New Content from Advances in Methods and Practices in Psychological Science
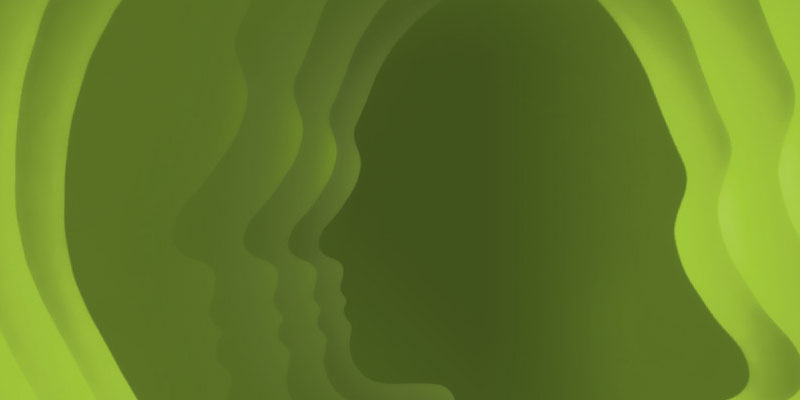
Quantifying Sources of Variability in Infancy Research Using the Infant-Directed-Speech Preference
The ManyBabies Consortium
This large-scale, multisite study assessed the replicability and variables that affect infants’ preference for infant-directed speech (IDS) over adult-directed speech (ADS). Across laboratories in several countries, the researchers presented recordings of women speaking in English to their infants and to adults and measured whether the infants preferred IDS over ADS, as indicated by head-turn preference or eye tracking. Overall, infants appeared to prefer IDS, although this effect was smaller than previous research had indicated. Also, infants preferred IDS more strongly when they were older (i.e., close to 12 months), when the IDS matched their native language, and when the measure used was a head turn.
Hidden Invalidity Among 15 Commonly Used Measures in Social and Personality Psychology
Ian Hussey and Sean Hughes
Hussey and Hughes investigated the psychometric properties of 15 questionnaires and 26 scales widely used in social and personality psychology. The internal consistency of these self-report measures indicated that 89% appeared to have good validity (i.e., appeared to measured what they intended to measure). However, when the researchers considered test-retest reliability, factor structure, and measurement invariance for age and gender groups, only 4% of the measures demonstrated good validity. Scales were more likely to fail tests that were underreported in the literature, which may represent widespread hidden invalidity of the measures used. The authors also introduce the concept of validity hacking (v-hacking).
StatBreak: Identifying “Lucky” Data Points Through Genetic Algorithms
Hannes Rosenbusch, Leon P. Hilbert, Anthony M. Evans, and Marcel Zeelenberg
Rosenbusch and colleagues present StatBreak, a method that allows researchers to identify the data points that most strongly contributed to a finding (e.g., effect size, model fit, p value, Bayes factor). This is useful because some interesting findings are produced by just a small number of “lucky” data points, and StatBreak identifies which and how many of these “lucky” data points would need to be excluded from the sample for the finding to be different. The authors demonstrate how StatBreak works with real and simulated data, across different designs and statistics, and present the StatBreak package for R.
Cross-Validation: A Method Every Psychologist Should Know
Mark de Rooij and Wouter Weeda
Cross-validation assesses how accurate a model’s predictions might be for another independent data set. The researchers introduce an R package to conduct cross-validation and present examples illustrating the use of this package for different types of problems. They suggest that although most researchers might be familiar with this procedure, they seldom use it to analyze their data. Yet it might be an easy-to-use alternative to the common null-hypothesis testing, with the benefit of not requiring the researcher to make as many assumptions.
Analysis of Open Data and Computational Reproducibility in Registered Reports in Psychology
Pepijn Obels, Daniël Lakens, Nicholas A. Coles, Jaroslav Gottfried, and Seth A. Green
Obels and colleagues examined data and code sharing for Registered Reports published between 2014 and 2018 and attempted to computationally reproduce their main results. Of the 62 articles analyzed, they found that 41 had data available, 37 had analysis scripts available, and 36 had both data and analysis scripts available. The researchers were able to reproduce the results of 21 articles. These findings suggest that there is room for improvement regarding the sharing of data, materials, and analysis scripts. Obels and colleagues provide recommendations for good research practices based on the studies whose main results they reproduced.
Average Power: A Cautionary Note
Blakeley B. McShane, Ulf Böckenholt, and Karsten T. Hansen
McShane and colleagues clarify the nature of average power, a measure that quantifies the power of a set of previous studies using a meta-analytic approach. They explain that average power is not relevant to the replicability of prospective replication studies. The researchers suggest that point estimates of average power are too variable and inaccurate and that interval estimates of average power depend on point estimates, rendering both estimates difficult to use in application. These findings do not imply that meta-analyses are not useful, especially when used to calculate variation in effect sizes rather than average power.
APS regularly opens certain online articles for discussion on our website. Effective February 2021, you must be a logged-in APS member to post comments. By posting a comment, you agree to our Community Guidelines and the display of your profile information, including your name and affiliation. Any opinions, findings, conclusions, or recommendations present in article comments are those of the writers and do not necessarily reflect the views of APS or the article’s author. For more information, please see our Community Guidelines.
Please login with your APS account to comment.