New Content from Advances in Methods and Practices in Psychological Science
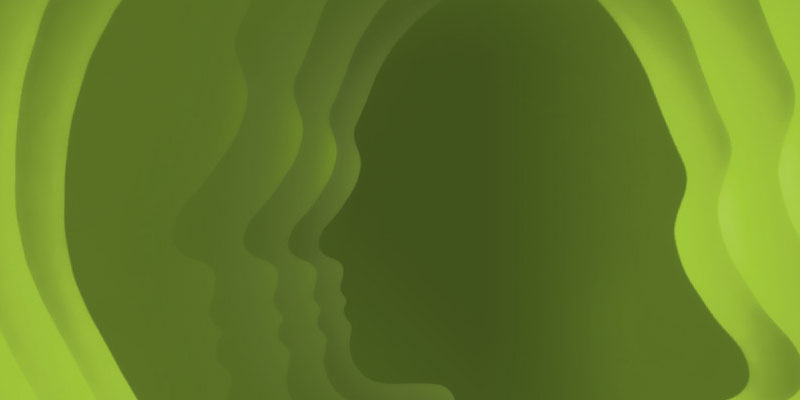
A Causal Framework for Cross-Cultural Generalizability
Dominik Deffner, Julia M. Rohrer, and Richard McElreath
Researchers increasingly recognize the need for more diverse samples that capture the breadth of human experience. Current attempts to establish generalizability across populations focus on threats to validity, constraints on generalization, and the accumulation of large, cross-cultural data sets. However, Deffner and colleagues argue that continued progress requires a framework that helps researchers determine which inferences can be drawn and then make informative cross-cultural comparisons. They describe a generative causal-modeling framework and outline criteria to derive analytic strategies and implied generalizations. They also demonstrate how to apply the framework, using both simulated and real data.
Adjusting for Publication Bias in JASP and R: Selection Models, PET-PEESE, and Robust Bayesian Meta-Analysis
František Bartoš, Maximilian Maier, Daniel S. Quintana, and Eric-Jan Wagenmakers
In this tutorial, Bartoš and colleagues demonstrate how to both conduct a publication-bias-adjusted meta-analysis in JASP and R and interpret the results. They explain two frequentist bias-correction methods: precision-effect test and precision-effect estimate with standard errors (PET-PEESE) and selection models. They then introduce robust Bayesian meta-analysis, a Bayesian approach that simultaneously considers both PET-PEESE and selection models. Bartoš and colleagues illustrate the methodology on an example data set, provide an instructional video (https://bit.ly/ pubbias) and an R-markdown script (https://osf.io/uhaew/), and discuss the interpretation of results. Finally, the researchers include concrete guidance on reporting the meta-analytic results in an academic article.
Hybrid Experimental Designs for Intervention Development: What, Why, and How
Inbal Nahum-Shani, John J. Dziak, Maureen A. Walton, and Walter Dempsey
Effective psychological interventions require the integration of digital and human-delivered components, Nahum-Shani and colleagues argue. Thus, they introduce a new approach—the hybrid experimental design (HED)—that can answer scientific questions about building those interventions and adapting them at multiple timescales. The researchers describe HED’s key characteristics, explain its scientific rationale (i.e., why it is needed), and provide guidelines for its design and corresponding data analysis, focusing on how data derived from HEDs can be used to inform effective and scalable psychological interventions.
That’s a Lot to Process! Pitfalls of Popular Path Models
Julia M. Rohrer, Paul Hünermund, Ruben C. Arslan, and Malte Elson
It might be difficult to understand the causal inference problems that underlie path models, which test moderation and moderation claims and are used to understand effects’ underlying processes and potential boundary conditions. Rohrer and colleagues explain the limited conditions under which standard procedures for mediation and moderation analyses can succeed. They discuss why reversing arrows or comparing model-fit indices do not reveal which model is the right one and how tests of conditional independence can at least determine where a model goes wrong. They suggest the need for a research culture in which causal inference is pursued deliberately and collaboratively.
Statistical Control Requires Causal Justification
Anna C. Wysocki, Katherine M. Lawson, and Mijke Rhemtulla
Controlling for relevant confounders in correlational or quasiexperimental studies can bring the estimated regression coefficient closer to the value of the true causal effect. However, when the selected control variables are inappropriate, controlling can result in estimates that are more biased than uncontrolled estimates. Wysocki and colleagues argue that to carefully select appropriate control variables, researchers must propose and defend a causal structure that includes the outcome, predictors, and plausible confounders. They underscore the importance of causality when selecting control variables by demonstrating how controlling for appropriate and inappropriate variables affects regression coefficients. They also provide practical recommendations for applied researchers who wish to use statistical control.
