Featured
Do We Want to Be Credible or Incredible?
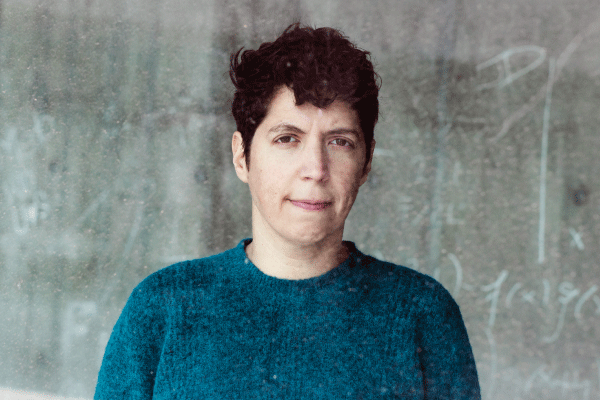
Psychological science is at a crossroads. The replicability crisis has turned into a credibility revolution. Many professional societies and journals, including APS, have raised their expectations for transparency and rigor. These early changes are heartening and signal our field’s commitment to earning credibility. Those first steps, however, were the easy part.
The challenge facing psychology now is this: Do we want to be credible or incredible? Up until now, we have been able to make important strides in increasing transparency and rigor without giving up much positive attention from the public. Alongside occasional failures to replicate and high-profile retractions, most of the literature continues to make bold claims of groundbreaking discoveries based on research that receives little scrutiny — often because what we’d need to scrutinize it (e.g., data, code, materials, preregistered plan) isn’t available. The bit of scrutiny to which we do subject claims, by way of formal peer review, is a black box, and only reviewers, authors, and editors get to look inside. This makes it easy to publish and promote incredible effects — headlines that reach the general public and provide a fleeting moment of positive press for our field — that are likely to shrink or disappear if submitted to scrutiny.
There is an inherent tension between being incredible and being credible. To be incredible, we have to keep producing eye-catching results at a fast pace. This is easier to do if we don’t provide a lot of details. As Buckheit and Donoho (1995) famously wrote, “An article . . . in a scientific publication is not the scholarship itself, it is merely advertising for the scholarship” (p. 5). In this advertisement-only model of science, it’s easier to convince ourselves, and others, of incredible results. If no one is going to look under the hood (check reproducibility) or take the claim out for a test drive (check replicability), extravagant claims will thrive (Vazire, 2017). To be transparent is to give our critics ammunition — to share any information about the research process, within legal and ethical constraints, that others might use to identify flaws or errors. What survives that scrutiny is likely to be more credible, but less exciting, than in the advertisement-only model.
It’s tempting to try to have it all. After all, why not support and encourage incremental improvements in our methods and practices while also celebrating discoveries that follow from the old style of research? Why not let a thousand flowers bloom? Let everyone choose their preferred standards and practices, and see what happens.
The first problem, as we have been made painfully aware, is that this can lead to a proliferation of false-positive results (Camerer et al., 2018; Ebersole, Axt, & Nosek, 2016; Hagger et al., 2016; Klein et al., 2014, 2018; O’Donnell et al., 2018; Open Science Collaboration, 2015; Wagenmakers et al., 2016).
A second problem is that it is very easy to predict what will happen if we let a thousand flowers bloom (and these predictions have been validated by formal models; Smaldino & McElreath, 2016). This doesn’t look good for transparency and quality control. If there are no negative consequences for being less transparent, for opting out of scrutiny and accountability, those who opt out will be able to take shortcuts, make bolder claims, and get more rewards. The more transparent researchers whose errors get caught and corrected will lose the race for jobs, tenure, grants, and prizes.
In a system in which we frequently have to compare research outputs across candidates (e.g., for jobs, awards, grants), how should we compare the researcher who transparently reports all analyses, studies, and results and therefore has a messy or boring story to tell with the researcher who tells us that they have a strong and compelling set of results but doesn’t give us the information we’d need to verify this claim? If we give the second researcher the benefit of the doubt, we are de facto punishing the first researcher. In a system in which opacity is the acceptable default, there is no way to survive as a transparent researcher.
Of course, we should not assume that transparent research is good research. But we don’t have to — that’s the point of transparency. Transparency is for scrutiny, critique, and correction. We shouldn’t assume transparent research is rigorous, we should evaluate whether it is. Transparency doesn’t guarantee credibility; transparency and scrutiny together guarantee that research gets the credibility it deserves. When research isn’t transparent, we should refuse to ascribe to it any particular level of credibility — letting nontransparent research enter the competition ensures that transparent research will get crowded out.
Another problem with the live-and-let-live approach is that it ignores our responsibility to the public. At least in the United States (Funk, Hefferon, Kennedy, & Johnson, 2019), the public has a great deal of trust in science, but the same survey also suggests that the public doesn’t trust individual scientists and expects us to hold each other accountable. Take, for example, the very low proportion of respondents who said they trust medical, nutrition, and environmental scientists to “admit and take responsibility for mistakes” (13%, 11%, and 16%, respectively) or to “provide fair and accurate information” (32%, 24%, and 35%).
How is it possible that almost 9 out of 10 Americans do not agree that medical researchers admit and take responsibility for their mistakes, yet 86% trust science? One clue is the finding, from the same Pew survey, that 57% of Americans say they would trust research more when the data are openly available (vs. 8% who say they would trust it less and 34% who say it makes no difference). The public doesn’t trust us as individuals, but they trust science because of the expectation of transparency and accountability. If we continue to make transparency and quality control optional — which we effectively do when we continue to give our seal of approval (and put out press releases) for research that is not transparent and has not passed through careful scrutiny — we are putting our long-term credibility in jeopardy. We may score more points in the short term by putting out more frequent and dramatic headlines, but we risk losing credibility in the long term when the public realizes we don’t make transparency and verification requirements for endorsing such claims.
