Practice
Methods: Measuring Change With Power in Intensive Longitudinal Research
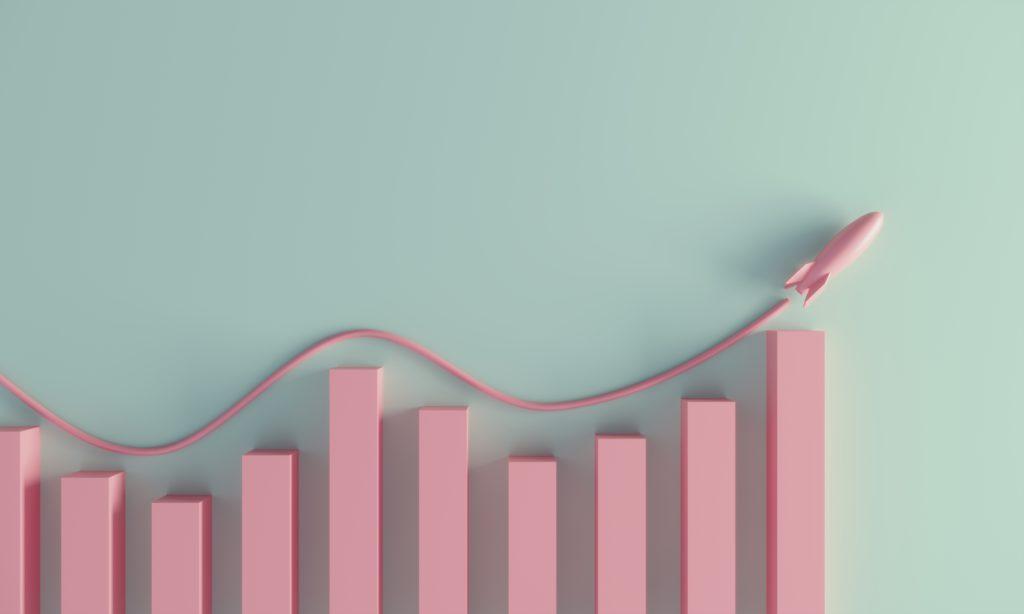
Intensive longitudinal designs allow researchers to characterize changes to complex psychological processes within individuals or groups, along with the causes and consequences of such changes. This type of research involves frequent and repeated measurements of individuals, sometimes in the course of their everyday lives, outside of lab environments, using smartphones and other mobile devices.
Using those repeated measurements, researchers can gain insight into the dynamic psychological processes within individuals, as well as individual differences in the dynamics of these processes. These differences have, for instance, been linked to individual differences in health and well-being. As an example, in a 2010 Psychological Science article, Peter Kuppens and colleagues used intensive longitudinal designs to show that emotional inertia—the degree to which an individual’s emotional states are resistant to change—can be linked to low self-esteem and depression.
Power and Sample Size
Statistical power is the probability of correctly rejecting a null hypothesis when the alternative hypothesis is true in the population under study (Cohen, 1988). Thus, the power to detect an effect depends on (a) the size of the effect on the population, (b) the predetermined Type I error rate (i.e., the significance level), and (c) the standard error of the statistical test used. Power is higher if the effect in the population is larger, the significance level is larger, and the standard error of the statistical test is smaller. Because standard error is related to sample size (larger sample sizes lead to smaller standard errors), power analysis can inform adequate sample sizes. Be sure you select a sample size large enough to detect an effect with a given size in the population. Studies with high power can improve reproducibility of research findings.
The importance of an adequate sample size
However, because intensive longitudinal designs use frequent repeated measures, they require adequate sample-size planning that the usual power calculations employed in other designs may not achieve. An adequate number of participants enables researchers to control the accuracy and power of statistical testing and modeling, contributing to the replicability of empirical findings (e.g., Szucs & Ioannidis, 2017).
“Although power analyses are often used to inform sample-size planning in general (Cohen, 1988), they are not yet well established in IL [intensive longitudinal] research,” write Ginette Lafit, Janne K. Adolf, Egon Dejonckheere, Inez Myin-Germeys, Wolfgang Viechtbauer (Maastricht University), and Eva Ceulemans (all at Katholieke Universiteit Leuven) in a 2021 article in Advances in Methods and Practices in Psychological Science.
