New Content From Advances in Methods and Practices in Psychological Science
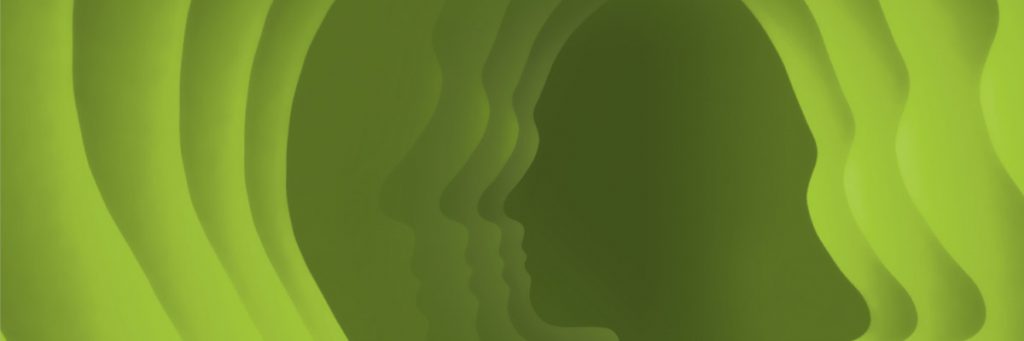
Keeping Meta-Analyses Alive and Well: A Tutorial on Implementing and Using Community-Augmented Meta-Analyses in PsychOpen CAMA
Lisa Bucher, Tanja Burgard, Ulrich Tran, Gerhard Prinz, Michael Bosnjak, and Martin Voracek
Newly developed, web-based, open-repository concepts, such as community-augmented meta-analysis (CAMA), provide open access to fulfill the needs for transparency and timeliness of synthesized evidence. The main idea of CAMA is to keep meta-analyses up-to-date by allowing the research community to include new evidence continuously. In 2021, the Leibniz Institute for Psychology released a platform, PsychOpen CAMA, which serves as a publication format for CAMAs in all fields of psychology. The present work serves as a tutorial on implementing and using a CAMA in PsychOpen CAMA from a data-provider perspective, using six large-scale meta-analytic data sets on the dark triad of personality as a working example. First, the processes of data contribution and implementation of either new or updated existing data sets are summarized. Furthermore, a step-by-step tutorial on using and interpreting CAMAs guides the reader through the web application. Finally, the tutorial outlines the major benefits and the remaining challenges of CAMAs in PsychOpen CAMA.
A Practical Guide to Conversation Research: How to Study What People Say to Each Other
Michael Yeomans, F. Katelynn Boland, Hanne Collins, Nicole Abi-Esber, and Alison Wood Brooks
Conversation—a verbal interaction between two or more people—is a complex, pervasive, and consequential human behavior. Conversations have been studied across many academic disciplines. However, advances in recording and analysis techniques over the last decade have allowed researchers to more directly and precisely examine conversations in natural contexts and at a larger scale than ever before, and these advances open new paths to understand humanity and the social world. Existing reviews of text analysis and conversation research have focused on text generated by a single author (e.g., product reviews, news articles, and public speeches) and thus leave open questions about the unique challenges presented by interactive conversation data (i.e., dialogue). In this article, we suggest approaches to overcome common challenges in the workflow of conversation science, including recording and transcribing conversations, structuring data (to merge turn-level and speaker-level data sets), extracting and aggregating linguistic features, estimating effects, and sharing data. This practical guide is meant to shed light on current best practices and empower more researchers to study conversations more directly—to expand the community of conversation scholars and contribute to a greater cumulative scientific understanding of the social world.