I understand the appeal of using carrots and not sticks. It’s unpleasant to punish researchers who sincerely believe that their practices are rigorous. But we now know that practices that we believed were rigorous turned out to be error-prone; we now know that we need more than a “trust me” from the researcher, however sincere they are. Researchers should not be able to exempt themselves from outside scrutiny — as psychologists, we should know better than anyone the risks of self-deception. Given that we now know that transparent reporting is vital for catching and correcting errors, the public won’t (and shouldn’t) be sympathetic if we want to let every researcher choose their own level of transparency, simply because we don’t want to step on anyone’s toes.
There are still many details to work out. What kind of transparency is most important for detecting and correcting errors? What if we make things more transparent, but no one wants to do the thankless work of checking for errors? As Vonnegut said, “everyone wants to build, nobody wants to do maintenance” (1997, p. 167). Which kinds of errors should most concern us? These are questions for methodologists and metascientists to figure out, with help from experts in sociology, history, and philosophy of science.
But we cannot wait until these details are settled to decide how serious we are about our commitment to credibility. Are we willing to reserve bold claims of discovery for findings that are transparently reported and withstand the scrutiny and verification that transparency invites? Are we willing to forego the positive attention we get from media coverage of claims that were never put to a severe test? It will be painful at first, but the knowledge we’ll produce in the long run will be better than incredible — it’ll be credible.
References
Buckheit, J. B., & Donoho, D. L. (1995). WaveLab and reproducible research. In A. Antoniadis & G. Oppenheim G. (Eds.), Wavelets and Statistics. New York, NY: Springer. https://doi.org/10.1007/978-1-4612-2544-7_5
Camerer, C. F., Dreber, A., Holzmeister, F., Ho, T.-H., Huber, J., Johannesson, M., . . . Wu, H. (2018). Evaluating the replicability of social science experiments in Nature and Science between 2010 and 2015. Nature Human Behaviour, 2, , 637–644., https://doi.org/10.1038/s41562-018-0399-z
Ebersole, C. R., Axt, J. R., & Nosek, B. A. (2016). Scientists’ reputations are based on getting it right, not being right. PLOS Biology, 14(5), Article e1002460. https://doi.org/10.1371/journal.pbio.1002460
Funk, C., Hefferon, M., Kennedy, B., & Johnson, C. (2019 August). Trust and mistrust in Americans’ views of scientific experts. Pew Research Center. Retrieved from https://www.pewresearch.org/science/2019/08/02/trust-and-mistrust-in-americans-views-of-scientific-experts/
Hagger, M. S., Chatzisarantis, N. L. D., Alberts, H., Anggono, C. O., Batailler, C., Birt, A. R., . . . Zwienenberg, M. (2016). A multilab preregistered replication of the ego-depletion effect. Perspectives on Psychological Science, 11, 546–573. https://doi.org/10.1177/1745691616652873
Klein, R. A., Ratliff, K. A., Vianello, M., Adams, R. B., Jr., Bahník, S., Bernstein, M. J., . . . Nosek, B. A. (2014) Investigating variation in replicability: A “many labs” replication project. Social Psychology, 45, 142–152. https://doi.org/10.1027/1864-9335/a000178
Klein, R. A., Vianello, M., Hasselman, F., Adams, B. G., Adams, R. B., Alper, S., . . . Nosek, B. A. (2018). Many Labs 2: Investigating variation in replicability across samples and settings. Advances in Methods and Practices in Psychological Science, 1, 443–490. https://doi.org/10.1177/2515245918810225
O’Donnell, M., Nelson, L. D., Ackermann, E., Aczel, B., Akhtar, A., Aldrovandi, S., . . . Zrubka, M. (2018). Registered Replication Report: Dijksterhuis and van Knippenberg (1998). Perspectives on Psychological Science, 13, 268–294. https://doi.org/10.1177/1745691618755704
Open Science Collaboration. (2015). Estimating the reproducibility of psychological science. Science, 349(6251), Article 4716. https://doi.org/10.1126/science.aac4716
Smaldino, P. E., & McElreath, R. (2016). The natural selection of bad science. Royal Society Open Science, 3, Article 160384. https://doi.org/10.1098/rsos.160384
Vazire, S. (2017). Quality uncertainty erodes trust in science. Collabra: Psychology, 3(1), 1. http://doi.org/10.1525/collabra.74
Vonnegut, K. (1997). Hocus pocus. New York, NY: Penguin.
Wagenmakers, E.-J., Beek, T., Dijkhoff, L., Gronau, Q. F., Acosta, A., Adams, R. B., . . . Zwaan, R. A. (2016). Registered Replication Report: Strack, Martin, & Stepper (1988). Perspectives on Psychological Science, 11, 917–928. https://doi.org/10.1177/1745691616674458
Comments
“Do we want to be credible or incredible?”
We can talk about it, but as scientists we know that it is only useful to talk about things that can be measured.
Fortunately, we can measure incredibility and replicability without asking for data or other materials.
Using this approach, I have examined the credibility of results in over 100 journals and I was able to show that the journal Psychological Science has improved during the 2010s under the leadership of Stephen Lindsay.
https://replicationindex.com/2018/12/29/2018-replicability-rankings/
APS regularly opens certain online articles for discussion on our website. Effective February 2021, you must be a logged-in APS member to post comments. By posting a comment, you agree to our Community Guidelines and the display of your profile information, including your name and affiliation. Any opinions, findings, conclusions, or recommendations present in article comments are those of the writers and do not necessarily reflect the views of APS or the article’s author. For more information, please see our Community Guidelines.
Please login with your APS account to comment.