New Content From Advances in Methods and Practices in Psychological Science
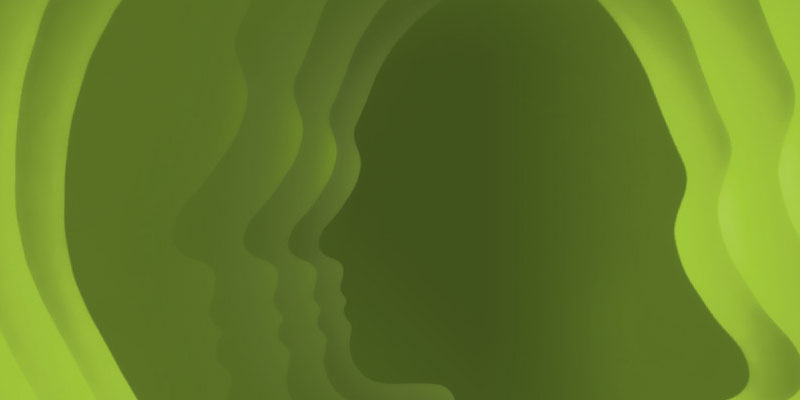
Does Your Smartphone “Know” Your Social Life? A Methodological Comparison of Day Reconstruction, Experience Sampling, and Mobile Sensing
Yannick Roos, Michael Krämer, David Richter, Ramona Schoedel, and Cornelia Wrzus
To study how well mobile sensing—observation of human social behavior using people’s mobile phones—can assess the quantity and quality of social interactions, Roos and colleagues examined how experience-sampling questionnaires, day reconstruction via daily diaries, and mobile sensing agreed in their assessments of face-to-face interactions, calls, and text messages. Results indicated some agreement between measurements of face-to-face interactions and high agreement between measurements of smartphone-mediated interactions. Still, a large number of social interactions were captured by only one method, and the quality of social interactions was difficult to capture with mobile sensing.
Improving Statistical Analysis in Team Science: The Case of a Bayesian Multiverse of Many Labs 4
Suzanne Hoogeveen, Sophie W. Berkhout, Quentin F. Gronau, Eric-Jan Wagenmakers, and Julia M. Haaf
Team science projects have become the gold standard for assessing the replicability and variability of key findings in psychological science. However, we believe the typical meta-analytic approach in these projects fails to match the wealth of collected data. Instead, we advocate the use of Bayesian hierarchical modeling for team science projects, potentially extended in a multiverse analysis. We illustrate this full-scale analysis by applying it to the recently published Many Labs 4 project. This project aimed to replicate the mortality salience effect – that being reminded of one’s own death strengthens one’s cultural identity. In a multiverse analysis we assess the robustness of the results with varying data inclusion criteria and prior settings. Bayesian model comparison results largely converge to a common conclusion: the data provide evidence against a mortality salience effect across the majority of our analyses. We issue general recommendations to facilitate full-scale analyses in team science projects.
A Tutorial on Causal Inference in Longitudinal Data With Time-Varying Confounding Using G-Estimation
Wen Wei Loh and Dongning Ren
Causal inference of longitudinal data (e.g., the effect of treatment on an outcome over time) in the presence of time-varying confounding can be challenging. Loh and Ren introduce g-estimation, a powerful analytic tool designed to handle time-varying confounding variables affected by treatment. They offer step-by-step guidance on implementing the g-estimation method using standard parametric regression functions familiar to psychological researchers and commonly available in statistical software. They provide software code at each step using R. All the R code presented in this tutorial is publicly available online.
Modeling Cluster-Level Constructs Measured by Individual Responses: Configuring a Shared Approach
Suzanne Jak, Terrence Jorgensen, Debby ten Hove, and Barbara Nevicka
When multiple items are used to measure cluster-level constructs with individual-level responses, multilevel confirmatory factor models are useful. How to model constructs across levels is still an active area of research with competing methods being available to capture what can be interpreted as a valid representation of cluster-level phenomena. Moreover, the terminology used for the cluster-level constructs in such models varies across researchers. We therefore provide an overview of used terminology and modeling approaches for cluster-level constructs measured through individual responses. We classify the constructs based on whether (a) the target of measurement is at the cluster level or at the individual level and (b) the construct requires a measurement model or not. Next, we discuss various two-level factor models that have been proposed for multilevel constructs that require a measurement model, and we show that the so-called doubly latent model with cross-level invariance of factor loadings is appropriate for all types of constructs that require a measurement model. We provide two illustrations using empirical data from students and organizational teams on stimulating teaching and on conflict in organizational teams, respectively.