Open-Science Guidance for Qualitative Research: An Empirically Validated Approach for De-Identifying Sensitive Narrative Data
Rebecca Campbell, McKenzie Javorka, Jasmine Engleton, Kathryn Fishwick, Katie Gregory, and Rachael Goodman-Williams
The open-science movement seeks to make research more transparent and accessible. To that end, researchers are increasingly expected to share de-identified data with other scholars for review, reanalysis, and reuse. In psychology, open-science practices have been explored primarily within the context of quantitative data, but demands to share qualitative data are becoming more prevalent. Narrative data are far more challenging to de-identify fully, and because qualitative methods are often used in studies with marginalized, minoritized, and/or traumatized populations, data sharing may pose substantial risks for participants if their information can be later reidentified. To date, there has been little guidance in the literature on how to de-identify qualitative data. To address this gap, we developed a methodological framework for remediating sensitive narrative data. This multiphase process is modeled on common qualitative-coding strategies. The first phase includes consultations with diverse stakeholders and sources to understand reidentifiability risks and data-sharing concerns. The second phase outlines an iterative process for recognizing potentially identifiable information and constructing individualized remediation strategies through group review and consensus. The third phase includes multiple strategies for assessing the validity of the de-identification analyses (i.e., whether the remediated transcripts adequately protect participants’ privacy). We applied this framework to a set of 32 qualitative interviews with sexual-assault survivors. We provide case examples of how blurring and redaction techniques can be used to protect names, dates, locations, trauma histories, help-seeking experiences, and other information about dyadic interactions.
Impossible Hypotheses and Effect-Size Limits
Wijnand van Tilburg and Lennert van Tilburg
Psychological science is moving toward further specification of effect sizes when formulating hypotheses, performing power analyses, and considering the relevance of findings. This development has sparked an appreciation for the wider context in which such effect sizes are found because the importance assigned to specific sizes may vary from situation to situation. We add to this development a crucial but in psychology hitherto underappreciated contingency: There are mathematical limits to the magnitudes that population effect sizes can take within the common multivariate context in which psychology is situated, and these limits can be far more restrictive than typically assumed. The implication is that some hypothesized or preregistered effect sizes may be impossible. At the same time, these restrictions offer a way of statistically triangulating the plausible range of unknown effect sizes. We explain the reason for the existence of these limits, illustrate how to identify them, and offer recommendations and tools for improving hypothesized effect sizes by exploiting the broader multivariate context in which they occur.
Evaluating the Pedagogical Effectiveness of Study Preregistration in the Undergraduate Dissertation
Madeleine Pownall, Charlotte Pennington, Emma Norris, Marie Juanchich, David Smailes, Sophie Russell, Debbie Gooch, Thomas Rhys Evans, Sofia Persson, Matthew Mak, Loukia Tzavella, Rebecca Monk, Thomas Gough, Christopher Benwell, Mahmoud Elsherif, Emily Farran, Thomas Gallagher-Mitchell, Luke Kendrick, Julia Bahnmueller, Emily Nordmann, Mirela Zaneva, Katie Gilligan-Lee, Marina Bazhydai, Andrew Jones, Jemma Sedgmond, Iris Holzleitner, James Reynolds, Jo Moss, Daniel Farrelly, Adam Parker, and Kait Clark
Research shows that questionable research practices (QRPs) are present in undergraduate final-year dissertation projects. One entry-level Open Science practice proposed to mitigate QRPs is “study preregistration,” through which researchers outline their research questions, design, method, and analysis plans before data collection and/or analysis. In this study, we aimed to empirically test the effectiveness of preregistration as a pedagogic tool in undergraduate dissertations using a quasi-experimental design. A total of 89 UK psychology students were recruited, including students who preregistered their empirical quantitative dissertation (n = 52; experimental group) and students who did not (n = 37; control group). Attitudes toward statistics, acceptance of QRPs, and perceived understanding of Open Science were measured both before and after dissertation completion. Exploratory measures included capability, opportunity, and motivation to engage with preregistration, measured at Time 1 only. This study was conducted as a Registered Report; Stage 1 protocol: https://osf.io/9hjbw (date of in-principle acceptance: September 21, 2021). Study preregistration did not significantly affect attitudes toward statistics or acceptance of QRPs. However, students who preregistered reported greater perceived understanding of Open Science concepts from Time 1 to Time 2 compared with students who did not preregister. Exploratory analyses indicated that students who preregistered reported significantly greater capability, opportunity, and motivation to preregister. Qualitative responses revealed that preregistration was perceived to improve clarity and organization of the dissertation, prevent QRPs, and promote rigor. Disadvantages and barriers included time, perceived rigidity, and need for training. These results contribute to discussions surrounding embedding Open Science principles into research training.
