New Content from Advances in Methods and Practices in Psychological Science
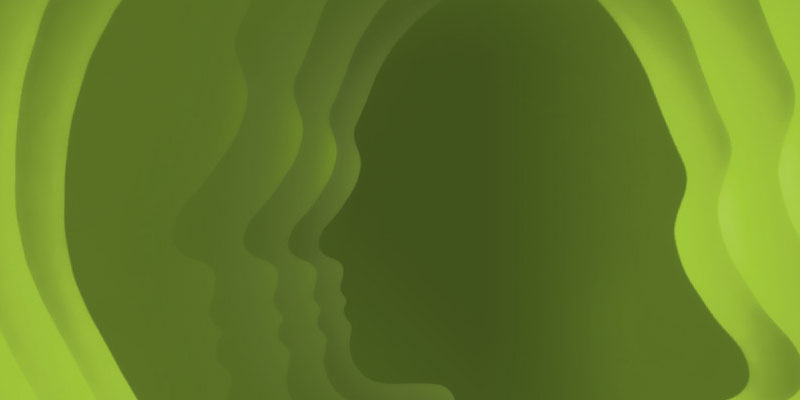
A Conceptual Introduction to Bayesian Model Averaging
Max Hinne, Quentin F. Gronau, Don van den Bergh, and Eric-Jan Wagenmakers
Bayesian model averaging (BMA) is a statistical approach that learns the parameters for all models that could describe the data-generating process and combines the parameter estimates according to each model’s posterior probabilities (i.e., the researcher’s updated evaluation of the model plausibility after seeing the data). Hinne and colleagues explain BMA and its advantages over the usual approach of selecting a model according to certain criteria and learning about the parameters of only that specific model. They also provide examples of how to use BMA in analysis of covariance designs, meta-analysis, and network analysis.
Crud (Re)Defined
Amy Orben and Daniël Lakens
With the increasing availability of large data sets in psychological science, the “crud factor”—the idea that everything correlates with everything else—is experiencing a renaissance. Orben and Lakens review the history of the crud factor and its development and use throughout the history of psychological science. The authors acknowledge a common lack of understanding about what the crud factor is and discuss whether it can be proven to exist or can be estimated and how it should be interpreted. Orben and Lakens clarify this concept, hoping it can be taken more seriously to improve psychological science.
Registered Replication Report on Fischer, Castel, Dodd, and Pratt (2003)
Lincoln J. Colling, Dénes Szűcs, Damiano De Marco, et al.
Fischer et al. (2003) found that participants are quicker to detect a visual target (e.g., a white circle) on the left side of a screen when they first see a small number (1 or 2) at the center and that they are quicker to detect a target on the right when they first see a large number (8 or 9) at the center. Colling and colleagues failed to replicate this attentional spatial-numerical association of response codes (Att-SNARC) effect. These findings suggest that researchers should not use the Att-SNARC effect as evidence that large numbers automatically direct attention to the right and small numbers to the left.
The Unbearable Lightness of Attentional Cuing by Symbolic Magnitude: Commentary on the Registered Replication Report by Colling et al.
Martin H. Fischer, Michael D. Dodd, Alan D. Castel, and Jay Pratt
Colling et al. (2020) failed to replicate the Att-SNARC effect (i.e., participants are quicker to detect a visual target on the left side of a screen when it is preceded by a small number and quicker to detect a target on the right when it is preceded by a large number). In this commentary, Fischer and colleagues highlight that the Att-SNARC has been replicated in other studies and describe possible issues with the attempted replication, including a failure to account for some possible moderators of the results (e.g., response speed, gaming experience).
Rock the MIC: The Matrix of Implied Causation, a Tool for Experimental Design and Model Checking
Timothy R. Brick and Drew H. Bailey
Brick and Bailey introduce the matrix of implied causation (MIC) as a tool for easily understanding and reporting a model’s implications for the causal relationships between variables. MICs can help to directly examine causal implications in path modeling, including structural equation modeling. The authors use examples from the literature to illustrate the use of MICs in model checking and experimental design and argue that when researchers use models with causal implications, they should also use MICs to help to differentiate among models with otherwise similar fit.
APS regularly opens certain online articles for discussion on our website. Effective February 2021, you must be a logged-in APS member to post comments. By posting a comment, you agree to our Community Guidelines and the display of your profile information, including your name and affiliation. Any opinions, findings, conclusions, or recommendations present in article comments are those of the writers and do not necessarily reflect the views of APS or the article’s author. For more information, please see our Community Guidelines.
Please login with your APS account to comment.