How Many Participants Do I Need to Test an Interaction? Conducting an Appropriate Power Analysis and Achieving Sufficient Power to Detect an Interaction
Nicolas Sommet, David Weissman, Nicolas Cheutin, and Andrew Elliot
Power analysis for first-order interactions poses two challenges: (a) The typical expected effect size of an interaction depends on its shape, and (b) achieving sufficient power is difficult because interactions are often modest in size. Sommet and colleagues address these challenges by (a) explaining the difference between power analyses for interactions and main effects, introducing a taxonomy of 12 types of interactions based on their shapes, and offering sample-size recommendations to detect each interaction; and (b) showing that the median power to detect interactions of a typical size is .18 and testing three approaches to increase power without increasing sample size. The authors also introduce INT×Power (www.intxpower.com), a web application that enables users to draw their interaction and determine the sample size needed to reach the power of their choice.
How Do Science Journalists Evaluate Psychology Research?
Julia Bottesini, Christie Aschwanden, Mijke Rhemtulla, and Simine Vazire
What information do science journalists use when evaluating psychology findings? We examined this in a preregistered, controlled experiment by manipulating four factors in descriptions of fictitious behavioral-psychology studies: (a) the study’s sample size, (b) the representativeness of the study’s sample, (c) the p value associated with the finding, and (d) institutional prestige of the researcher who conducted the study. We investigated the effects of these manipulations on 181 real journalists’ perceptions of each study’s trustworthiness and newsworthiness. Sample size was the only factor that had a robust influence on journalists’ ratings of how trustworthy and newsworthy a finding was; larger sample sizes led to an increase of about two-thirds of 1 point on a 7-point scale. University prestige had no effect in this controlled setting, and the effects of sample representativeness and of p values were inconclusive, but any effects in this setting are likely quite small. Exploratory analyses suggest that other types of prestige might be more important (i.e., journal prestige) and that study design (experimental vs. correlational) may also affect trustworthiness and newsworthiness.
Psychology Is a Property of Persons, Not Averages or Distributions: Confronting the Group-to-Person Generalizability Problem in Experimental Psychology
Ryan McManus, Liane Young, and Joseph Sweetman
When experimental psychologists make a claim (e.g., “Participants judged X as morally worse than Y”), how many participants are represented? Such claims are often based exclusively on group-level analyses; here, psychologists often fail to report or perhaps even investigate how many participants judged X as morally worse than Y. More troubling, group-level analyses do not necessarily generalize to the person level: “the group-to-person generalizability problem.” We first argue for the necessity of designing experiments that allow investigation of whether claims represent most participants. Second, we report findings that in a survey of researchers (and laypeople), most interpret claims based on group-level effects as being intended to represent most participants in a study. Most believe this ought to be the case if a claim is used to support a general, person-level psychological theory.
Third, building on prior approaches, we document claims in the experimental-psychology literature, derived from sets of typical group-level analyses, that describe only a (sometimes tiny) minority of participants. Fourth, we reason through an example from our own research to illustrate this group-to-person generalizability problem. In addition, we demonstrate how claims from sets of simulated group-level effects can emerge without a single participant’s responses matching these patterns. Fifth, we conduct four experiments that rule out several methodology-based noise explanations of the problem. Finally, we propose a set of simple and flexible options to help researchers confront the group-to-person generalizability problem in their own work.
Selective Hypothesis Reporting in Psychology: Comparing Preregistrations and Corresponding Publications
Olmo van den Akker, Marcel van Assen, Manon Enting, Myrthe De Jonge, How Hwee Ong, Franziska Rüffer, Martijn Schoenmakers, Andrea Stoevenbelt, Jelte Wicherts, and Marjan Bakker
In this study, we assessed the extent of selective hypothesis reporting in psychological research by comparing the hypotheses found in a set of 459 preregistrations with the hypotheses found in the corresponding articles. We found that more than half of the preregistered studies we assessed contained omitted hypotheses (N = 224; 52%) or added hypotheses (N = 227; 57%), and about one-fifth of studies contained hypotheses with a direction change (N = 79; 18%). We found only a small number of studies with hypotheses that were demoted from primary to secondary importance (N = 2; 1%) and no studies with hypotheses that were promoted from secondary to primary importance. In all, 60% of studies included at least one hypothesis in one or more of these categories, indicating a substantial bias in presenting and selecting hypotheses by researchers and/or reviewers/editors.