It’s All About Timing: Exploring Different Temporal Resolutions for Analyzing Digital-Phenotyping Data
Anna Langener, Gert Stulp, Nicholas Jacobson, Andrea Costanzo, Raj Jagesar, Martien Kas, and Laura Bringmann
The use of smartphones and wearable sensors to passively collect data on behavior has great potential for better understanding psychological well-being and mental disorders with minimal burden. However, there are important methodological challenges that may hinder the widespread adoption of these passive measures. A crucial one is the issue of timescale: The chosen temporal resolution for summarizing and analyzing the data may affect how results are interpreted. Despite its importance, the choice of temporal resolution is rarely justified. In this study, we aim to improve current standards for analyzing digital-phenotyping data by addressing the time-related decisions faced by researchers. For illustrative purposes, we use data from 10 students whose behavior (e.g., GPS, app usage) was recorded for 28 days through the Behapp application on their mobile phones. In parallel, the participants actively answered questionnaires on their phones about their mood several times a day. We provide a walk-through on how to study different timescales by doing individualized correlation analyses and random-forest prediction models. By doing so, we demonstrate how choosing different resolutions can lead to different conclusions. Therefore, we propose conducting a multiverse analysis to investigate the consequences of choosing different temporal resolutions. This will improve current standards for analyzing digital-phenotyping data and may help combat the replications crisis caused in part by researchers making implicit decisions.
A Delphi Study to Strengthen Research-Methods Training in Undergraduate Psychology Programs
Robert Thibault, Deborah Bailey-Rodriguez, James Bartlett, Paul Blazey, Robin Green, Madeleine Pownall, and Marcus Munafò
Psychology programs often emphasize inferential statistical tests over a solid understanding of data and research design. This imbalance may leave graduates underequipped to effectively interpret research and employ data to answer questions. We conducted a two-round modified Delphi to identify the research-methods skills that the UK psychology community deems essential for undergraduates to learn. Participants included 103 research-methods instructors, academics, students, and nonacademic psychologists. Of 78 items included in the consensus process, 34 reached consensus. We coupled these results with a qualitative analysis of 707 open-ended text responses to develop nine recommendations for organizations that accredit undergraduate psychology programs—such as the British Psychological Society. We recommend that accreditation standards emphasize (1) data skills, (2) research design, (3) descriptive statistics, (4) critical analysis, (5) qualitative methods, and (6) both parameter estimation and significance testing; as well as (7) give precedence to foundational skills, (8) promote transferable skills, and (9) create space in curricula to enable these recommendations. Our data and findings can inform modernized accreditation standards to include clearly defined, assessable, and widely encouraged skills that foster a competent graduate body for the contemporary world.
How to Safely Reassess Variability and Adapt Sample Size? A Primer for the Independent Samples t Test
Lara Vankelecom, Tom Loeys, and Beatrijs Moerkerke
When researchers aim to test hypotheses, setting up adequately powered studies is crucial to avoid missing important effects and to increase the probability that published significant effects reflect true effects. Without a priori good knowledge about the population effect size and variability, power analyses may underestimate the true required sample size. However, a specific type of a two-stage adaptive design in which the sample size can be reestimated during the data collection might partially mitigate the problem. In the design proposed in this article, the variability of the data collected at the first stage is estimated and then used to reassess the originally planned sample size of the study while the unstandardized effect size is fixed at a smallest effect size of interest. In this article, we explain how to implement such a two-stage sample-size reestimation design in the setting in which interest lies in comparing means of two independent groups. We investigate through simulation the implications on the Type I error rate (T1ER) of the final independent samples t test. Inflation can be substantial when the interim variance estimate is based on a small sample. However, the T1ER approaches the nominal level when more first-stage data are collected. An R-function is provided that enables researchers to calculate for their specific study (a) the maximum T1ER inflation and (b) the adjusted α level to be used in the final t test to correct for the inflation. Finally, the desired property of this design to better ensure the power of the study is verified.