Justify Your Alpha: A Primer on Two Practical Approaches
Maximilian Maier and Daniël Lakens
Maier and Lakens explain two approaches that can be used to justify a better choice of an alpha level than relying on the default threshold of .05. The first approach relates to minimizing or balancing Type 1 and Type 2 error rates. The second approach lowers the alpha level as a function of the sample size to prevent Lindley’s paradox (i.e., in studies with very high statistical power, p values lower than the alpha level can be more likely when the null hypothesis is true than when the alternative hypothesis is true). The researchers argue that both approaches have limitations but are an improvement to current practices. The authors provide an R package and Shiny app to perform the required calculations.
Analyzing GPS Data for Psychological Research: A Tutorial
Sandrine R. Müller et al.
In this tutorial, Müller and colleagues provide a practical guide to analyzing GPS data in R and introduce researchers to key procedures and resources for conducting spatial analytics. They show readers how to clean GPS data, compute mobility features (e.g., time spent at home, number of unique places visited), and visualize locations and movement patterns. They also discuss the challenges of ensuring participant privacy and interpreting the psychological implications of mobility behaviors. The tutorial is accompanied by an R Markdown script and a simulated GPS data set available at https://osf.io/2d5ep.
Four Internal Inconsistencies in Tversky and Kahneman’s (1992) Cumulative Prospect Theory Article: A Case Study in Ambiguous Theoretical Scope and Ambiguous Parsimony
Michel Regenwetter, Maria M. Robinson, and Cihang Wang
Regenwetter and colleagues advocate for accelerating scientific discovery by investing more effort in overtly specifying and painstakingly delineating the intended purview of any proposed new theory at the time of its inception. They consider Tversky and Kahneman (1992) as a case study, indicating that the reported findings provide evidence that at least half of their participants violated the authors’ proposed cumulative prospect theory. Regenwetter and colleagues highlight a combination of conflicting findings in the original article that make it ambiguous to evaluate both the scope and parsimony of cumulative prospect theory using the authors’ own evidence. They suggest that this is illustrative of a social and behavioral research culture in which the role of theoretical scope is mostly to call existing theory into question and motivate surrogate proposals.
Data Visualization Using R for Researchers Who Do Not Use R
Emily Nordmann, Phil McAleer, Wilhelmiina Toivo, Helena Paterson, and Lisa M. DeBruine
In this tutorial, Nordmann and colleagues detail the rationale for using R for data visualization and introduce the “grammar of graphics” underlying data visualization used by the ggplot package. They then walk the reader through how to replicate plots that are commonly available in point-and-click software, such as histograms and box plots, and show how the code for these “basic” plots can be easily extended to less commonly available options, such as violin box plots. The data set and code used in this tutorial and an interactive version with activity solutions, additional resources, and advanced plotting options are available at https://osf.io/bj83f/.
Effective Maps, Easily Done: Visualizing Geo-Psychological Differences Using Distance Weights
Tobias Ebert, Lars Mewes, Friedrich M. Götz, and Thomas Brenner
In this tutorial, Ebert and colleagues introduce psychologists to an easy-to-use mapping technique: distance-based weighting (i.e., calculating area estimates that represent distance-weighted averages of all measurement locations). This is an alternative to most psychologists’ basic mapping technique of color-coding disaggregated data (i.e., grouping individuals into predefined spatial units and then mapping out average scores across these spatial units). Ebert and colleagues explain how to implement distance-based weighting so that it is effective for geo-psychological research. They use large-scale mental-health data from the United States to illustrate the technique and provide fully annotated R code and open access to all data used in their analyses.
Feedback on this article? Email [email protected] or login to comment. Interested in writing for us? Read our contributor guidelines.
APS regularly opens certain online articles for discussion on our website. Effective February 2021, you must be a logged-in APS member to post comments. By posting a comment, you agree to our Community Guidelines and the display of your profile information, including your name and affiliation. Any opinions, findings, conclusions, or recommendations present in article comments are those of the writers and do not necessarily reflect the views of APS or the article’s author. For more information, please see our Community Guidelines.
Please login with your APS account to comment.