New Content From Advances in Methods and Practices in Psychological Science
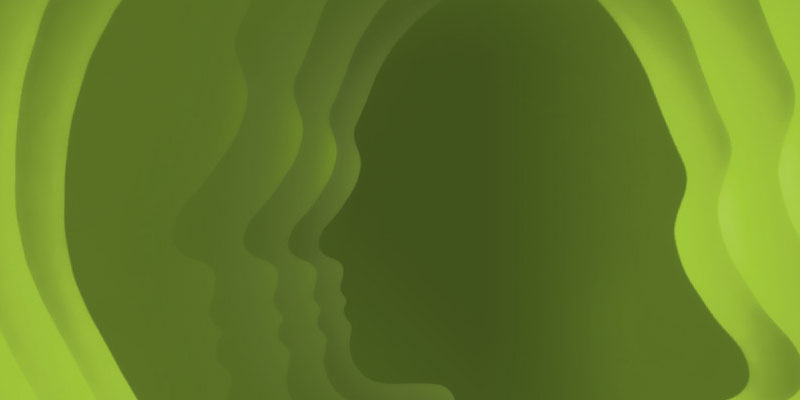
iCatcher+: Robust and Automated Annotation of Infants’ and Young Children’s Gaze Behavior From Videos Collected in Laboratory, Field, and Online Studies
Yotam Erel et al.
Erel and colleagues build on a system for automatic gaze annotation in young children, iCatcher, by engineering improvements and then training and testing the improved system—iCatcher+. When trained on three data sets (videos of children aged 4 months–3.5 years, collected in labs and field settings, in the U.S. and Senegal), iCatcher+ performed with near human-level accuracy on held-out videos on distinguishing “LEFT” versus “RIGHT” and “ON” versus “OFF” looking behavior. The system achieved this high performance at the level of individual frames, experimental trials, and study videos. The performance also held across participant demographics (e.g., age, race/ethnicity), participant behavior (e.g., movement, head position), and video characteristics (e.g., luminance), as well as generalized to a different online data set.
PsyCalibrator: An Open-Source Package for Display Gamma Calibration and Luminance and Color Measurement
Zhicheng Lin, Qi Ma, and Yang Zhang
The appearance of visual stimuli on digital screens depends on properties such as luminance and color, making it critical to measure them. In this tutorial, Lin and colleagues present an open-source integrated software package—PsyCalibrator—that uses consumer hardware (SpyderX, Spyder5) to make luminance/color measurement and gamma calibration easily accessible. Validation of PsyCalibrator indicates (a) excellent accuracy in linear correction and luminance/color measurement and (b) low measurement variances. The authors offer a detailed tutorial on using PsyCalibrator and recommend reporting templates to describe visual stimuli that are simple (e.g., computer-generated shapes) as well as complex (e.g., naturalistic images and videos).
When to Use Different Inferential Methods for Power Analysis and Data Analysis for Between-Subjects Mediation
Jessica L. Fossum and Amanda K. Montoya
Fossum and Montoya explore the similarity of power estimates from six inferential methods for between-subjects mediation when the samples are the same size. They found that when data meet the assumptions of linear regression, the joint significance test, the Monte Carlo confidence interval, and the percentile bootstrap confidence interval perform similarly. When the assumptions are violated, the nonbootstrapping methods tended to have vastly different power estimates compared with the bootstrapping methods. Thus, the researchers recommend using the joint significance test for power analysis only when no assumption violations are hypothesized, and using the percentile bootstrap confidence interval when assumption violations are suspected.
Selecting the Number and Labels of Topics in Topic Modeling: A Tutorial
Sara J. Weston, Ian Shryock, Ryan Light, and Phillip A. Fisher
Weston and colleagues describe tools researchers can use to identify the number and labels of topics in topic modeling—a type of text analysis that identifies clusters of co-occurring words, or latent topics. The authors outline the procedure for narrowing down a large range of models to a select number by comparing them on fit metrics, including exclusivity, residuals, variational lower bound, and semantic coherence. Weston and colleagues also describe tools for labeling topics, including frequent and exclusive words, key examples, and correlations among topics.
Beyond the Mean: Can We Improve the Predictive Power of Psychometric Scales?
Yngwie Asbjørn Nielsen, Isabel Thielmann, and Stefan Pfattheicher
Reliance on the mean score of psychometric scales might be justifiable, this research indicates, but it is also important to explore the predictive power of other summary statistics. Nielsen and colleagues explored whether some seldom-used summary statistics of a psychometric scale (e.g., the standard deviation, the median, or the kurtosis) can improve the prediction of certain outcome measures: life satisfaction, mental health, self-esteem, work behavior, and social value orientation. Across 32 psychometric scales and three data sets, they found that the mean was the strongest predictor of the outcomes considered, with little additional variance explained by other summary statistics.
Using Market-Research Panels for Behavioral Science: An Overview and Tutorial
Aaron J. Moss et al.
Moss and colleagues highlight the unique capabilities of online market-research panels and demonstrate how researchers can effectively sample from them. Unlike microtask platforms (e.g., MTurk), market-research panels have access to more than 100 million potential participants worldwide, provide more representative samples, and excel at demographic targeting. However, efficiently gathering data from online panels requires researchers to integrate their surveys with the panels in ways that are rarely used on microtask sites. For instance, they can target demographics that are not preprofiled but are screened for within the survey (e.g., parents of autistic children). Moss and colleagues show how to sample hard-to-reach groups using market-research panels and describe best practices for conducting research using them, including setting in-survey quotas to control sample composition and managing data quality.