Best Laid Plans: A Guide to Reporting Preregistration Deviations
Emily Willroth and Olivia Atherton
Psychological scientists are increasingly using preregistration as a tool to increase the credibility of research findings. Many of the benefits of preregistration rest on the assumption that preregistered plans are followed perfectly. However, research suggests that this is the exception rather than the norm, and there are many reasons why researchers may deviate from their preregistered plans. Preregistration can still be a valuable tool, even in the presence of deviations, as long as those deviations are well documented and transparently reported. Unfortunately, most preregistration deviations in psychology go unreported or are reported in unsystematic ways. In the current article, we offer a solution to this problem by providing a framework for transparent and standardized reporting of preregistration deviations, which was developed by drawing on our own experiences with preregistration, existing unpublished templates, feedback from colleagues and reviewers, and the results of a survey of 34 psychology-journal editors. This framework provides a clear template for what to do when things do not go as planned. We conclude by encouraging researchers to adopt this framework in their own preregistered research and by suggesting that journals implement structural policies around the transparent reporting of preregistration deviations.
A Multilab Replication of the Induced-Compliance Paradigm of Cognitive Dissonance
David Vaidis et al.
According to cognitive-dissonance theory, performing counterattitudinal behavior produces a state of dissonance that people are motivated to resolve, usually by changing their attitude to be in line with their behavior. One of the most popular experimental paradigms used to produce such attitude change is the induced-compliance paradigm. Despite its popularity, the replication crisis in social psychology and other fields, as well as methodological limitations associated with the paradigm, raise concerns about the robustness of classic studies in this literature. We therefore conducted a multilab constructive replication of the induced-compliance paradigm based on Croyle and Cooper (Experiment 1). In a total of 39 labs from 19 countries and 14 languages, participants (N = 4,898) were assigned to one of three conditions: writing a counterattitudinal essay under high choice, writing a counterattitudinal essay under low choice, or writing a neutral essay under high choice. The primary analyses failed to support the core hypothesis: No significant difference in attitude was observed after writing a counterattitudinal essay under high choice compared with low choice. However, we did observe a significant difference in attitude after writing a counterattitudinal essay compared with writing a neutral essay. Secondary analyses revealed the pattern of results to be robust to data exclusions, lab variability, and attitude assessment. Additional exploratory analyses were conducted to test predictions from cognitive-dissonance theory. Overall, the results call into question whether the induced-compliance paradigm provides robust evidence for cognitive dissonance.
Reproducibility of Published Meta-Analyses on Clinical-Psychological Interventions
Rubén López-Nicolás, Daniel Lakens, Jose A. López-López, Maria Rubio-Aparicio, Alejandro Sandoval-Lentisco, Carmen López-Ibáñez, Desirée Blázquez-Rincón, and Julio Sánchez-Meca
Meta-analysis is one of the most useful research approaches, the relevance of which relies on its credibility. Reproducibility of scientific results could be considered as the minimal threshold of this credibility. We assessed the reproducibility of a sample of meta-analyses published between 2000 and 2020. From a random sample of 100 articles reporting results of meta-analyses of interventions in clinical psychology, 217 meta-analyses were selected. We first tried to retrieve the original data by recovering a data file, recoding the data from document files, or requesting it from original authors. Second, through a multistage workflow, we tried to reproduce the main results of each meta-analysis. The original data were retrieved for 67% (146/217) of meta-analyses. Although this rate showed an improvement over the years, in only 5% of these cases was it possible to retrieve a data file ready for reuse. Of these 146, 52 showed a discrepancy larger than 5% in the main results in the first stage. For 10 meta-analyses, this discrepancy was solved after fixing a coding error of our data-retrieval process, and for 15 of them, it was considered approximately reproduced in a qualitative assessment. In the remaining meta-analyses (18%, 27/146), different issues were identified in an in-depth review, such as reporting inconsistencies, lack of data, or transcription errors. Nevertheless, the numerical discrepancies were mostly minor and had little or no impact on the conclusions. Overall, one of the biggest threats to the reproducibility of meta-analysis is related to data availability and current data-sharing practices in meta-analysis.