Contrary to our expectations, we did not find sufficient evidence that added hypotheses and changed hypotheses were more likely to be statistically significant than nonselectively reported hypotheses. For the other types of selective hypothesis reporting, we likely did not have sufficient statistical power to test for a relationship with statistical significance. Finally, we found that replication studies were less likely to include selectively reported hypotheses than original studies. In all, selective hypothesis reporting is problematically common in psychological research. We urge researchers, reviewers, and editors to ensure that hypotheses outlined in preregistrations are clearly formulated and accurately presented in the corresponding articles.
Tutorial: Power Analyses for Interaction Effects in Cross-Sectional Regressions
David Baranger, Megan Finsaas, Brandon Goldstein, Colin Vize, Donald Lynam, and Thomas Olino
Interaction analyses (also termed “moderation” analyses or “moderated multiple regression”) are a form of linear regression analysis designed to test whether the association between two variables changes when conditioned on a third variable. It can be challenging to perform a power analysis for interactions with existing software, particularly when variables are correlated and continuous. Moreover, although power is affected by main effects, their correlation, and variable reliability, it can be unclear how to incorporate these effects into a power analysis. The R package InteractionPoweR and associated Shiny apps allow researchers with minimal or no programming experience to perform analytic and simulation-based power analyses for interactions.
At minimum, these analyses require the Pearson’s correlation between variables and sample size, and additional parameters, including reliability and the number of discrete levels that a variable takes (e.g., binary or Likert scale), can optionally be specified. In this tutorial, we demonstrate how to perform power analyses using our package and give examples of how power can be affected by main effects, correlations between main effects, reliability, and variable distributions. We also include a brief discussion of how researchers may select an appropriate interaction effect size when performing a power analysis.
Bayesian Analysis of Cross-Sectional Network Psychometrics: A Tutorial in R and JASP
Karoline Huth, Jill de Ron, Anneke Goudriaan, Judy Luigjes, Reza Mohammadi, Ruth van Holst, Eric-Jan Wagenmakers, and Maarten Marsman
Network psychometrics is a new direction in psychological research that conceptualizes psychological constructs as systems of interacting variables. In network analysis, variables are represented as nodes, and their interactions yield (partial) associations. Current estimation methods mostly use a frequentist approach, which does not allow for proper uncertainty quantification of the model and its parameters. Here, we outline a Bayesian approach to network analysis that offers three main benefits. In particular, applied researchers can use Bayesian methods to (1) determine structure uncertainty, (2) obtain evidence for edge inclusion and exclusion (i.e., distinguish conditional dependence or independence between variables), and (3) quantify parameter precision. In this article, we provide a conceptual introduction to Bayesian inference, describe how researchers can facilitate the three benefits for networks, and review the available R packages. In addition, we present two user-friendly software solutions: a new R package, easybgm, for fitting, extracting, and visualizing the Bayesian analysis of networks and a graphical user interface implementation in JASP. The methodology is illustrated with a worked-out example of a network of personality traits and mental health.
Conducting Research With People in Lower-Socioeconomic-Status Contexts
Lydia Emery, David Silverman, and Rebecca Carey
In recent years, the field of psychology has increasingly recognized the importance of conducting research with lower-socioeconomic-status (SES) participants. Given that SES can powerfully shape people’s thoughts and actions, socioeconomically diverse samples are necessary for rigorous, generalizable research. However, even when researchers aim to collect data with these samples, they often encounter methodological and practical challenges to recruiting and retaining lower-SES participants in their studies. We propose that there are two key factors to consider when trying to recruit and retain lower-SES participants—trust and accessibility. Researchers can build trust by creating personal connections with participants and communities, paying participants fairly, and considering how participants will view their research. Researchers can enhance accessibility by recruiting in participants’ own communities, tailoring study administration to participants’ circumstances, and being flexible in payment methods. Our goal is to provide recommendations that can help to build a more inclusive science.
Feedback on this article? Email [email protected] or login to comment.
APS regularly opens certain online articles for discussion on our website. Effective February 2021, you must be a logged-in APS member to post comments. By posting a comment, you agree to our Community Guidelines and the display of your profile information, including your name and affiliation. Any opinions, findings, conclusions, or recommendations present in article comments are those of the writers and do not necessarily reflect the views of APS or the article’s author. For more information, please see our Community Guidelines.
Please login with your APS account to comment.