New Content from Advances in Methods and Practices in Psychological Science
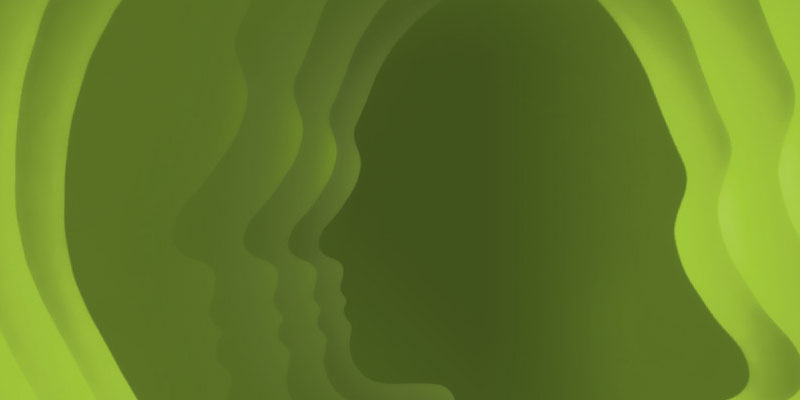
Your Coefficient Alpha Is Probably Wrong, but Which Coefficient Omega Is Right? A Tutorial on Using R to Obtain Better Reliability Estimates
David B. Flora
In this tutorial, Flora describes alternative forms of the coefficient omega—an alternative to the coefficient alpha, for conveying reliability estimates—and provides guidelines for choosing the appropriate omega estimates. He shows several examples and demonstrates how to perform omega calculations using R. The different forms of coefficient omega are reliability estimates calculated from models that represent associations between a test’s items and the construct the test is intended to measure. This coefficient appears to reflect reliability better than alpha coefficients, which depend on restricted and unrealistic psychometric models.
Visualization of Brain Statistics With R Packages ggseg and ggseg3d
Athanasia M. Mowinckel and Didac Vidal-Piñeiro
In this tutorial, Mowinckel and Vidal-Piñeiro present two packages for the statistical software R that integrate a spatial component in data visualization, inherent in neuroimaging data. However, this spatial component is lost in common statistical representations, such as bar charts. These packages visualize predefined brain segmentations in 2D polygons and 3D meshes and are integrated with other R packages. The researchers describe the main data and functions in these packages. Mowinckel and Vidal-Piñeiro suggest that these tools may improve and facilitate the dissemination of neuroimaging results.
Measurement Schmeasurement: Questionable Measurement Practices and How to Avoid Them
Jessica Kay Flake and Eiko I. Fried
Flake and Fried define questionable measurement practices that jeopardize the validity of measures and study results. They also offer practical actions to avoid these practices, arguing for the transparency of measurement decisions. Reporting the following information may help to ensure transparency about measurement practices: construct definition and its theoretical/empirical support; justification for the measure selection; existing validity evidence; measure and administration procedure; response coding and transformation; detailed score calculation; all psychometric analyses; detailed descriptions of measurement modifications; and creation of any new measures and their detailed description and justification.
A Traveler’s Guide to the Multiverse: Promises, Pitfalls, and a Framework for the Evaluation of Analytic Decisions
Marco Del Giudice and Steven W. Gangestad
Multiverse methods (e.g., specification curve, vibration of effects) estimate an effect across an entire set of possible analytical specifications to expose the impact of hidden degrees of freedom and/or obtain less-biased estimates of the effect being studied. However, when the specifications are not arbitrary but treated as such, they can inflate the size of the multiverse, exaggerating the perceived exhaustiveness of the multiverse while making it difficult to extract relevant findings. Del Giudice and Gangestad offer a framework and conceptual tools that will help researchers make the best use of multiverse-style methods. They illustrate the framework with a simulated data set and published examples.
Getting Started Creating Data Dictionaries: How to Create a Shareable Data Set
Erin M. Buchanan et al.
In this tutorial, Buchanan and colleagues provide a guide for creating data dictionaries and codebooks to accompany data repositories shared with other researchers (e.g., in OSF). Data dictionaries and codebooks provide metadata, including information about variables and data collection, that can help other researchers understand a dataset. Metadata can facilitate search-engine indexing of a dataset and provide insights about how the data might be used in future research. The authors explain relevant terminology and format standards, show how to use the codebook (for R) and Data Dictionary Creator applications, discuss other available applications, and provide accompanying information on OSF.
Making the Black Box Transparent: A Template and Tutorial for Registration of Studies Using Experience-Sampling Methods
Olivia J. Kirtley, Ginette Lafit, Robin Achterhof, Anu P. Hiekkaranta, and Inez Myin-Germeys
The experience-sampling method (ESM) consists of having participants complete brief questionnaires one or more times per day, usually via a smartphone app, to give momentary reports about their thoughts, behaviors, emotions, and contexts. Kirtley and colleagues discuss ways in which ESM is vulnerable to threats to transparency, reproducibility, and replicability, and they propose that study preregistration may address some of these threats. They also discuss ways to select models, account for potential model-convergence issues, use preexisting data sets, and document these sets in preregistration. Kirtley and colleagues also provide a registration template for ESM.
Assessing Change in Intervention Research: The Benefits of Composite Outcomes
David Moreau and Kristina Wiebels
Combining assessments might be a better way to evaluate the effectiveness of interventions than relying on individual measures, recommend Moreau and Wiebels. They argue that composite scores that pool information from single measures into a single outcome can provide better estimates of the underlying constructs of interest while retaining interpretability. The researchers describe different methods to compute, evaluate, and use composite assessments depending on different goals, experimental design, and data. They provide a preregistration template with examples of psychological interventions and the accompanying R code. They also provide a Shiny app and R code, available at https://osf.io/u96em/.
Analyzing Individual Differences in Intervention-Related Changes
Tanja Könen and Julia Karbach
Könen and Karbach discuss the benefits and limitations of analyzing individual differences in intervention studies in addition to analyzing only group effects. They suggest that analyzing individual differences cannot replace group analyses and provides only correlational and descriptive information about individuals and interventions. Individual-differences analyses can, however, enhance the future implementation of interventions. They also discuss methods for analyzing individual differences and give three examples of latent change models as a framework for analyzing individual differences in interventions.
APS regularly opens certain online articles for discussion on our website. Effective February 2021, you must be a logged-in APS member to post comments. By posting a comment, you agree to our Community Guidelines and the display of your profile information, including your name and affiliation. Any opinions, findings, conclusions, or recommendations present in article comments are those of the writers and do not necessarily reflect the views of APS or the article’s author. For more information, please see our Community Guidelines.
Please login with your APS account to comment.