Calculating Repeated-Measures Meta-Analytic Effects for Continuous Outcomes: A Tutorial on Pretest–Posttest-Controlled Designs
David R. Skvarc, Matthew Fuller-Tyszkiewicz
Meta-analysis is a statistical technique that combines the results of multiple studies to arrive at a more robust and reliable estimate of an overall effect or estimate of the true effect. Within the context of experimental study designs, standard meta-analyses generally use between-groups differences at a single time point. This approach fails to adequately account for preexisting differences that are likely to threaten causal inference. Meta-analyses that take into account the repeated-measures nature of these data are uncommon, and so this article serves as an instructive methodology for increasing the precision of meta-analyses by attempting to estimate the repeated-measures effect sizes, with particular focus on contexts with two time points and two groups (a between-groups pretest–posttest design)—a common scenario for clinical trials and experiments. In this article, we summarize the concept of a between-groups pretest–posttest meta-analysis and its applications. We then explain the basic steps involved in conducting this meta-analysis, including the extraction of data and several alternative approaches for the calculation of effect sizes. We also highlight the importance of considering the presence of within-subjects correlations when conducting this form of meta-analysis.
Reliability and Feasibility of Linear Mixed Models in Fully Crossed Experimental Designs
Michele Scandola, Emmanuele Tidoni
The use of linear mixed models (LMMs) is increasing in psychology and neuroscience research In this article, we focus on the implementation of LMMs in fully crossed experimental designs. A key aspect of LMMs is choosing a random-effects structure according to the experimental needs. To date, opposite suggestions are present in the literature, spanning from keeping all random effects (maximal models), which produces several singularity and convergence issues, to removing random effects until the best fit is found, with the risk of inflating Type I error (reduced models). However, defining the random structure to fit a nonsingular and convergent model is not straightforward. Moreover, the lack of a standard approach may lead the researcher to make decisions that potentially inflate Type I errors. After reviewing LMMs, we introduce a step-by-step approach to avoid convergence and singularity issues and control for Type I error inflation during model reduction of fully crossed experimental designs. Specifically, we propose the use of complex random intercepts (CRIs) when maximal models are overparametrized. CRIs are multiple random intercepts that represent the residual variance of categorical fixed effects within a given grouping factor. We validated CRIs and the proposed procedure by extensive simulations and a real-case application. We demonstrate that CRIs can produce reliable results and require less computational resources. Moreover, we outline a few criteria and recommendations on how and when scholars should reduce overparametrized models. Overall, the proposed procedure provides clear solutions to avoid overinflated results using LMMs in psychology and neuroscience.
Understanding Meta-Analysis Through Data Simulation With Applications to Power Analysis
Filippo Gambarota, Gianmarco Altoè
Meta-analysis is a powerful tool to combine evidence from existing literature. Despite several introductory and advanced materials about organizing, conducting, and reporting a meta-analysis, to our knowledge, there are no introductive materials about simulating the most common meta-analysis models. Data simulation is essential for developing and validating new statistical models and procedures. Furthermore, data simulation is a powerful educational tool for understanding a statistical method. In this tutorial, we show how to simulate equal-effects, random-effects, and metaregression models and illustrate how to estimate statistical power. Simulations for multilevel and multivariate models are available in the Supplemental Material available online. All materials associated with this article can be accessed on OSF (https://osf.io/54djn/).
Feedback on this article? Email [email protected] or login to comment.
APS regularly opens certain online articles for discussion on our website. Effective February 2021, you must be a logged-in APS member to post comments. By posting a comment, you agree to our Community Guidelines and the display of your profile information, including your name and affiliation. Any opinions, findings, conclusions, or recommendations present in article comments are those of the writers and do not necessarily reflect the views of APS or the article’s author. For more information, please see our Community Guidelines.
Please login with your APS account to comment.