Bayesian Repeated-Measures Analysis of Variance: An Updated Methodology Implemented in JASP
Don van den Bergh, Eric-Jan Wagenmakers, and Frederik Aust
Although the Bayesian analysis of variance (ANOVA) is modeled after its frequentist counterpart that uses p values, the two can yield very different conclusions when the design involves multiple repeated-measures factors. Van den Bergh and colleagues illustrate such a discrepancy with a real data set and show that the two types of ANOVA use different model specifications. Contrary to the frequentist ANOVA, the Bayesian ANOVA assumes that there are no individual differences in the magnitude of effects. The authors believe that this assumption is untenable in most applications and therefore neither obvious to nor desired by most researchers. Thus, they argue that the Bayesian ANOVA should be revised to allow for individual differences and provide guidance on how to implement the revised model.
Dynamic Data Visualizations to Enhance Insight and Communication Across the Life Cycle of a Scientific Project
Kristina Wiebels and David Moreau
In scientific communication, figures are typically rendered as static displays, which often prevents active exploration of the underlying data. Wiebels and Moreau present recent developments to build interactivity and animations into scientific communications using examples and illustrations in the R language. They discuss when and how to build dynamic figures, with step-by-step reproducible code that can be adapted to the reader’s own projects. The authors illustrate how interactivity and animations can facilitate insight and communication across a project life cycle—from initial exchanges and discussions in a team to peer review and final publication—and provide recommendations to use dynamic visualizations effectively.
Best Practices in Supervised Machine Learning: A Tutorial for Psychologists
Florian Pargent, Ramona Schoedel, and Clemens Stachl
Supervised machine learning (ML) is becoming an influential analytical method. This tutorial intends to provide a primer and introduction to supervised ML for psychologists. Pargent and colleagues introduce the basic terminology and mindset of supervised ML. They a) cover how to use resampling methods to evaluate the performance of ML models and introduce the nonlinear random forest, a type of ML model; b) explain how to compare the performance of several ML models on multiple data sets and discuss the interpretation of ML models; and c) offer code examples using the mlr3 and companion packages in R.
Multidimensional Signals and Analytic Flexibility: Estimating Degrees of Freedom in Human-Speech Analyses
Stefano Coretta, Joseph Casillas, Timo Roettger, and Cheman Baira Agitok
Recent empirical studies have highlighted the large degree of analytic flexibility in data analysis which can lead to substantially different conclusions based on the same data set. Thus, researchers have expressed their concerns that these researcher degrees of freedom might facilitate bias and can lead to claims that do not stand the test of time. Even greater flexibility is to be expected in fields in which the primary data lend themselves to a variety of possible operationalizations. The multidimensional, temporally extended nature of speech constitutes an ideal testing ground for assessing the variability in analytic approaches, which derives not only from aspects of statistical modeling, but also from decisions regarding the quantification of the measured behavior. In the present study, the researchers gave the same speech production data set to 46 teams of researchers and asked them to answer the same research question, resulting in substantial variability in reported effect sizes and their interpretation.
Does Your Smartphone “Know” Your Social Life? A Methodological Comparison of Day Reconstruction, Experience Sampling, and Mobile Sensing
Yannick Roos, Michael Krämer, David Richter, Ramona Schoedel, and Cornelia Wrzus
To study how well mobile sensing—observation of human social behavior using people’s mobile phones—can assess the quantity and quality of social interactions, Roos and colleagues examined how experience-sampling questionnaires, day reconstruction via daily diaries, and mobile sensing agreed in their assessments of face-to-face interactions, calls, and text messages. Results indicated some agreement between measurements of face-to-face interactions and high agreement between measurements of smartphone-mediated interactions. Still, a large number of social interactions were captured by only one method, and the quality of social interactions was difficult to capture with mobile sensing.
The Appropriateness of Outlier Exclusion Approaches Depends on the Expected Contamination: Commentary on André (2022)
Daniel Villanova
In a recent article, André (2022) addresses the decision to exclude outliers using a threshold across conditions or within conditions and offers a clear recommendation to avoid within-condition exclusions because of the possibility for large false-positive inflation. This commentary notes that André’s simulations did not include the situation for which within-condition exclusion has previously been recommended – when across-condition exclusion would exacerbate selection bias. Examining test performance in this situation confirms the recommendation for within-condition exclusion in such a circumstance. Critically, the suitability of exclusion criteria must be considered in relationship to assumptions about the data-generating mechanism(s).
Feedback on this article? Email [email protected] or login to comment. Interested in writing for us? Read our contributor guidelines.
APS regularly opens certain online articles for discussion on our website. Effective February 2021, you must be a logged-in APS member to post comments. By posting a comment, you agree to our Community Guidelines and the display of your profile information, including your name and affiliation. Any opinions, findings, conclusions, or recommendations present in article comments are those of the writers and do not necessarily reflect the views of APS or the article’s author. For more information, please see our Community Guidelines.
Please login with your APS account to comment.