads to false information cascades and maladaptive social contagion strongly depends on the cognitive mechanisms underlying social interactions. Here we argue that cognitive modeling, in tandem with experiments that allow collective dynamics to emerge, can mechanistically link cognitive processes at the individual and collective levels. We illustrate the strength of this cognitive computational approach with two highly successful cognitive models that have been applied to interactive group experiments: evidence-accumulation and reinforcement-learning models. We show how these approaches make it possible to simultaneously study (a) how individual cognition drives social systems, (b) how social systems drive individual cognition, and (c) the dynamic feedback processes between the two layers.
Body as First Teacher: The Role of Rhythmic Visceral Dynamics in Early Cognitive Development
Andrew Corcoran, Kelsey Perrykkad, Daniel Feuerriegel, and Jonathan Robinson
Embodied cognition—the idea that mental states and processes should be understood in relation to one’s bodily constitution and interactions with the world—remains a controversial topic within cognitive science. Recently, however, increasing interest in predictive processing theories among proponents and critics of embodiment alike has raised hopes of a reconciliation. This article sets out to appraise the unificatory potential of predictive processing, focusing in particular on embodied formulations of active inference. Our analysis suggests that most active-inference accounts invoke weak, potentially trivial conceptions of embodiment; those making stronger claims do so independently of the theoretical commitments of the active-inference framework. We argue that a more compelling version of embodied active inference can be motivated by adopting a diachronic perspective on the way rhythmic physiological activity shapes neural development in utero. According to this visceral afferent training hypothesis, early-emerging physiological processes are essential not only for supporting the biophysical development of neural structures but also for configuring the cognitive architecture those structures entail. Focusing in particular on the cardiovascular system, we propose three candidate mechanisms through which visceral afferent training might operate: (a) activity-dependent neuronal development, (b) periodic signal modeling, and (c) oscillatory network coordination.
Reference-Point Theory: An Account of Individual Differences in Risk Preferences
Barbara Mellers and Siyuan Yin
We propose an account of individual differences in risk preferences called “reference-point theory” for choices between sure things and gambles. Like most descriptive theories of risky choice, preferences depend on two drivers—hedonic sensitivities to change and beliefs about risk. But unlike most theories, these drivers are estimated from judged feelings about choice options and gamble outcomes. Furthermore, the reference point is assumed to be the less risky option (i.e., sure thing). Loss aversion (greater impact of negative change than positive change) and pessimism (belief the worst outcome is likelier) predict risk aversion. Gain seeking (greater impact of positive change than negative change and optimism (belief the best outcome is likelier) predict risk seeking. But other combinations of hedonic sensitivities and beliefs are possible, and they also predict risk preferences. Finally, feelings about the reference point predict hedonic sensitivities. When decision makers feel good about the reference point, they are frequently loss averse. When they feel bad about it, they are often gain seeking. Three studies show that feelings about reference points, feelings about options and feelings about outcomes predict risky choice and help explain why individuals differ in their risk preferences.
Feedback on this article? Email [email protected] or login to comment.
APS regularly opens certain online articles for discussion on our website. Effective February 2021, you must be a logged-in APS member to post comments. By posting a comment, you agree to our Community Guidelines and the display of your profile information, including your name and affiliation. Any opinions, findings, conclusions, or recommendations present in article comments are those of the writers and do not necessarily reflect the views of APS or the article’s author. For more information, please see our Community Guidelines.
Please login with your APS account to comment.