In their article, Lafit and colleagues provide a tutorial showing how to perform simulation-based power analyses and select the appropriate number of participants for models widely used in intensive longitudinal research. They also provide the R code for a Shiny application—an interactive web app built straight from R. The code is available via a Git repository hosted on GitHub at github.com/ginettelafit/PowerAnalysisIL and via OSF at osf.io/vguey.
What makes power analyses in intensive longitudinal designs complex?
Lafit and colleagues (2021) explain why performing power analyses for intensive longitudinal designs and selecting an adequate sample size can be challenging, given the intricacy of data obtained with these designs and the potential complexity of the applied statistical models. The reasons include:
- Intensive longitudinal data have a multilevel structure, in that repeated observations are nested within individuals.
- Observations are closer in time in intensive longitudinal research than in traditional longitudinal designs (i.e., measures usually take place several times per day).
- The statistical models used (usually multilevel regression models) have to distinguish interindividual differences from intraindividual changes.
- The statistical models should take temporal dependencies into account to control for them or to quantify and model them, which requires researchers to include either serially correlated errors or the lagged outcome variable as a predictor in the multilevel models.
According to Lafit and colleagues (2021), the main problem researchers might encounter when trying to calculate power (and sample size needed) for intensive longitudinal designs is that the tools available for calculating power in multilevel models do not account for temporal dependencies. The user-friendly application they developed allows researchers to properly account for such temporal dependencies. In their tutorial, they explain how to deploy the app in models that are widely used to study individual differences in intensive longitudinal studies.
Using a Shiny app to perform power analysis
If you are interested in using the power-analysis tool developed by Lafit and colleagues (2021) to calculate the recommended number of participants in intensive longitudinal designs, you can download the app and run it locally on your computer in R or RStudio. On the opening page of the app, select the population model of interest, set the parameter values, and run your power analysis.
“Because many studies use the same sampling protocol (i.e., a fixed number of at least approximately equidistant observations) within individuals, we assume that this protocol is fixed and focus on the number of participants,” Lafit and colleagues (2021) note. In their article, they provide step-by-step instructions and illustrations of computations for different types of models that explicitly account for the temporal dependencies in data by assuming serially correlated errors or including autoregressive effects. These models include:
- models estimating differences between two groups of individuals in the mean of the outcome variable,
- models assessing the effect of a continuous Level 1 predictor on the outcome of interest,
- models assessing the effect of a continuous Level 2 predictor on the outcome of interest,
- models investigating differences between two groups of individuals regarding the association between a Level 1 predictor and the outcome of interest,
- models that account for cross-level interaction between a continuous Level 2 predictor and a continuous Level 1 predictor, and
- multilevel autoregressive models that capture the amount of temporal dependence in the outcome.
This article was initially published in the print edition of the March/April 2022 Observer under the title, “Methods: Measuring Change With Power.”
Feedback on this article? Email [email protected] or scroll down to comment.
References
Cohen, J. (1988). Statistical power analysis for the behavioral sciences (2nd ed.). Erlbaum.
Kuppens, P., Allen, N. B., & Sheeber, L. B. (2010). Emotional inertia and psychological maladjustment. Psychological Science, 21(7), 984–991. https://doi.org/10.1177/0956797610372634
Lafit, G., Adolf, J., Dejonckheere, E., Viechtbauer, W., & Ceulemans, E. (2021). Selection of the number of participants in intensive longitudinal studies: A user-friendly Shiny app and tutorial to perform power analysis in multilevel regression models that account for temporal dependencies. Advances in Methods and Practices in Psychological Science, 4(1). https://doi.org/10.1177/2515245920978738
Szucs, D., & Ioannidis, J. P. A. (2017). Empirical assessment of published effect sizes and power in the recent cognitive neuroscience and psychology literature. PLOS Biology, 15(3), Article e2000797. https://doi.org/10.1371/journal.pbio.2000797
APS regularly opens certain online articles for discussion on our website. Effective February 2021, you must be a logged-in APS member to post comments. By posting a comment, you agree to our Community Guidelines and the display of your profile information, including your name and affiliation. Any opinions, findings, conclusions, or recommendations present in article comments are those of the writers and do not necessarily reflect the views of APS or the article’s author. For more information, please see our Community Guidelines.
Please login with your APS account to comment.